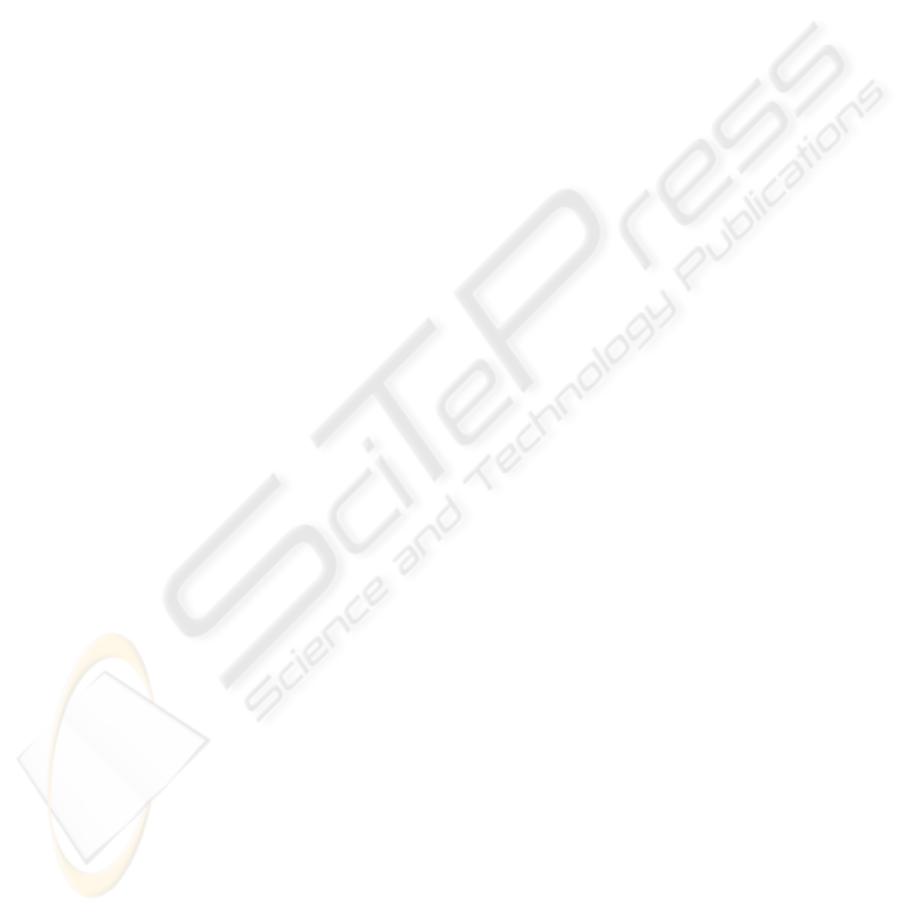
6 CONCLUSIONS AND FUTURE
WORK
In the work reported in this paper we have proposed
an approach to detect MCs in digital mammograms
using the DT-CWT. The approach consists of the
DT-CWT application to obtain a mammogram’s
subband decomposition, mammogram’s denoising
by applying an optimal threshold at each
decomposition level, suppression of mammogram’s
low-frequencies, application of morphological
operators to enhanced MCs visualization, and
finally, the reconstruction of the mammogram. The
results obtained using the DT-CWT are compared to
the results obtained using the SWT and the Top-Hat
transformations. The proposed approach shows the
best performance to detect MCs in mammograms.
The SWT detects the MCs but other details are also
observed as MCs. Another inconvenient presented
by the SWT is the computational complexity, O(n
2
),
in contrast, the computational complexity of the DT-
CWT is O(2n) only. From results obtained
morphological filtering is the worst method to detect
MCs, because MCs are not well appreciated, in
addition tissue and breast glands are presented in the
reconstructed mammogram. The approach presented
in this work can be used as a basis to develop an
automatic diagnostic system to aid the results on
mammogram’s interpretation and to get an earlier
and opportune diagnostic for breast cancer.
REFERENCES
Akay, M., 1997. Wavelet applications in medicine, IEEE
Spectrum, 34(5).
Alarcon-Aquino, V. 2003. Anomaly Detection and
Prediction in Communication Networks using Wavelet
Transforms, PhD Thesis, Imperial College London,
UK.
Azzalini A., Farge M., Schneider K., 2005. Nonlinear
wavelet thresholding: A recursive method to determine
the optimal denoising threshold, Applied and
Computational Harmonic Analysis, Elsevier, 18, pp.
177– 185.
Burrus, C. S., Ramesh A. Gopinath, 1998. Introduction to
Wavelets and Wavelet Transforms, Prentice Hall.
Cheng, H., Lui, YM, Feiimanis, RI., 1998. A novel
approach to microcalcification detection using fuzzy
logic techniques. IEEE Transactions on Medical
Imaging, 17 (6).
Gonzalez, Rafael C., Richard E. Woods, 2001. Digital
Image Processing, Second Edition, Prentice Hall.
Jamarani, S.M.H.; Rezai-rad, G.; Behnam, H.; 2006. A
Novel Method for Breast Cancer Prognosis Using
Wavelet Packet Based Neural Network”. IEEE-EMBS
Engineering in Medicine and Biology Society, 27th
Annual International Conference
Jansen M., Bultheel A.,1999. Asymptotic behavior of the
minimum mean squared error threshold for noisy
wavelet coefficients of piecewise smooth signals,
Katholieke Universiteit Leuven. Department of
Computer Science. Belgium.
Karahaliou, A. N., et al., 2008. Breast Cancer Diagnosis:
Analyzing Texture of Tissue Surrounding
Microcalcifications, IEEE Transactions on
Information Technology in Biomedicine, 12 (6).
Melloul, M., Joscowicz, L., 2002. Segmentation of
microcalcification in X-ray mammograms using
entropy thresholding, In Proceedings of the 16th
International Congress on Computer-Assisted
Radiology and Surgery CARS/Springer.
Mencattinni, A., M. Salmeri, R. Lojacono, M. Frigeno, F.
Caselli, 2008. Mammographic Images Enhancement
and Denoising for Breast Cancer Detection Using
Dyadic Wavelet processing, IEEE Transactions on
Instrumentation and Measurement, 57 (7).
Sebri, A., J. Malek, R. Tourki, 2007. Automated Breast
Cancer Diagnosis based on GVF-Snake Segmentation,
Wavelet Features Extraction and Neural Networks
Classification, Journal of Computer Science 3(8), 600-
607.
Selesnick, I., Baraniuk, R.G., Kingsbury, N.G., 2005. The
Dual-Tree Complex Wavelet Transform, IEEE Signal
Processing Magazine.
Shukla P. D. 2003. Complex Wavelet Transforms and
Their Applications. M.Phil. Thesis, University of
Strathclyde. Scotland, UK.
Strickland, R., Hahn., 1996. Wavelet Transforms for
Detecting Microcalcifications in Mammograms. IEEE
Transactions on Medical Imaging, 12 (4).
Suckling, J et al., 1994. The Mammographic Image
Analysis Society Digital Mammogram Database
Exerpta Medica. International Congress Series 1069
pp375-378
Taswell C. 2000. The What, How and Why of Wavelet
Shrinkage Denoising, Computing in Science &
Engineering.
Vazquez-Muñoz, K. 2006. Detection of
Microcalcifications in Digital Mammograms Using
the Dual-Tree Complex Wavelet Transform, BSc
Thesis, Universidad de las Americas Puebla.
Wang, T., Karayiannis, N., 1998. Detection of
microcalcification in Digital mammograms using
wavelets. IEEE Transactions on Medical Imaging, 17
(4).
Zhao, D., 1993. Rule-based morphological feature
extraction of microcalcifications in mammograms.
Proceedings of SPIE. Vol. 1905.
MAMMOGRAPHIC IMAGE ANALYSIS FOR BREAST CANCER DETECTION USING COMPLEX WAVELET
TRANSFORMS AND MORPHOLOGICAL OPERATORS
85