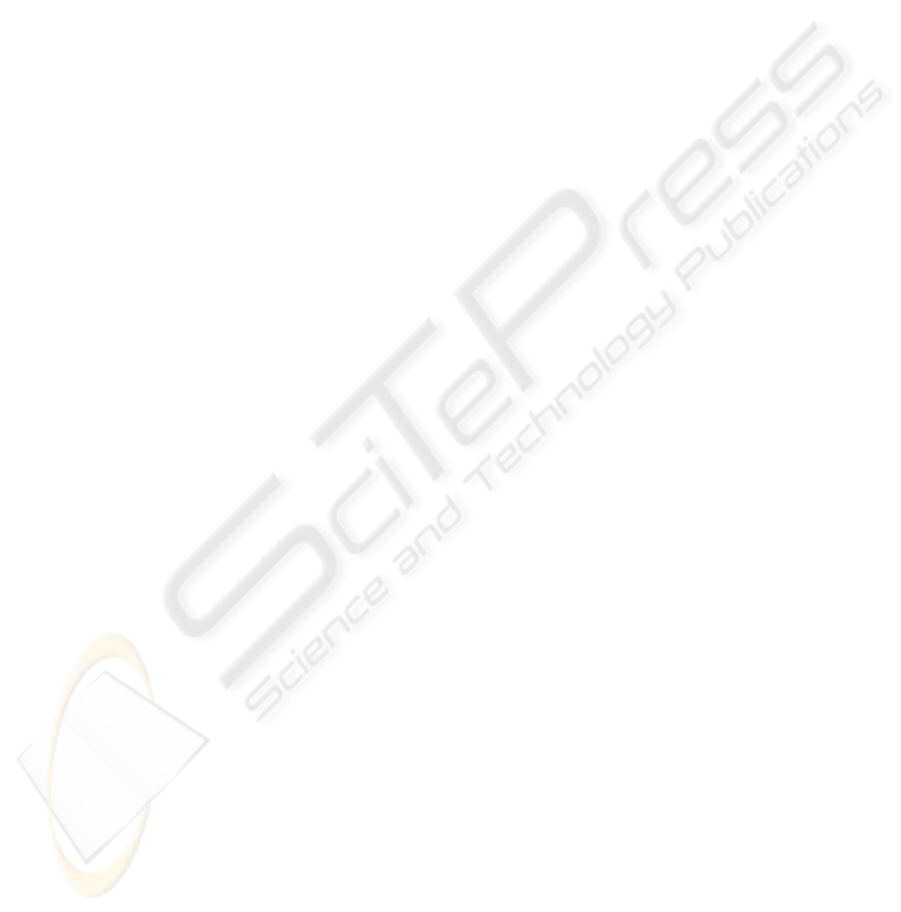
• Flat floor model differences.
Future work will be addressed to solve the above
problems. We believe that the occupancy grid
framework can be used to obtain 3D obstacle
structure. Therefore, there is not limitation
concerning to the number of frames that can be time-
integrated. The future goal will consist in to find a
set of parameters in order to infer 3D obstacle
structure. These set of parameters should be
independent of the source of errors pointed in this
section. The knowledge of 3D structure can afford
several benefits that can be summarised as follows:
• To reduce the trajectories.
• Visual Odometry.
• Landmark detection.
Despite the work that remains undone the
methodology presented can be used to direct the
future research. Moreover, some good features and
results are presented in this work.
ACKNOWLEDGEMENTS
This work has been partially funded by the
Commission of Science and Technology of Spain
(CICYT) through the coordinated project DPI-2007-
66796-C03-02, and by the Government of Catalonia
through the Network Xartap and the consolidated
research group’s grant SGR2005-01008.
REFERENCES
Bruhn A., Weickert J., Schnörr C., 2002. Combining the
advantages of local and global optimal flow methods,
In Proc. Pattern Recognition, Lect. Notes in Comp.
Science, Springer-Verlag, 454-462.
Coue, C., Pradalier, C., Laugier, C., Fraichard, T.,
Bessiere, P., 2006. Bayesian Occupancy Filtering for
Multitarget Tracking: An Automotive Application.
The Inter. Journal of Robotics Research, 25(1) 19-30.
Cumani A., Denasi S., Guiducci A., Quaglia G., 2004.
Integrating Monocular Vision and Odometry for
SLAM. WSEAS Trans. on Computers, 3(3) 625-630.
Elfes, A., 1989. Using occupancy grids for mobile robot
perception and navigation. IEEE Computer, 22(6) 46-
57 .
Gonzalez, R. C., Woods, R. E., 2002. Digital Image
Processing, Prentice Hall Int. Ed., Second Edition.
Hiura, S., Matsuyama, T., 1998. Depth Measurement by
the Multi-Focus Camera, Proc. IEEE CVPR, 953-959.
Horn, B. K. P., 1998. Robot Vision, McGraw-Hill Book
Company, MIT Press Edition, 12
th
printing.
Nayar S.K., Nakagawa, Y., 1994. Shape from Focus, IEEE
Trans. PAMI, 16(8), 824-831.
Nourbakhsh, I., Andre, D., Tomasi, C., Genesereth, M.R.,
1997. Mobile Robot Obstacle Avoidance via Depth
from Focus, Robotics and Autom. Systems, Vol. 22,
151-158.
Pacheco, L., Luo, N., 2007. Trajectory Planning with
Control Horizon Based on Narrow Local Occupancy
Grid Perception. Lect. Notes in Control and Inform.
Sciences 360, Springer-Verlag, pp. 99-106.
Pacheco, L., Cufí, X., Cobos, J., 2007. Constrained
Monocular Obstacle Perception with Just One Frame,
Lect. Notes in Comp. Science, Springer-Verlag, Pattern
Recog. and Image Analysis, Vol. 1, 611-619.
Pacheco, L., Luo, N., Ferrer, I., Cufí,, X., 2008. Control
Education within a Multidisciplinary Summer Course
on Applied Mobile Robotics, Proc. 17th IFAC World
Congress, pp. 11660-11665.
Schäfer H., Proetzsch M., Berns K., 2007. Obstacle
Detection in Mobile Outdoor Robots, Proc. Inter.
Conf. on Informatics in Control, Autom. and Robotics,
pp. 141-148.
Schechner, Y., Kiryati, N., 1998. Depth from Defocus vs.
Stereo: How Different Really Are They?, Proc. IEEE
CVPR, Vol. 2, 256-261.
Subbarao, M., Choi, T., Nikzad, A., 1992. Focusing
Techniques, Tech. Report 92.09.04, Stony Brook,
NewYork.
Surya, G., 1994. Three Dimensional Scene Recovery from
Image Defocus. PHD thesis, Stony Brook, New York.
Thrun S., 2002. Robotic mapping: a survey. Exploring
Artificial Intelligence in the New Millennium, Morgan
Kaufmann, San Mateo.
ICINCO 2009 - 6th International Conference on Informatics in Control, Automation and Robotics
432