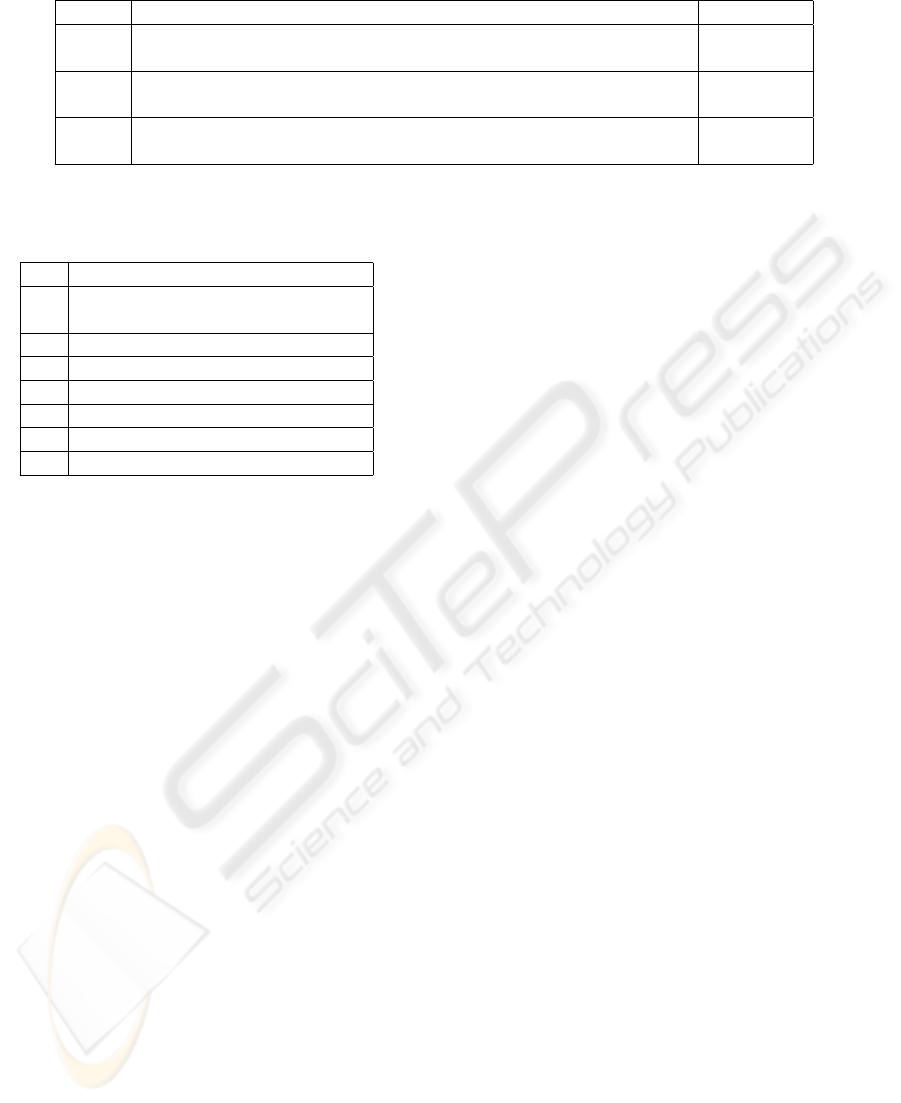
Table 3: Rules found by FAGNIS in experiment 2.
Rule # Rule description Data points
1 IF x = (-2.863 -0.715 1.839 -0.294 0.264 -0.014 0.035) 110
THEN y = (1.442 -0.024 0.001 0.032 -0.033 0.005 -0.004 -0.003)
2 IF x = (2.554 -1.616 -1.161 0.165 0.154 -0.095 -0.022) 68
THEN y = (-0.290 -0.002 -0.378 -0.117 -0.392 0.006 -0.005 -0.235)
3 IF x = (4.356 0.069 2.064 -0.852 -0.152 -0.221 0.596) 42
THEN y = (-2.804 0.874 0.339 -0.386 0.737 -0.027 0.001 0.127)
Table 4: Most important variables used for principal com-
ponents characterization.
PC Description
1 Industrial load, 60 days ago and
Industrial load, 30 days ago
2 Average temperature, 60 days ago
3 Average temperature, 120 days ago
4 Industrial load, 30 days ago
5 Average humidity, 60 days ago
6 Average humidity, 90 days ago
7 Average humidity, 120 days ago
6 CONCLUSIONS
A methodology for the acquisition of rules from neu-
ral networks trained to forecast electric load demand
has been presented here. Results found through sev-
eral experiments (been two of them shown in this
paper) attest the methodology’s efficiency in extract
and present high quality rules for different amounts
of time in the future.
Throughout the execution of many experiments, it
was made clear that there is a need to differentiate the
neural networks of load forecast from those used to
rule extraction: the former needs several training cy-
cles in order to obtain a perfect fit to the load demand
curve; the latter requires only a few training cycles to
obtain the overall knowledge about the load demand,
that is, so that a small number of rules can be used to
refer to a large quantity of data points.
Both the forecast model and the rules acquired can
be used as decision support tools for energy supply
companies. For example, several simulations could
be used for the executives to better understand load
demand behavior in different scenarios, such as future
climatic changes.
REFERENCES
Andrews, R., Diederich, J., and Tickle, A. (1995). Survey
and critique of techniques for extracting rules from
trained neural networks. Elsevier Knowledge-Based
Systems.
Angelov, P. (2002). Evolving Rule-based Models: A Tool
for Design of Flexible Adaptive Systems (Studies in
Fuzziness and Soft Computing). Physica-Verlag, Hei-
delberg, first edition.
Benitez, J., Castro, J., and Requena, I. (1997). Are artificial
neural networks black boxes? Neural Networks, IEEE
Transactions on.
Cechin, A. (1998). The Extraction of Fuzzy Rules from Neu-
ral Networks. Shaker Verlag, Tubingen.
Ghods, L. and Kalantar, M. (2008). Methods for long-term
electric load demand forecasting; a comprehensive in-
vestigation. Industrial Technology, 2008. ICIT 2008.
IEEE International Conference on.
Gross, G. and Galiana, F. (1987). Short-term load forecast-
ing. Proceedings of the IEEE.
Hastie, T., Tibshirani, R., and Friedman, J. (2009). The
Elements of Statistical Learning: Data Mining, In-
ference, and Prediction. Springer Series in Statistics,
New York, second edition.
Jang, J., Sun, T., and Mizutani, E. (1997). Neuro-Fuzzy and
Soft Computing. A Computational Approach to Learn-
ing and Machine Intelligence. Prentice-Hall, New Jer-
sey.
Jolliffe, I. (2002). Principal Component Analysis. Springer
Series in Statistics, New York.
Nauck, D., Klawonn, F., and Kruse, R. (1994). Neuronale
Netze und Fuzzy-Systeme. Vieweg and Sohn.
Srinivasan, D., Chang, C., and Liew, A. (1995). Demand
forecasting using fuzzy neural computation, with spe-
cial emphasis on weekend and public holiday forecast-
ing. Power Systems, IEEE Transactions on.
Srinivasan, D., Tan, S. S., Cheng, C., and Chan, E. K.
(1999). Parallel neural network-fuzzy expert system
strategy for short-term load forecasting: system im-
plementation and performance evaluation. Power Sys-
tems, IEEE Transactions on.
ICINCO 2009 - 6th International Conference on Informatics in Control, Automation and Robotics
200