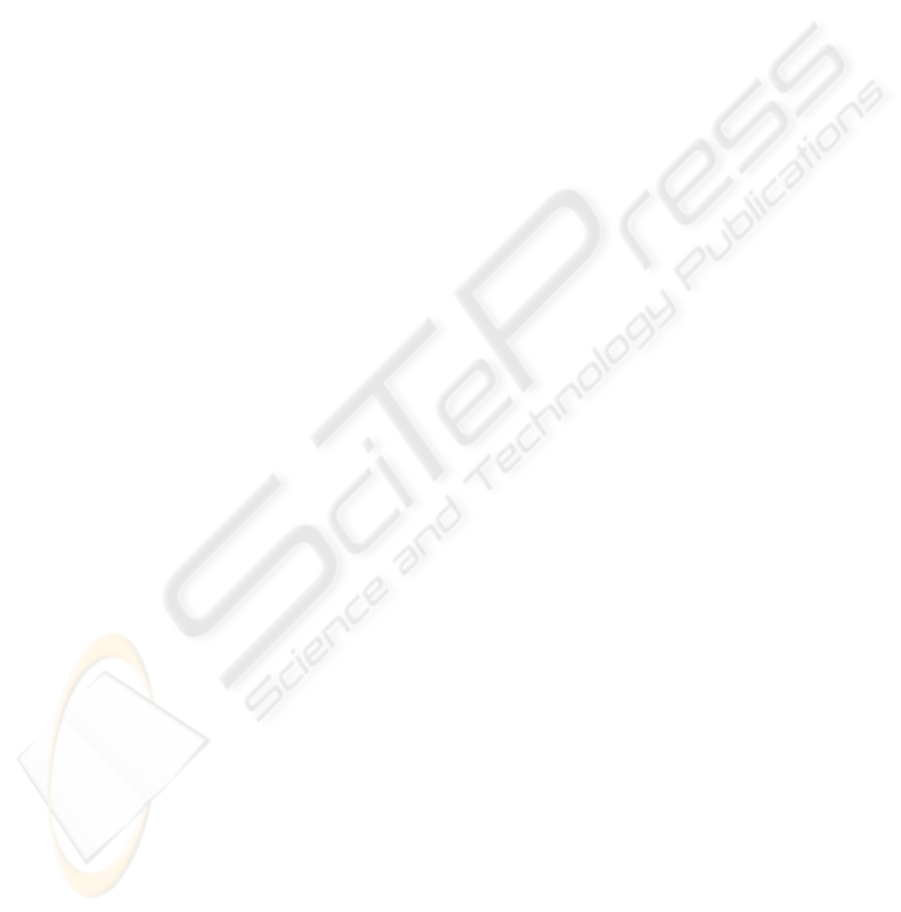
In general, comparison of the two recursive real-
isations of the EBPM reveals that it is the REBPM
2
which produces the more accurate results overall.
Moreover, it is noted that in the case of the REBPM
1
the algorithm diverged twice, producing two outliers.
In terms of the computational burden, the time re-
quired by the REBPM
2
is slightly greater when com-
pared to that of REBPM
1
, i.e. the former technique is
faster by approximately 17% with respect to the latter
method.
In general, the experiments carried out seem to
suggest that the REBPM
2
is more advantageous than
the REBPM
1
due to a simpler initialisation, greater
robustness and an absence of convergence problems,
at least under the conditions considered here.
6 CONCLUSIONS
A recursive realisation of the extended bilinear
parametrisation method for the identification of dy-
namical linear discrete-time time-invariant single-
input single-output errors-in-variables models has
been proposed. Two alternative approaches for the
online update of the pseudo-inverse of the extended
observation covariance matrix have been considered.
The first approach is based on the pseudo-inverse ma-
trix lemma, whereas the second is constructed within
the framework of the extended instrumental variables
technique. For the cases considered, the two resulting
algorithms appear to be relatively robust and they are
also found to yield precise estimates of the model pa-
rameters. Results suggest that the instrumental vari-
ables based approach would appear to be the superior
of the two developed algorithms.
REFERENCES
Bj¨orck,
˚
A. (1996). Numerical Methods for Least Squares
Problems. SIAM, Philadelphia.
Ekman, M. (2005a). Identification of linear systems with
errors in variables using separable nonlinear least
squares. In Proc. of 16th IFAC World Congress,
Prague, Czech Republic.
Ekman, M. (2005b). Modeling and Control of Bilinear Sys-
tems: Applications to the Activated Sludge Process.
PhD thesis, Uppsala University, Sweden.
Ekman, M., Hong, M., and S¨oderstr¨om, T. (2006). A sep-
arable nonlinear least-squares approach for identifica-
tion of linear systems with errors in variables. In 14th
IFAC Symp. on System Identification, Newcastle, Aus-
tralia.
Feng, D., Zhang, H., Zhang, X., and Bao, Z. (2001). An
extended recursive least-squares algorithm. Signal
Proc., 81(5):1075–1081.
Friedlander, B. (1984). The overdetermined recursive in-
strumental variable method. IEEE Trans. on Auto-
matic Control, 29(4):353–356.
Ikenoue, M., Kanae, S., Yang, Z., and Wada, K.
(2008). Bias-compensation based method for errors-
in-variables model identification. In Proc. of 17th
IFAC World Congress, pages 1360–1365, Seul, South
Korea.
Larkowski, T., Linden, J. G., Vinsonneau, B., and Burn-
ham, K. J. (2008). Identification of errors-in-variables
systems via extended compensated least squares for
the case of coloured output noise. In The 19th Int.
Conf. on Systems Engineering, pages 71–76, Las Ve-
gas, USA.
Linden, J. G. (2008). Algorithms for recursive Frisch
scheme identification and errors-in-variables filter-
ing. PhD thesis, Coventry University, UK.
Ljung, L. (1999). System Identification - Theory for the
User. Prentice Hall PTR, New Jersey, USA, 2nd edi-
tion.
Mahata, K. (2007). An improved bias-compensation ap-
proach for errors-in-variables model identification.
Automatica, 43(8):1339–1354.
Markovsky, I. and Van Huffel, S. (2007). Overview of to-
tal least-squares methods. Signal Proc., 87(10):2283–
2302.
S¨oderstr¨om, T. (2007). Errors-in-variables methods in sys-
tem identification. Automatica, 43(6):939–958.
RECURSIVE EXTENDED COMPENSATED LEAST SQUARES BASED ALGORITHM FOR
ERRORS-IN-VARIABLES IDENTIFICATION
147