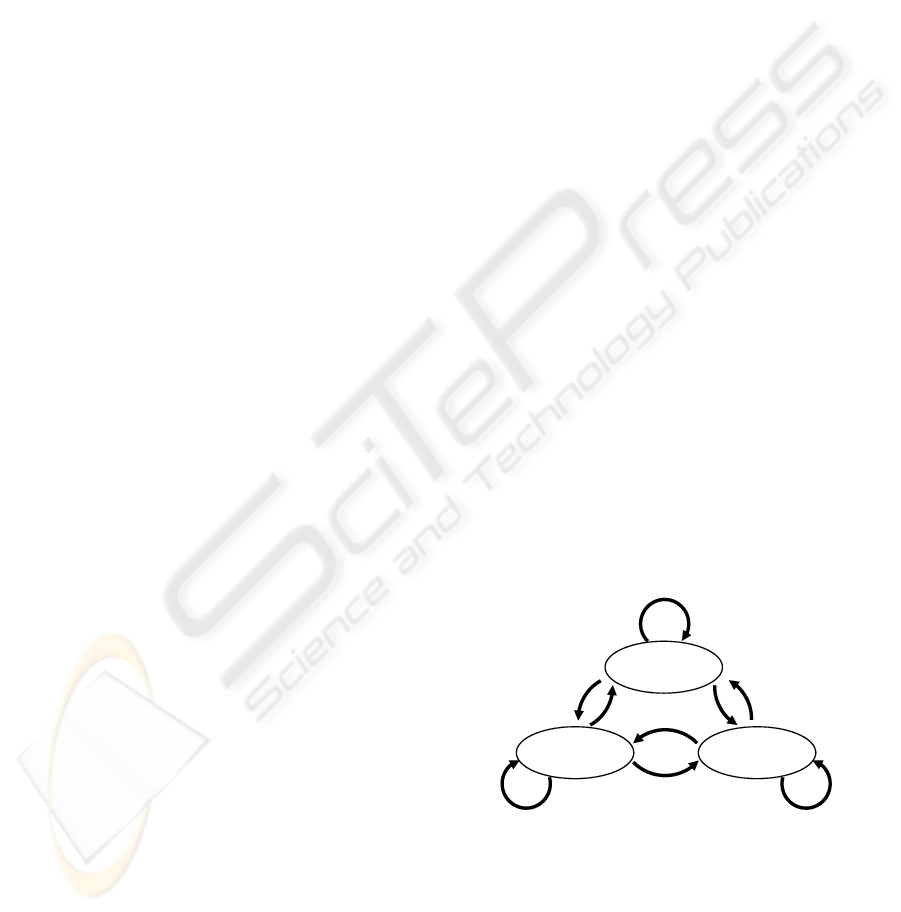
FATIGUE RECOGNITION USING EMG SIGNALS AND
STOCHASTIC SWITCHED ARX MODEL
Hiroyuki Okuda, Fumio Kometani, Shinkichi Inagaki and Tatsuya Suzuki
Nagoya University, Furo-cho, Chikusa-ku, Nagoya, Japan
Keywords:
Human fatigue, Hybrid system, Electro Myo-Gram, Recognition.
Abstract:
The man-machine cooperative system is attracting great attention in many fields, such as industry, welfare
and so on. The assisting system must be designed so as to accommodate the operator’s skill, which might
be strongly affected by the fatigue. This paper presents a new fatigue recognizer based on the Electro Myo-
Gram (EMG) signals and the Stochastic Switched ARX (SS-ARX) model which is one of the extended model
of the standard Hidden Markov Model (HMM). Since the SS-ARX model can represent complex dynamical
relationship which involves switching and stochastic variance, it is expected to show higher performance as
the fatigue recognizer than using simple statistical characteristics of the EMG signal and/or standard HMM.
The usefulness of the proposed strategy is demonstrated by applying to a peg-in-hole task.
1 INTRODUCTION
The man-machine cooperative system is attracting
great attention in many fields, such as manufacturing,
medicine, welfare and so on. The main purpose of
assisting system is to reduce physical burden of the
operator. Since a human skill is strongly affected by
fatigue of the operator, the assisting system must be
designed so as to accommodate with the change of
skill characteristics caused by fatigue. To meet this
requirement, fatigue must be detected and evaluated
based on some quantitative manner. One of the ba-
sic ideas to evaluate the degree of fatigue is to mea-
sure physiological signals, such as the density of lac-
tic acid in blood. This approach, however, requires
the operator to stop the task, to take special examina-
tion and to be injured for sampling.
Recently, Electro Myo-Gram (EMG) signal is rec-
ognized as a promising one to measure the degree
of physical fatigue without any special examination.
EMG signal can be easily detected by only putting
the probe on surface of the corresponding muscle.
The relationship between the fatigue and the change
of features such as Muscle Fiber Conduction Veloc-
ity (MFCV), magnitude, spectrum of EMG and so
on are reported (Sadoyama and Miyano, 1981; Lip-
pold et al., 1960; Arendt-Nielsen and Mills, 1988;
D. K. Kumar and Bradley, 2003). Although these
previous researches enable us to characterize the re-
lationship between fatigue and the statistical charac-
teristics of the EMG signal, their applications have
been restricted in simple monotonous motion because
those measures are developedunder the Maximal Vol-
untary Contraction (MVC) condition. If the target
task is more complex, fatigue recognition based on
these features turns difficult cause of large variance
of the measured signals in dynamic motion. To over-
come this problem, a model-based approach, which
can reflect the effect of the dynamic motion, must be
exploited for the fatigue recognition.
22
a
12
a
21
a
13
a
31
a
33
a
23
a
32
a
11
,t
T
tt
ey
22
+= θφ
,t
T
tt
ey
11
+= θφ
,t
T
tt
ey
33
+= θφ
Figure 1: SS-ARX model (three states).
This paper presents a new fatigue recognizer
based on the EMG signals and the Stochastic
Switched ARX (SS-ARX) model. The SS-ARX
model (Sekizawa et al., 2007) can be regarded as an
extension of standard Hidden Markov Model (HMM)
wherein each Auto Regressive eXogenous (ARX)
model is embedded in each discrete state of the
202
Okuda H., Kometani F., Inagaki S. and Suzuki T. (2009).
FATIGUE RECOGNITION USING EMG SIGNALS AND STOCHASTIC SWITCHED ARX MODEL.
In Proceedings of the 6th International Conference on Informatics in Control, Automation and Robotics - Robotics and Automation, pages 202-207
DOI: 10.5220/0002205502020207
Copyright
c
SciTePress