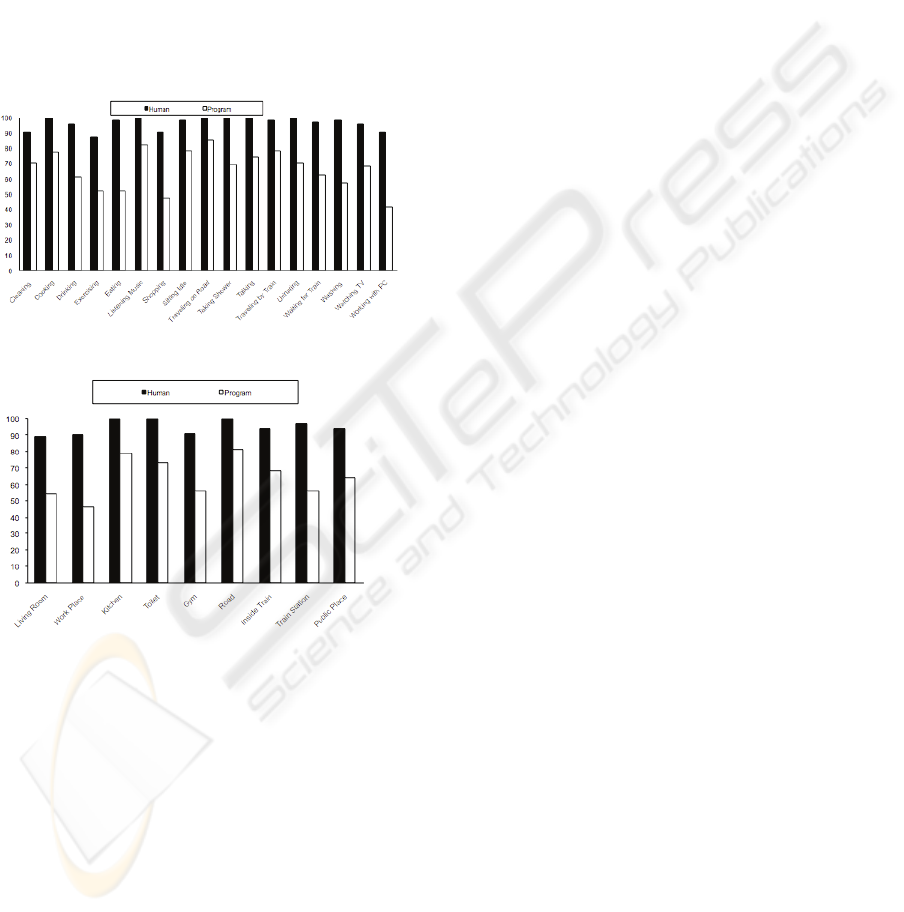
expected affinity to a particular activity and location.
Ten human (i.e., five male, five female) judges were
engaged to listen to the test signals and judge an
input signal to infer the activity from the given list
of 17 activities (i.e., forced choice judgment) as well
as the possible location of that activity from the list
of given nine locations of our choice. Each judge
was given all the 17 groups of signals to listen and
assess. Therefore a judge listen each test signal to
infer the location and activity that the given signal
seemed most likely to be associated with. In the
same way the signals were given to the system to
process. Recognition results for activity and location
are presented in Figure 2 and 3 respectively.
Figure 2: Comparisons of recognition rates for 17
activities of our interest with respect to human judges.
Figure 3: Comparisons of recognition rates for 9 locations
of our interest with respect to human judges.
The recognition accuracy for activity and
location is encouraging with most being above than
66% and 64% respectively. From Figure 4 and 5,
we notice that humans are skillful in recognizing the
activity and location from sounds (i.e., for humans’
the average recognition accuracy of activity and
location is 96% and 95% respectively). It is also
evident that the system receives the highest accuracy
(i.e., 85% and 81% respectively) to detect “traveling
on road” activity and “road” location respectively,
which is a great achievement and pioneer effort in
this research that no previous research attempted to
infer outdoor activities with sound cues. The correct
classification of sounds related to activity “working
with pc” and location “work place” were found to be
very challenging due to the sounds’ shortness in
duration and weakness in strength, hence the
increased frequency for them to be wrongly
classified as ‘wind’ type object recognition.
5 CONCLUSIONS
In this paper, we described a novel acoustic indoor
and outdoor activities monitoring system that
automatically detects and classifies 17 major
activities usually occur at daily life. Carefully
designed HMM parameters using MFCC features
are used for accurate and robust sound based activity
and location classification with the help of
commonsense knowledgebase. Preliminary results
are encouraging with the accuracy rate for outdoor
and indoor sound categories for activities being
above 67% and 61% respectively. We believe that
integrating sensors into the system will also enable
acquire better understanding of human activities.
The enhanced system will be shortly tested in a full-
blown trial on the neediest elderly peoples residing
alone within the cities of Tokyo evaluating its
suitability as a benevolent behavior understanding
system carried by them.
REFERENCES
Kam, A. H., Zhang, J., Liu, N., and Shue, L., 2005.
Bathroom Activity Monitoring Based on Sound. In
PERVASIVE’05, 3rd International Conf. on Pervasive
Computing. Germany, LNCS 3468/2005, pp. 47-61.
Temko, A., Nadeu, C., 2005. Classification of meeting-
room acoustic events with Support Vector Machines
and Confusion-based Clustering. In ICASSP’05, pp.
505-508.
Wang, D., and Brown, G., 2006. Computational Auditory
Scene Analysis: Principles, Algorithms and
Applications. Wiley-IEEE
Okuno, H.G., Ogata, T., Komatani, K., and Nakadai, K.,
2004. Computational Auditory Scene Analysis and Its
Application to Robot Audition. In International
Conference on Informatics Research for Development
of Knowledge Society Infrastructure, pp., 73–80
Eronen, A., Tuomi, J., Klapuri, A., Fagerlund, S., Sorsa,
T., Lorho, G., and Huopaniemi, J., 2003. Audio-based
Context Awareness-Acoustic Modeling and Perceptual
Evaluation. In ICASSP '03, Int’l Conference on
Acoustics, Speech, and Signal Processing, pp. 529-532
Hatzivassiloglou, V. and McKeown, K. R., 1997.
Predicting the Semantic Orientation of Adjectives. In 35th
annual meeting on ACL, pp.174-181
SIGMAP 2009 - International Conference on Signal Processing and Multimedia Applications
196