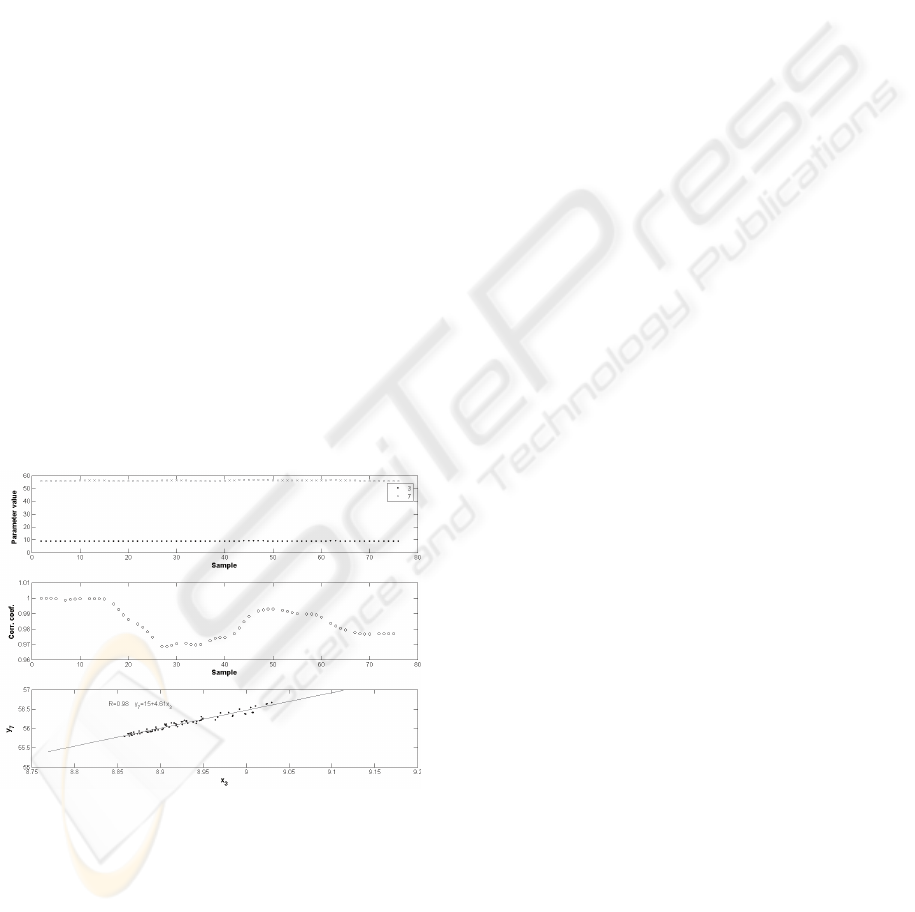
have been also built and expert information used to
enrich the model presented.
3.2 Statistical Analysis for Modeling
Nowadays it is possible to measure, store and retrieve
process sensors’ data and afterwards perform
statistical analysis to “mine” some knowledge. For
this purpose, descriptive and inferential statistics need
to be used.
Taking different combinations of data sets of the
essential process variables, the following results have
been obtained:
(1) Scatter plots of the parameters.
(2) Reduced linear correlation matrix with
entries of defined correlation degree (used for fast
determination of parameter combinations which have
a strong linear correlation).
(3) Linear models of the first order polynomial
(application of stepwise regression, which is a
technique for choosing variables, i.e. terms, to include
in a multiple regression model).
(4) Residuals, confidence intervals of
parameters, t-statistic, p-value, R
2
calculated for the
generated linear models.
(5) Plots of cross-correlation function for
probable lead/lag determination.
Figure 4 presents the fragment correlation and
regression analysis of two process parameters (
3 and
7).
Figure 4: Results of the regression analysis of process
parameters.
These findings are significant for determination
of process parameters and their relationship. They
can also provide additional knowledge for the plant
diagnostics. A more detailed statistical analysis of
granulation process can be found in (Valiulis and
Simutis, 2007).
Mere statistical analysis is rarely helpful. Some
heuristic knowledge should be also applied to make it
work.
3.3 Knowledge-based Modeling
Complex multiscale process systems which are
difficult to model properly (such as granulation)
require a combination of various analytical and
heuristic techniques. Effective solutions are often
based on information from heterogeneous
knowledge sources. One of them is knowledge-
based systems built on the methods and techniques
of Artificial Intelligence.
The expert knowledge of the process is an
invaluable source of knowledge, especially, when
there is a lack of reliable physical description and
suitable measurement equipment. Rule-based expert
systems use “if…then…” rules to represent human
expert knowledge, which is often a mix of
theoretical knowledge, heuristics derived from
experience, and special-purpose rules for dealing
with abnormal situations (Shang, 2004).
An example of the “if…then…” rule of new seed
formation inside the drum granulator-dryer is
presented as follows:
If
granule curtain in the spray zone is poor and
gas temperature in the spray zone is high, then new
small nuclei formation rate is high.
In the proposed modeling approach, the expert
knowledge is represented by the rule set. The rules
involve variables such as “poor”, “high”, dealing
with fuzziness, which is very common in real world
problems. Unlike conventional expert systems,
which are mainly symbolic reasoning engines, fuzzy
expert systems are oriented toward numerical
processing (Hemmer, 2008). These principles can be
applied for the future development of the granulation
process model and simulator for automated guidance
and diagnostic purposes.
4 SIMULATOR
Increasing capabilities of computer hardware and
software ensure the incorporation of complex
knowledge (models) represented by differential and
algebraic equations, measured process data, process
experts’ information, etc. But to be of use for the
day-to-day work of the engineer these models have
to become more user friendly, than the one that the
scientist is dealing with (Ihlow et al., 2004). A new
“GrowSim” simulation package for granulation
process modeling and simulation is under
MODELING OF CONTINUOUS FERTILIZER GRANULATION-DRYING CIRCUIT FOR COMPUTER
SIMULATION AND CONTROL PURPOSES
101