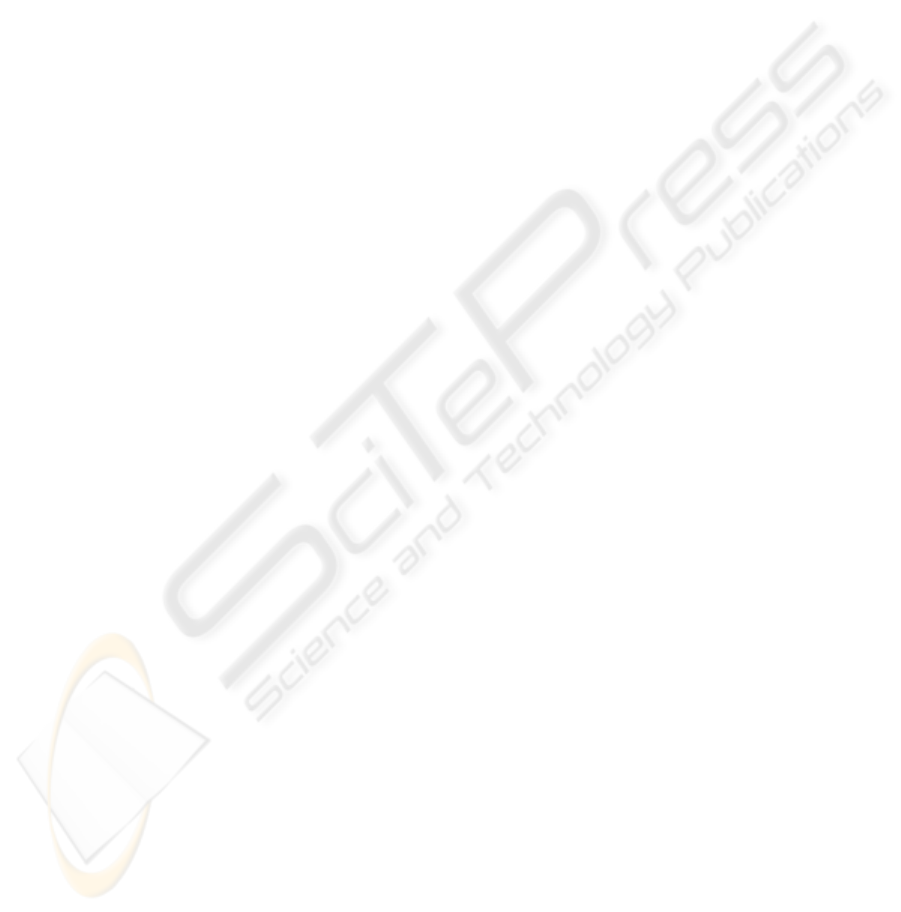
the WB of the camera in much the same way as the
proprietary mechanism in certain situations; see
Figure 4 (left), for example. In contrast, other
situations can produce deviations in WB settings
between the subnet and proprietary mechanisms; see
Figure 4 (right), for example. The size of WB steps
should also be considered, as too large a step size
will introduce over-correction and, ultimately, a
ping-ponging of the WB parameter as the subnet
slowly narrows in on the correct value; on the other
hand, too small a step size will lead to a very slowly
adjusting WB. Finally, in the current network,
following a change in the white-balance parameter,
it was necessary to insert a short delay before
another step could be made; this was needed to
allow the changes in image color due to the WB
parameter change to spread through the various
neural layers.
4 EXPOSURE CONTROL
One of the most remarkable properties of the human
vision system is its ability to function over a
strikingly large range of luminance conditions, a
span of approximately 10 billion to 1 (Dowling,
1987). The human eye has essentially two ways of
dealing with the variation it experiences in day-to-
day luminance levels. (1) The pupil can reduce its
area by a factor of approximately 16 due to changes
in ambient illumination. (2) The circuitry in the
retina is specially designed to handle two general
lighting conditions: dim light, primarily handled by
the rod-pathway in the retina; and bright light,
handled by the cone-pathway in the retina.
Video cameras, on the other hand, do not have
the luxury of such robust input mechanisms.
Nevertheless, various methods have been developed
to allow cameras to function under a rather
impressive span of luminance levels – at least when
all things are considered. The camera used for the
present study employs two primary parameters that
can be adjusted to compensate for luminance levels:
iris size and gain control.
The network control of exposure is similar to that
of WB in that a conceptually simple subnet
progressively computes various properties of the
incoming image which allows it to ‘step’ the
relevant parameter towards optimizing some
computation. The computation that is optimized in
exposure control is contrast; too much light entering
and the image gets ‘washed-out’; too little light
creates an underexposed image. This mechanism in
particular will likely be very important to robotic
vision systems using biologically neural models, as
contrast has shown to play a particularly critical role
in the neural computations that take place in the
primate visual cortex (Sceniak et al., 1999).
As with the WB subnet, the functioning of the
exposure subnet is conceptually very simple.
Essentially, the subnet attempts to maximize the
contrast of two spatially adjacent areas using the on-
and off-center ganglion cells. Recall that in the
neural model of the retina (and the biological retina)
contrast plays a specific role for two classes of
neurons, bipolar cells and ganglion cells. That is,
these cells compute an activation that highlights high
contrast areas of the image. Consequently, much of
the work required for computing our exposure
control function is already implemented.
The remaining work is performed by two
independent subnets, an off-subnet and an on-
subnet. Each subnet first computes a local
maximum of the incoming ganglion cell activations
(on-center ganglion cells will have higher
activations in bright areas, especially if it is adjacent
to a dark area, and vice-versa for off-center ganglion
cells). This maximum is then averaged across the
image to produce an exposure step-value similar in
nature to the WB step-value, with one difference, the
step value for the exposure control must work to
control both the iris and gain of the camera, which is
handled by a simple scheme: changes to the iris take
precedence over changes to gain, which instead
serves to fine-tune the exposure using small step-
values.
Sample results of the exposure subnet are shown
in Figure 5 for both bright-light conditions (left) and
dim-light conditions (right). Exposure values in
dim-light conditions closely follow those computed
by the proprietary control mechanisms. However,
significant differences can be seen in bright-light
conditions. This is likely due to the goal of the
exposure subnet to extract a maximum contrast
signal, which may only occur in a portion of the
image, as opposed to enhancing a global contrast
signal. This difference is most prominent around the
wheel in Figure 5 (left) where the proprietary
mechanism introduces spectral highlighting on the
rubber (black) portion of the wheel; whereas when
under the exposure subnet’s control, the gain is
weaker to allow the natural blackness of the wheel to
maximize the contrast between the plastic (white)
and rubber (black) portions.
AUTONOMOUS CAMERA CONTROL BY NEURAL MODELS IN ROBOTIC VISION SYSTEMS
309