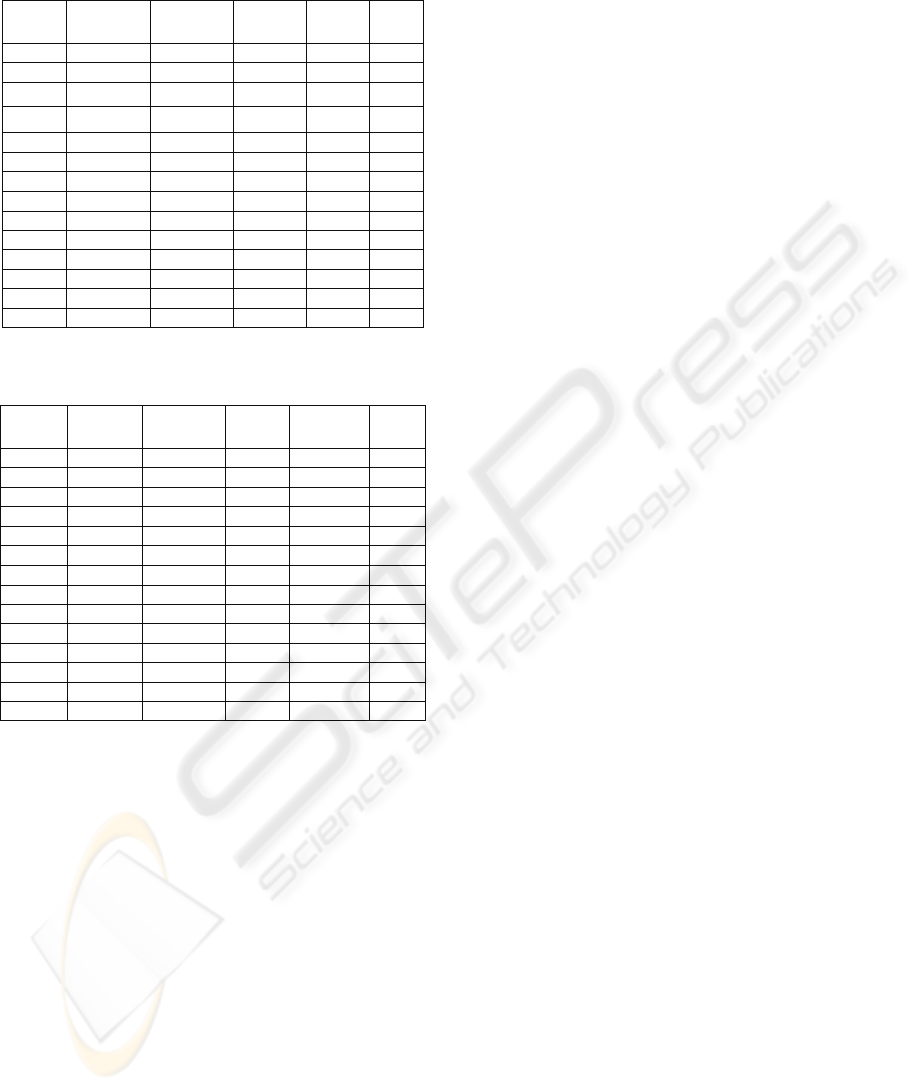
Table 2: Results for the 14 Functions for the CAEP(Nsd)
configuration.
Function MIN FEs AVG FEs
BEST
Fitness
AVG
Fitness
Success
%
F01 12360 12712.8 -450 -450 100
F02 155340 139003.2 -450 -450 100
F03 300000 300000 4.48E5 2.29E6 0
F04 300000 300000 -449.9996 -449.96 0
F05 300000 300000 1553.32 2315.67 0
F06 300000 300000 390.00 410.95 0
F07 300000 300000 4516.28 4516.28 0
F08 300000 300000 -119.14 -119.06 0
F09 300000 300000 -319.05 -260.31 0
F10 300000 300000 -173.88 -167.66 0
F11 300000 300000 95.47 117.13 0
F12 300000 300000 2.75E5 3.82E6 0
F13 300000 300000 -116.52 -115.85 0
F14 300000 300000 -287.01 -286.91 0
Table 3. Results for the 14 Functions for the CAEP(FIS-
Nsd) configuration.
Function MIN FEs AVG FEs
BEST
Fitness
AVG
Fitness
Success
%
F01 10320 10886.4 -450 -450 100
F02 151020 162852 -450 -450 100
F03 300000 300000 3.26E5 4.45E5 0
F04 268268 294892,8 -450 -449.996 36
F05 300000 300000 1964.69 2412.47 0
F06 300000 300000 390.34 397.56 0
F07 300000 300000 4516.28 4516.28 0
F08 300000 300000 -119.29 -119.10 0
F09 300000 300000 212.17 -186.38 0
F10 300000 300000 -173.91 -159.71 0
F11 300000 300000 128.62 129.48 0
F12 300000 300000 1562.40 14900.4 0
F13 300000 300000 -116.78 -116.42 0
F14 300000 300000 -286.68 -286.64 0
7 FINAL REMARKS
We observed that the addition of a fuzzy inference
system to regulate the intensity of the influence
function applied to the individuals alone can
improve the performance of the CAEP(Nsd)
configuration. However, the contribution is only
perceived in unimodal functions, as can be seen in
the results. For improving the performance in
multimodal functions, we envision the addition of
other knowledge sources, such as historical,
topographic and domain knowledge, and fuzzy
influence functions that make use of these
knowledge sources to the CAEP framework as
future work.
REFERENCES
Chung, C., Reynolds, R. G., 1997. Fuzzy Approaches to
Acquiring Experimental Knowledge in Cultural
Algorithms. In Proceedings of the 9th International
Conference on Tools with Artificial Intelligence
(ICTAI), IEEE Computer Society Washington, DC.
Fogel, D. B., 1995. Evolutionary Computation: Toward a
New Philosophy of Machine Intelligence, IEEE Press,
Piscataway, NJ.
Fogel, D. B., Ghozeil, A., 1996. Using Fitness
Distributions to Design More Efficient Evolutionary
Computations. In, Proceedings of IEEE International
Conference on Evolutionary Computation.
Reynolds, R. G., 1994. An Introduction to Cultural
Algorithms. In Proceedings of the Third Annual
Conference on Evolutionary Programming, February
24-26, San Diego, California.
Reynolds, R. G., Peng, B., Whallon, R., 2005. Emergent
Social Structures in Cultural Algorithms. In
Proceedings of the Annual Conference of the North
American Association for Computational Social and
Organizational Science, Notre Dame, Indiana.
Rodrigues, N. M., 2007. Um Algoritmo Cultural para
Problemas de Despacho de Energia Elétrica, Master
Dissertation, Universidade Estadual de Maringá,
Maringá, Paraná, Brazil.
Saleem, S. M., Reynolds, R. G., 2000. Cultural
Algorithms in Dynamic Environments. In Proceedings
of the 2000 Congress on Evolutionary Computation,
La Jolla, CA.
Saleem, S. M., Reynolds, R. G., 2001. Knowledge-Based
Solution to Dynamic Optimization Problems using
Cultural Algorithms. Ph.D. Thesis, Wayne State
University, Detroit, Michigan.
Suganthan, P. N., Hansen, N., Liang, J. J., Deb, K., Chen,
Y. P., Auger, A., Tiwari, S., 2005. Problem
Definitions and Evaluation Criteria for the CEC 2005
Special Session on Real-Parameter Optimization,
Technical report, Nanyang Technological University,
Singapore.
Zhu, S., Reynolds, R. G., 1998. Fuzzy Cultural Algorithms
with Evolutionary Programming for Real-Valued
Function Optimization, Ph.D. Thesis, Wayne State
University, Detroit, MI.
A FUZZY-CONTROLLED INFLUENCE FUNCTION FOR THE CULTURAL ALGORITHM WITH EVOLUTIONARY
PROGRAMMING APPLIED TO REAL-VALUED FUNCTION OPTIMIZATION - Intelligent Control Systems and
Optimization
245