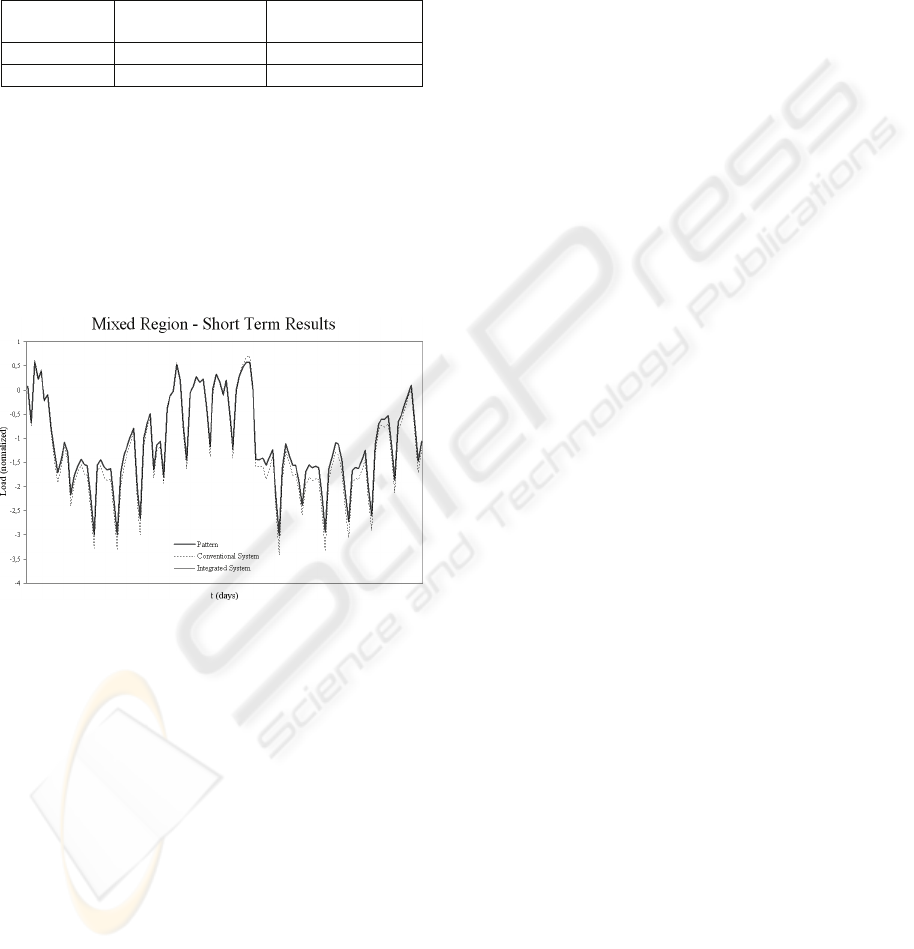
is a balance between residential and industrial
consumers. In those areas there is no definition
about the load behaviour, because it follows the
trend given by the industrial and residential load.
The system outcome to the mixed type of load
consumption is given in Table 3.
Table 3: Industrial region test results.
Forecast
Horizon
Propose
Integrated System
Individually
Models
Long Term 5,5% 22,1%
Short Term 11,7% 24,6%
In Figure 5 is ploted the results for short term
forecast, comparing the pattern wait with outcomes
of conventional forecasting system and the new
neural system proposed in this paper. Note that the
proposed system (represented by solid black line)
fits perfectly with the pattern waited (grey line), the
conventional neural system, represented by the short
term model (dashed line) before developed has a
worst behaviour.
Figure 5: Short term load forecasting for mixed region.
4 CONCLUSIONS
The results show that integration of long and short
term model is beneficial to the response of the
integrated system. This integration improve the
system accuracy for both forecast horizon and also
turns the resulting model generic. That affirmation
can be proved by the close results for the tree types
of load consumption. A generic forecasting system
has a important advantage for commercial usage,
because they could forecast many instances with
only one model.
Finally, the main contribution of this work is a
new neural model for load forecasting, by the
topological level integration usage. With this
integration, the computational system has proved
flexible and capable to generating excellent results.
Some other aspects of the load forecast in electric
systems, like the expansion of the time horizon, will
be published in future works.
REFERENCES
Abdel-Aal, R. E., Short-Term Hourly Load Forecasting
Using Abductive Networks, 2004. IEEE Transactions
on Power Systems, Vol. 19, No. 1.
Ardil, C., Manjunath, T., Mehta H., Mehta, A., 2007. A
Multi-Layer Artificial Neural Network Architecture
Design for Load Forecasting in Power Systems.
International Journal of Applied Mathematics and
Computer Sciences, Vol. 4, No. 4.
Botha E. C., Vermaak, J., 1998. Recurrent Neural
Networks for Short-Term Load Forecasting. IEEE
Transactions on Power Systems, Vol. 13, No. 1.
Charytoniuk, W.; Chen, M. S.; Very Short-Term Load
Forecasting Using Artificial Neural Networks. IEEE
Transactions on Power Systems, Vol. 15, No. 1,
February 2000.
Drezga, I., Rahman, S.; Short-Term Load Forecasting with
Local ANN Predictors. IEEE Transactions on Power
Systems, Vol. 14, No. 3, August 1999.
Fukuyama,Y.; Matsui, T.; Iizaka, T.; A Novel Daily Peak
Load Forecasting Method using Analyzable Structured
Neural Network. IEEE T&D Asia, October 2002.
Funabashi, T.; Uezato, K.; Takara, H.; Senju, T.; One-
Hour-Ahead Load Forecasting Using Neural Network.
IEEE Transactions on Power Systems, Vol. 17, No. 1,
February 2002.
Quintanilha, P., Souza, A., Leme, R., Carpinteiro, O.,
2005. A Hierarchical Hybrid Neural Model with Time
Integrators in Long-Term Peak-Load Forecasting.
International Joint Conference on Neural Networks,
2005. IJCNN '05. Proceedings. 2005 IEEE. Vol. 5,
Pages: 2960- 2965.
Saad, M.; Sood, V.; Kandil, N.; Use of ANNs for Short-
Term Load Forecasting. IEEE Canadian Confrerence
on Electrical and Computer Engineering, May 1999.
Seeger, M., Williams, C., Lawrence, N., 2003. Fast
Forward Selection to Speed Up Sparse Gaussian
Process Regression. Workshop on AI and Statistics.
Shirvany, Y., Hayati, M., 2007. Artificial Neural Network
Approach for Short Term Load Forecasting for Illam
Region. International Journal of Electrical, Computer,
and Systems Engineering, Vol. 1 No. 2.
Swinder, D., Barth, R., Meibom, P., Weber, C., 2007.
Changes of System Operation Costs Due to Large-
Scale Wind Integration. Business and Policy Track:
Integrating wind in electricity markets, 2007.
ICINCO 2009 - 6th International Conference on Informatics in Control, Automation and Robotics
366