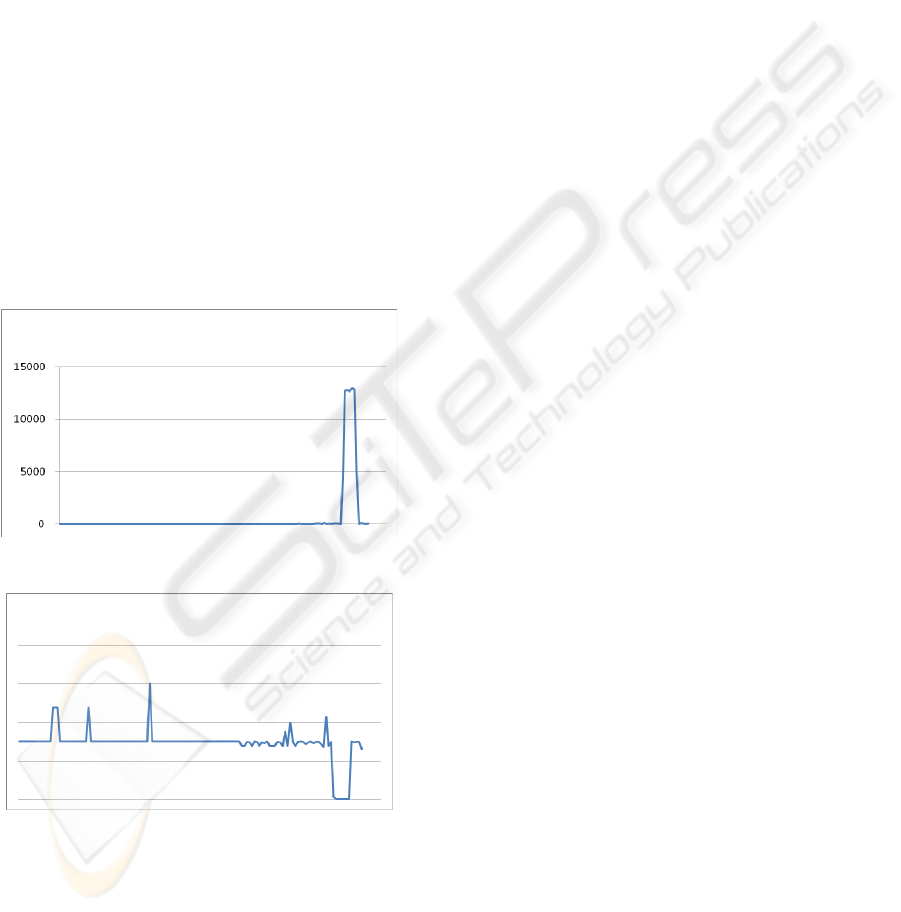
during the flash crowds is much smaller than the
number of distinct clients. But, DDoS attacks
requests come from clients widely distributed across
clusters in the Internet; (iii) a large number of
clusters active during flash crowds had also visited
the sites before the event. However, in the case of
DDoS attacks, an overwhelming majority of the
client clusters that generate requests are new clusters
not seen by the site before the attack.
4.3 Experimentation
In the simulation, we use the 2000 DARPA Data Set
which includes a DDoS attack run by a novice
attacker (MIT Lincoln Lab, 2000). This attack
scenario is carried out over five phases. In phase 1
and 2, the attacker sends ICMP packet to probe of
IP’s to look for the sadmind daemon running on
Solaris hosts. The attacker installs Trojan mstream
DDoS software on hosts in Phase 3 and 4. In Phase,
the attacker launches the DDoS attack. The number
of packets and randomness variation shows in figure
2 and 3.
Figure 2: The Number of packets.
Figure3: The randomness of source IP address in
Destination IP address cluster.
5 CONCLUSIONS
In this paper, we propose discrimination methods
that classify cluster of traffic behaviour of flash
crowds and DDoS attacks such as traffic pattern and
characteristics and check cluster randomness. The
main research objectives are to find way to
proactively resolve problems such as DDoS attacks
by detection and resolving attacks in their early
development stages.
In the future work, we expect to analyze network
traffic more effectively by extracting more variables
and develop an advanced detection algorithm. We
plan to find a way of mitigating DDoS attacks by
using this early detection.
REFERENCES
U. Herman, 2006. Flash Crowd Prediction, Master’s
Thesis, Warsaw University.
SLASHDOT. http://slashdot.org.
Gordon, L.A., Loeb, M.P., Lucyshn, W., Richardson, R.,
2004. CSI/FBI computer crime and security survey. In
Computer Security Inst..2004
G. Carl and G. Kesidis, Denial-of-Service Attack
Detection Techniques, IEEE Internet Computing 2006,
IEEE Computer Society.
Kenneth Theriault, Daniel Vukelich, Wilson Farrell,
Derrick Kong, John Lowry, Network Traffic Analysis
Using Behavior-Based Clustering
Krishnamurthy, B., Wang, J., 2000. On network-aware
clustering of web clients. In ACM SIGCOMM’00.
Jung, J., Krishnamurthy, B., Rabinovich, M., 2002. Flash
crowds and denial of service attacks: Characterization
and implications for CDNs and web sites. In WWW
2002.
A. McGregor, M. Hall, P. Lorier, and J. Brunskill., 2004.
Flow Clustering Using Machine Learning Techniques.
In PAM 2004, Antibes Juan-les-Pins, France.
S. Zander, T. Nguyen, and G. Armitage., 2005. Automated
Traffic Classification and Application Identification
using Machine Learning. In LCN’05, Sydney,
Australia.
He, Y., Chen, W., Xiao, B., 2005. Detecting SYN flooding
attacks near innocent side. In MSN 2005.
Wang, H., Zhang, D., Shin, K.G., 2002. Detecting SYN
flooding attacks. In INFOCOM2002.
Feinstein, L., Schackenberg, D., Balupari, R., Kindred, D.,
2003. Statistical approaches to DDoS attack detection
and response. In DISCEX 2003.
Peng, T., Leckie, C., Rnmamohanarao, K, 2004.,
Proactively detecting Distributed Denial of Service
attacks using source IP address monitoring.
Networking 2004.
H. Park et al, Distinguishing between FE and DDoS Using
Randomness Check, In ISC 2008.
Yan Hu, Dah-Mng Chiu, and John C.S. Lui, Entropy
Based Flow Aggregation, In Networking 2006.
BEHAVIOR-BASED CLUSTERING FOR DISCRIMINATION BETWEEN FLASH CROWDS AND DDoS ATTACKS
143