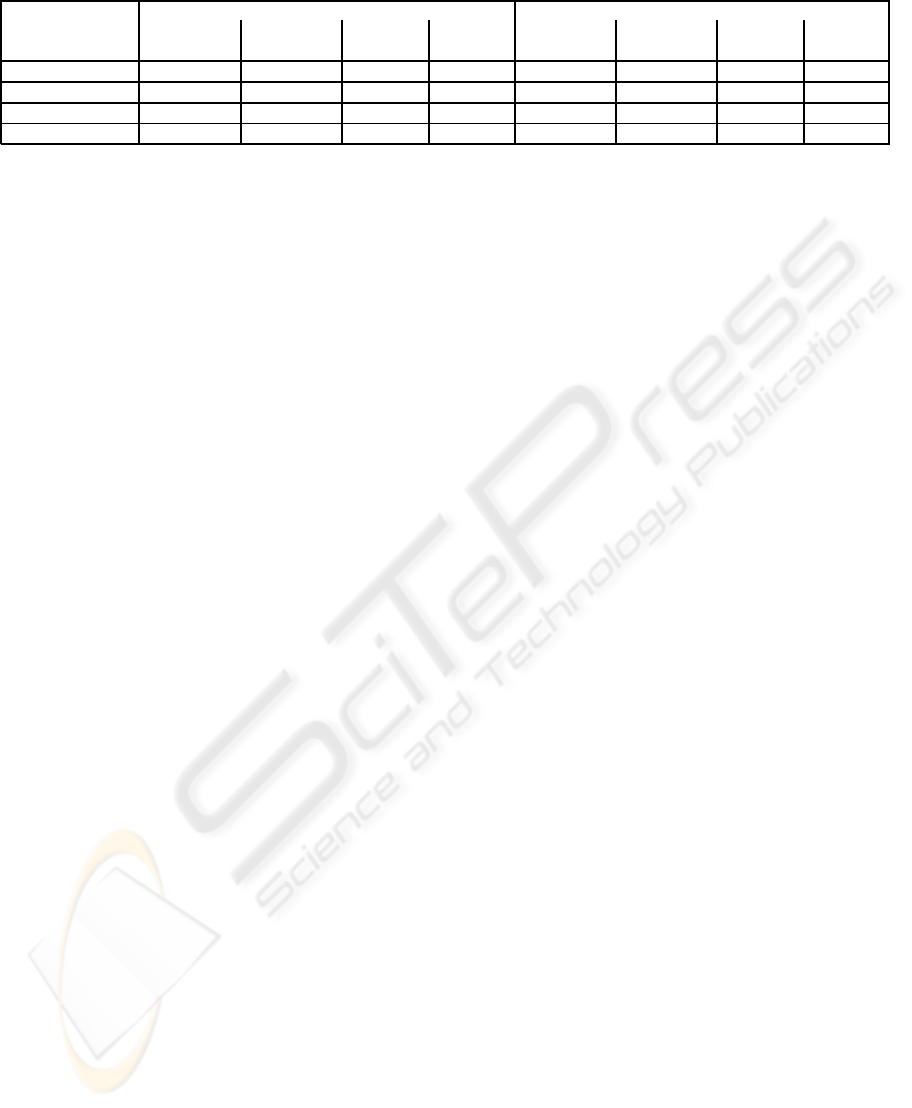
Table 3: Comparison of DPCA and DO approaches.
DPCA DO
Abrupt Gradual Actuator Multiple Abrupt Gradual Actuator Multiple
Metrics Fault(TT
2
) Fault(TT
2
) faults faults Fault(TT
2
) Fault(TT
2
) faults faults
Detection (s) 0 10−14 9−18 0 0 5 5−8 0
Isolation X X X - X X X X
Explanation X X X - X X X X
False alarm (%) 0 0 14.13 0 0 0 9.94 0
be complicated. In this work, DO shows a quicker
detection (i.e. almost the half of detection time) than
DPCA when faults in both actuators are implemented
at different times. For these faults, DO presents a
lower false alarm rate than DPCA (Table 3).
On the other hand, both FDI methods can de-
tect multiple faults which are implemented in all sen-
sors. However, DPCA can not isolate correctly when
several faults have been implemented. According to
computational requirements, the design of DO needs
greater computational resources. The training stage
of this method is more complicated than the DPCA
training; DO requires firstly a reliable ARX model
which must be translated to a state space model. Fur-
thermore, each fault case must be modeled in a partic-
ular state space model. Once the fault model is known
with high reliability, is designed a state observer; par-
ticularly in this work all models (fault cases and nor-
mal operating) are obtained in parallel. On the other
hand, the DPCA training is quickly executed once his-
torical data of the normal operating point are known.
6 CONCLUSIONS
A comparison between the Dynamic Principal Com-
ponent Analysis (DPCA) and a set of Diagnostic Ob-
servers (DO) under same experimental data from an
industrial Heat Exchanger (HE) is presented. DPCA
do very well on fast detection of abnormal situations,
it is easier to implement in industrial applications. A
process model was not required; however, a broad
acquisition of the historical measurements is needed.
Respect to false alarm rate, DPCA showed 42% more
of false alarms than DO for actuator faults.
DO presents a quicker detection than DPCA ([4−
10] seconds lower), DO requires an accurate state
space model of the process. Furthermore, each fault
case must be modeled. If the model is not reliable,
DO can not detect a fault correctly. Due to HE is
inherently a nonlinear system, it is more difficult to
implement a FDI method based on quantitative mod-
els. Finally, DPCA can not identify multiple faults
whereas DO can.
REFERENCES
Aitouche, A., Maquin, D., and Busson, F. (1998). Multiple
Sensor Fault Detection in Heat Exchanger Systems .
In Proc. of Int. Conf. on Ctrl. Appl., pages 741–745,
Trieste, Italy.
Ball´e, P., Fischer, M., F¨ussel, D., and Isermann, R. (1997).
Integrated Control, Diagnosis and Reconfiguration of
a Heat Exchanger . In American Control Conference,
pages 922–926, Albuquerque, New Mexico.
Caccavale, F. and Villani, L. (2004). An Adaptive Observer
for Fault Diagnosis Nonlinear Discrete-Time Systems.
In American Control Conference, pages 2463–2468,
Boston, Massachusetts.
Detroja, K., Gudi, R., and Patwardhan, S. (2005). Plant-
wide Detection and Diagnosis using Correspondece
Analysis. Control Engineering Practice.
Hotelling, H. (1993). Analysis of a Complex of Statistical
Variables into Principal Components. J. Educ. Psy-
chol., 24.
Isermann, R. (2006). Fault-Diagnosis Systems. Springer,
Germany, 1
st
edition.
Jackson, J. and Mudholkar, G. (1979). Control Procedures
for Residuals Associated with Principal Component
Analysis. Technometrics, 21:341–349.
Krishnan, R. and Pappa, N. (2005). Real Time Fault Diag-
nosis for a Heat Exchanger A Model Based Approach.
In IEEE Indicon Conference, pages 78–82, Chennai,
India.
Ku, W., Storer, R., and Georgakis, C. (1995). Disturbance
Detection and Isolation by Dinamic Principal Compo-
nents Analysis. Chemometrics and Intelligent. Lab.
Syst., 30:179–196.
Miller, P., Swanson, R., and Heckler, C. (1998). Contri-
bution Plots: A Missing Link in Multivariate Quality
Control. Appl. Math. and Comp. Sci., 4(8):775–792.
Mina, J. and Verde, C. (2007). Fault Detection for MIMO
Systems Integrating Multivariate Statistical Analysis
and Identification Methods . In American Control
Conference, pages 3234–3239, New York City, USA.
Morales-Menendez, R., Freitas, N. D., and Poole, D.
(2003). State Estimation and Control of Industrial
Processes using Particles Filters. In American Control
Conference, pages 579–584, Denver, Colorado, USA.
Simmani, S. and Patton, R. (2008). Fault Diagnosis of an In-
dustrial Gas Turbine prototype Using a System Iden-
tification Approach. Ctrl. Eng. Prac., (16):769–786.
Verde, C. (2001). Multi-leak Detection and Isolation in
Fluid Pipelines . Control Eng. Practice, 9:673–682.
ICINCO 2009 - 6th International Conference on Informatics in Control, Automation and Robotics
270