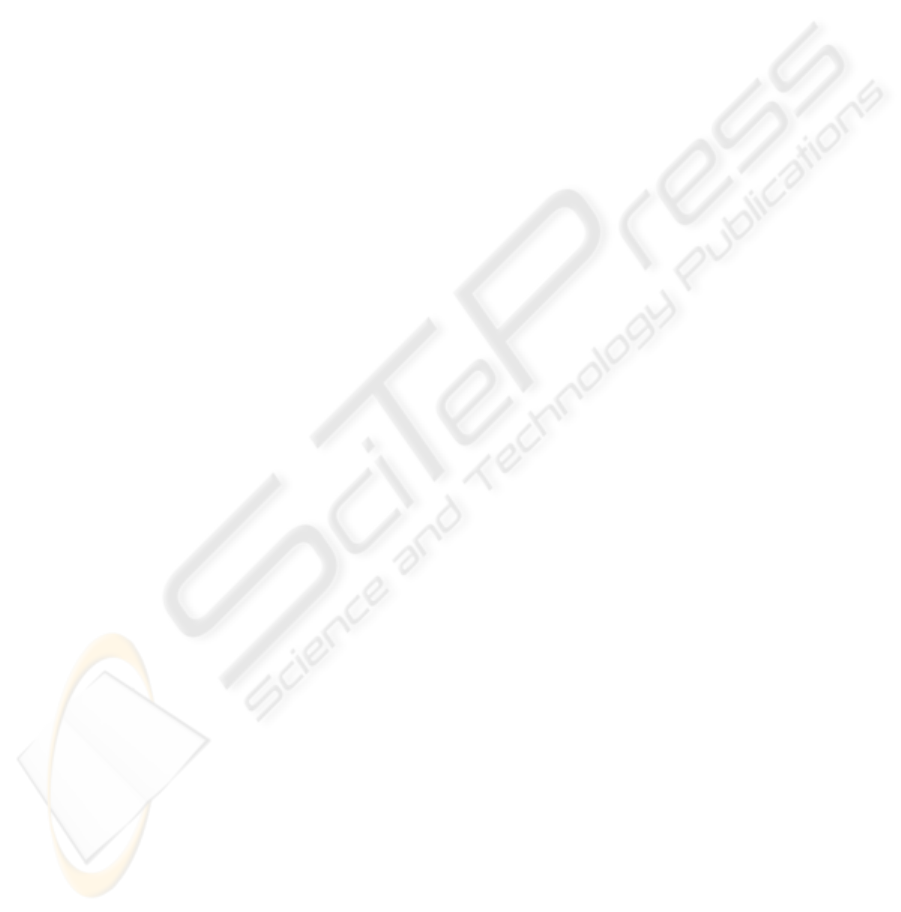
faster, even with the calculation of the Hessian requir-
ing a high computational effort.
5 CONCLUSIONS
In this work a new approach of the Neural GPC was
presented which complies a little modification in the
control law, given by the adding of one more degree
of freedom associated to the decrecent gradient. This
modification caused a significant improvement in the
control effort and in the general system’s closed-loop
response without significant increase of the compu-
tational effort. Furthermore, the algorithm becomes
much more flexible when compared to other one-
degree of freedom based strategies.
The trials were executed in a real-time nonlin-
ear physical system with complex dynamics, with
non-minimum phase states and highly nonlinear static
gains along the diferente operation ranges. The neu-
ral model was able to represent with very good accu-
racy the system dynamics, which shows the efficiency
of the neural networks when applied in the nonlinear
system identification. The system was implemented
to prove in practice the superior performance of the
proposed technique. The same algorithm may be ap-
plied in the control of important industrial process,
as in multivariable control, level, concentration and
temperature. The developed algorithm may estim-
ulate new applications involving the ideas presented
in this work. The low computational cost allows the
practical implementation of the proposed algorithm in
real-time existing embedded systems.
REFERENCES
(1999). Theromeletric coolers and accessories. Melcor
Corporation, first edition.
Almeida, L. A. L. (2003). Modelo de Histerese para
Transic˜ao Semicondutor-Metal em Filmes Finos de
VO2. PhD thesis, Universidade Federal de Campina
Grande.
Almeida, L. A. L. (2004). Modelo dinmico n˜ao-linear para
m´odulo termoel´etrico. In XV Congresso Brasileiro de
Automtica.
Camacho, E. F. and Bordons, C. (2004). Model predictive
control. Springer-Verlag Limited.
Clarke, D. W. (1994). Advanced in model-based predictive
control. Oxford Univesity Press.
Fontana, M. (2001). Caracterizac˜ao e modelagem das pro-
priedades ´opticas de sensores de di´oxido de van´adio.
Master’s thesis, Universidade Federal de Campina
Grande.
Fontes, A. B., Dorea, C. E. T., and Garcia, M. R. S. (2008).
An iterative algorithm forconstrained mpc with stabil-
ity of bilinear systems. In 16th Mediterranean Confer-
ence on Control and Automation, pages 1526–1531.
Garcia, M. R. S. (2008). Controle preditivo por realimen-
tac˜ao de estado de sistemas bilineares sob restric˜oes
aplicado a um m´odulo termoel´etrico. Master’s thesis,
Universidade Federal da Bahia.
Goodhart, S. G., Burnham, K. J., and James, D. J. G.
(1994). Bilinear self-tuning control of a high temper-
ature heat treatment plant. IEE Proc.-Control Theory
Appl., 141(1):12–18.
Hagan, M. T. and Menhaj, M. (1994). Trainning feedfor-
ward networks with the marquadt algorithm. IEEE
Transactions on Neural Networks, 5(6):989–993.
Hao, J., Tan, S., and Vandewalle, J. (1993). One step ahead
predictive control of nonlinear systems by neural net-
works. In Proceedings of 1993 International Joint
Conference on Neural Networks.
Lee, J. H. and Ricker, N. L. (1994). Extended kalman filter
based nonlinear model predictive control. Ind. Eng.
Chem. Res., 33(6):1530–1541.
Li, W. C. and Biegler, L. T. (1988). Process control strate-
gies for constrained nonlinear systems. Ind. Eng.
Chem. Res., (27):1421–1433.
Lima, J. S. (2007). T´ecnicas de controle preditivo bilinear
aplicado a um m´odulo termoel´etrico. Master’s thesis,
Universidade Federal da Bahia.
Propoi, A. I. (1963). Use of lp methods for synthesiz-
ing sampled-data automatic systems. Automn Remote
Control, 24.
Santana, M. J. C. (2008). Controle preditivo multimod-
elo aplicado a um m´odulo termoel´etrico: Uma nova
m´etrica. Master’s thesis, Universidade Federal da
Bahia.
Sobrinho, M. O. S. L., Lima, J. S., Souza, V. O. S. T.,
Fontes, A. B., and Almeida, L. A. L. (2006). Car-
acterizac˜ao de um m´odulo termoel´etrico por modelo
param´etrico bilinear. Congresso Brasileiro de Au-
tomtica.
Soloway, D. and Halcy, P. J. (1996). Neural generalized pre-
dictive control, a newton-raphason implementation. In
Symposium on Intelligent Control.
Zamarreo, J. M. and Vega, P. (1999). Neural predictive con-
trol. application to a highly non-linear system. En-
gineering Applications of Artificial IntelligenceJapan,
(12):149–158.
ICINCO 2009 - 6th International Conference on Informatics in Control, Automation and Robotics
194