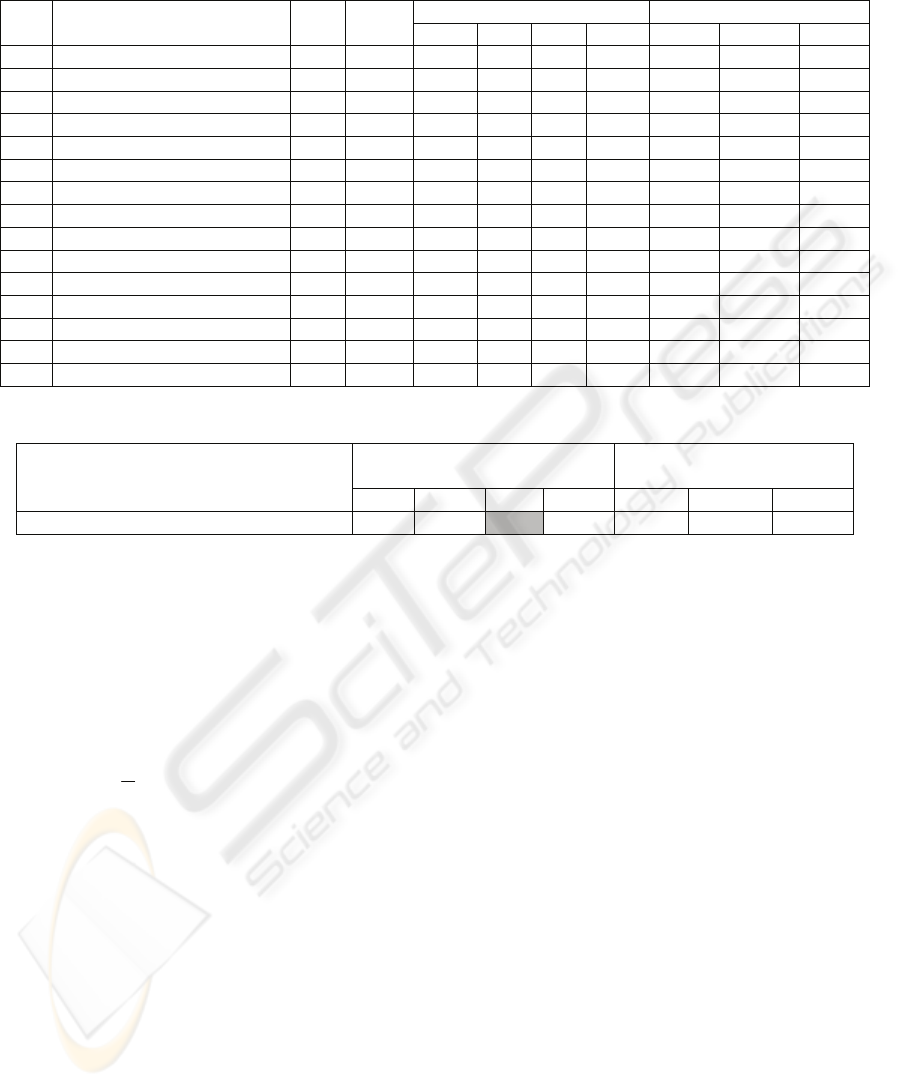
Table 2: Comparison of the HTER between the likelihood ratio based fusion and the simple sum rule.
No. Fusion candidates Face Voice
(log-likelihood ratio) Simple sum rule
1 2 4 8 zscore Min-max Tanh
1 (FH,MLP)(LFCC,GMM) 1,883 1,148 1,108 0,426 0,565 0,297 0,795 0,862 0,737
2 (FH,MLP)(PAC,GMM) 1,883 6,208 1,441 1,097 0,992 1,079 1,133 1,161 1,026
3 (FH,MLP)(SSC,GMM) 1,883 4,494 1,339 1,054 0,962 0,963 0,868 1,072 0,778
4 (DCTs,GMM)(LFCC,GMM) 4,250 1,148 0,574 0,571 0,575 0,568 0,526 0,492 0,583
5 (DCTs,GMM)(PAC,GMM) 4,250 6,208 1,417 1,331 1,428 1,422 1,436 1,417 1,376
6 (DCTs,GMM)(SSC,GMM) 4,250 4,494 1,201 1,197 1,152 1,155 1,144 1,218 1,132
7 (DCTb,GMM)(LFCC,GMM) 1,734 1,148 0,499 0,476 0,479 0,486 0,553 0,503 0,467
8 (DCTb,GMM)(PAC,GMM) 1,734 6,208 1,106 1,087 1,068 1,066 1,127 1,093 1,661
9 (DCTb,GMM)(SSC,GMM) 1,734 4,494 0,764 0,747 0,849 0,841 0,747 0,720 0,733
10 (DCTs,MLP)(LFCC,GMM) 3,363 1,148 1,193 0,574 0,597 0,575 0,841 0,972 0,728
11 (DCTs,MLP)(PAC,GMM) 3,363 6,208 1,982 1,000 0,894 0,961 1,119 1,413 0,822
12 (DCTs,MLP)(SSC,GMM) 3,363 4,494 1,721 1,111 0,909 0,965 1,372 1,594 1,036
13 (DCTb,MLP)(LFCC,GMM) 6,225 1,148 1,693 0,719 0,609 0,682 1,621 3,278 0,874
14 (DCTb,MLP)(PAC,GMM) 6,225 6,208 3,547 2,579 2,167 2,410 3,653 4,121 2,623
15 (DCTb,MLP)(SSC,GMM) 6,225 4,494 3,722 2,038 1,671 1,831 2,883 4,329 2,058
Table 3: Comparison of the average of the HTER between the likelihood ratio based fusion and the simple sum rule.
(log-likelihood ratio)
Number of mixtures
Simple sum rule
1 2 4 8 zscore Min-max Tanh
Average of HTER of the 15 combinations 1,554 1,067 0,994 1,020 1,616 1,321 1,109
calculated as follows:
()
Δ
ΔΔ
EERminarg
*
=
(7)
where
() ()()
ΔΔ
FRRFAR
2
1
EER +=
(8)
where FAR and FRR designate the false acceptance
rate and false rejection rate respectively.
We can notice from table 2, that using LR test with
only one Gaussian gives the worst results. This is
expected because only one Gaussian is not sufficient
to estimate efficiently the score distributions.
However a consistent performance improvement is
obtained by increasing the number of Gaussians to 4
where the best performance are abstained, good
results are obtained with eight Gaussians but it is
clear that 8 Gaussians are more than enough to
estimate the client and impostor distributions and
also this is due to the lack of data.
To summarize Table 2, we have computed the
average HTER of the 15 possible matcher
combinations, the results are summarized in Table 3.
It is so clear from this table the superiority of the LR
test using GMM for modelling the genuine and
impostor classes. We can conclude that although the
sum rule can obtain a better performance with an
appropriate normalisation (min-max or tanh in our
case) the gain compared to the LR is not significant.
4 CONCLUSIONS
In this paper, we have analyzed the performance of
combining face and voice biometrics at the score
level using the LR classifier. Our experiments on the
publicly available scores of the XM2VTS
Benchmark database show a consistent high
performance regardless of the score nature of
different speech and face matchers. As a perspective
of this work is the introduction of user specific
information jointly with the LR test and GMM score
modelling.
REFERENCES
Alsaade, F. 2008. Score-Level Fusion for Multimodal
SIGMAP 2009 - International Conference on Signal Processing and Multimedia Applications
60