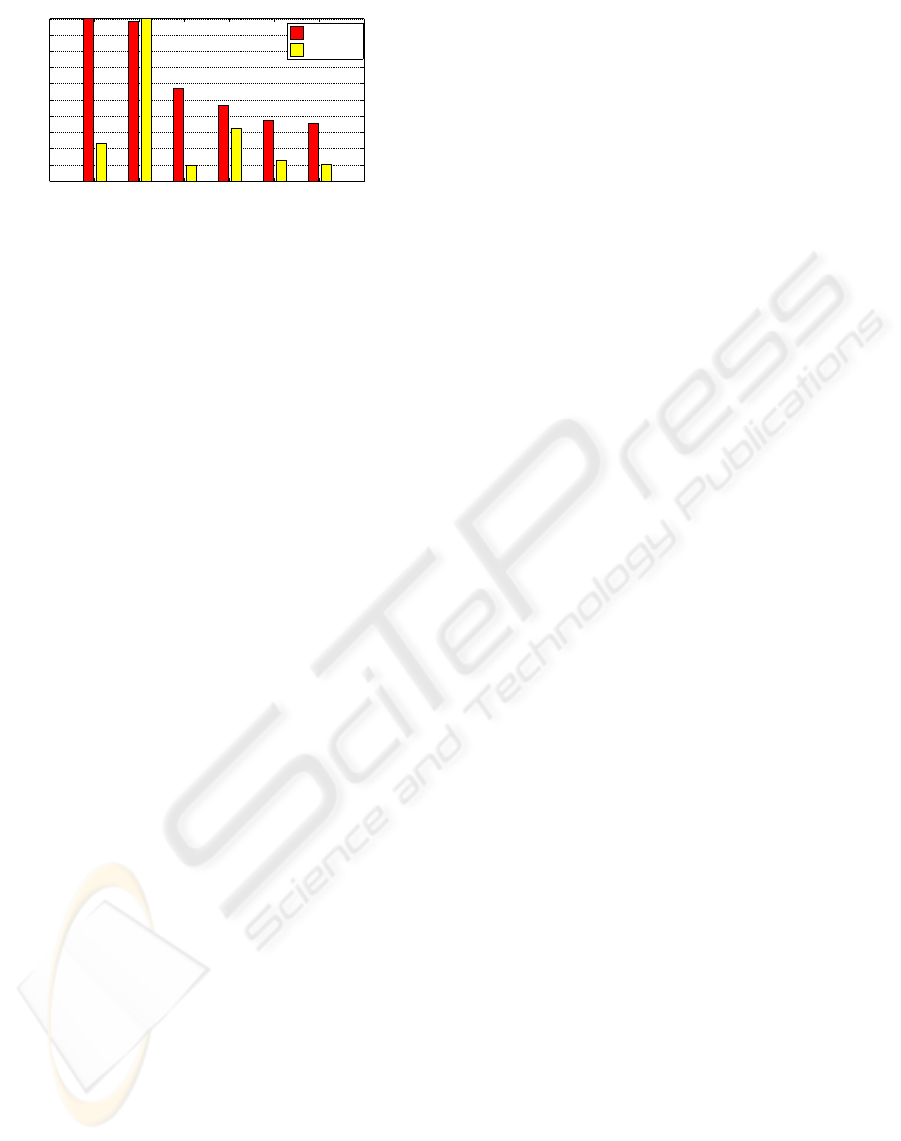
c_max c_min SH ADD Mixed SH−ADD RL
0
0.1
0.2
0.3
0.4
0.5
0.6
0.7
0.8
0.9
1
Comparison of performance indexes obtained by different policies
Normalized performance index value
J
2
=sum(ddz
2
)
J
1
=sum(dz
2
)
Figure 6: Comparison of different policies by using both
cost function J1 and J
2
.
The time domain results are condensed in Figure 6
where the cost functions J
1
and J
2
are reported for dif-
ferent control strategies and compared to the extreme
passive configurations.
Figure 5 shows that BRL policy outperforms the
Mixed SH-ADD at low frequency. This is paid in
terms of filtering at high frequencies where Mixed
SH-ADD shows a better behavior. Figure 5 points
out that BRL policy provides the overall best perfor-
mance in terms of minimization of integral of squared
vertical body accelerations.
7 CONCLUSIONS
In this work we applied Batch Reinforcement Learn-
ing (BRL) to the design problem of optimal comfort-
oriented semi-active suspension which has not been
solved with standard techniques due to its complex-
ity. Results showed that BRL policy provides the best
results in terms of road disturbance filtering. However
the achieved performances are not far from the ones
obtained by the Mixed SH-ADD. Thus, comparing
the numerical approximation given by BRL, against
the analytical approximation given by the Mixed ap-
proach, we showed that they result in a similar strat-
egy. This is an important finding which shows how
numerical-based model-free algorithms can be used
to solve complex control problems. Since BRL tech-
niques can be applied to systems with unknown dy-
namics and are robust to noisy sensors, we expect to
obtain even larger improvements on real motorbikes,
as shown by preliminary experiments.
REFERENCES
Ahmadian, M., Reichert, B. A., and Song, X. (2001).
System non-linearities induced by skyhook dampers.
Shock and Vibration, 8(2):95–104.
Antos, A., Munos, R., and Szepesvari, C. (2008). Fitted
q-iteration in continuous action-space mdps. In Platt,
J., Koller, D., Singer, Y., and Roweis, S., editors, Ad-
vances in Neural Information Processing Systems 20,
pages 9–16. MIT Press, Cambridge, MA.
Ernst, D., Geurts, P., Wehenkel, L., and Littman, L. (2005).
Tree-based batch mode reinforcement learning. Jour-
nal of Machine Learning Research, 6:503–556.
Geurts, P., Ernst, D., and Wehenkel, L. (2006). Extremely
randomized trees. Machine Learning, 63(1):3–42.
Guardabassi, G. and Savaresi, S. (2001). Approximate lin-
earization via feedback - an overview. Survey paper
on Automatica, 27:1–15.
Hrovat, D. (1997). Survey of advanced suspension devel-
opments and related optimal control applications. Au-
tomatica(Oxford), 33(10):1781–1817.
Kaelbling, L. P., Littman, M. L., and Moore, A. W. (1996).
Reinforcement learning: a survey. Journal of Artificial
Intelligence Research, 4:237–285.
Karnopp, D. and Crosby, M. (1974). System for Controlling
the Transmission of Energy Between Spaced Mem-
bers. US Patent 3,807,678.
Riedmiller, M. (2005). Neural fitted q iteration - first experi-
ences with a data efficient neural reinforcement learn-
ing method. In ECML, pages 317–328.
Sammier, D., Sename, O., and Dugard, L. (2003). Sky-
hook and H8 Control of Semi-active Suspensions:
Some Practical Aspects. Vehicle System Dynamics,
39(4):279–308.
Savaresi, S., Silani, E., and Bittanti, S. (2005).
Acceleration-Driven-Damper (ADD): An Optimal
Control Algorithm For Comfort-Oriented Semiactive
Suspensions. Journal of Dynamic Systems, Measure-
ment, and Control, 127:218.
Savaresi, S. and Spelta, C. (2007). Mixed Sky-Hook and
ADD: Approaching the Filtering Limits of a Semi-
Active Suspension. Journal of Dynamic Systems,
Measurement, and Control, 129:382.
Savaresi, S. and Spelta, C. (2008). A single-sensor control
strategy for semi-active suspensions. To Appear, -:–.
Silani, E., Savaresi, S., Bittanti, S., Visconti, A., and
Farachi, F. (2002). The Concept of Performance-
Oriented Yaw-Control Systems: Vehicle Model and
Analysis. SAE Transactions, Journal of Passenger
Cars - Mechanical Systems, 111(6):1808–1818. ISBN
No.0-7680-1290-2,.
Sutton, R. and Barto, A. (1998). Reinforcement Learning:
An Introduction. MIT Press.
Valasek, M., Kortum, W., Sika, Z., Magdolen, L., and
Vaculin, O. (1998). Development of semi-active
road-friendly truck suspensions. Control Engineering
Practice, 6:735–744.
Watkins, C. (1989). Learining from Delayed Rewards. PhD
thesis, Cambridge University, Cambridge,England.
Williams, R. (1997). Automotive active suspensions Part
1: basic principles. Proceedings of the Institution of
Mechanical Engineers, Part D: Journal of Automobile
Engineering, 211(6):415–426.
BATCH REINFORCEMENT LEARNING - An Application to a Controllable Semi-active Suspension System
233