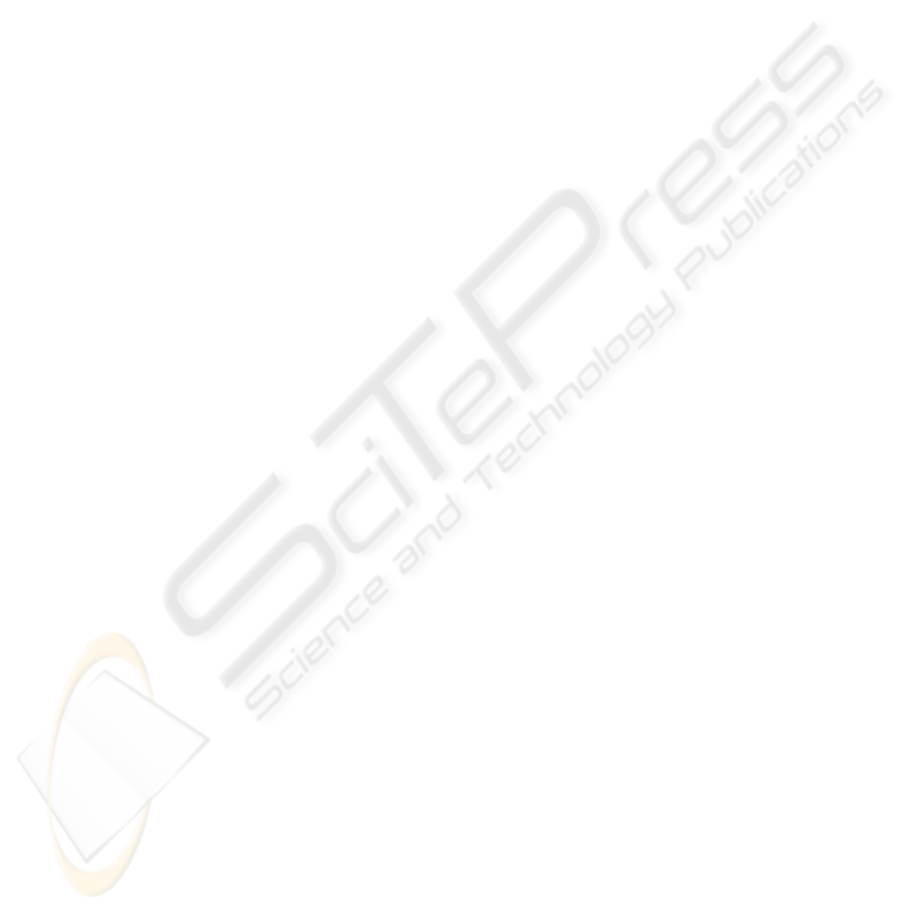
remains under inhibition of return due to the use of
spatial memory even when its 2D location in the view
frame and size has changed with respect to its last at-
tended instance. Figures 4(b) shows attention on the
second target whereas the ball is still under inhibition.
The subfigure (c) shows the view in the spatial mem-
ory after aligning its sensors to the real world. Fig-
ures 4 (d) and (e) demonstrate views in the real world
and the simulation framework after overt attention on
the second target while the activated model of the at-
tended object (the robot) can be seen in figure 4(f).
4 DISCUSSION
A conceptual framework of integrating a spatial mem-
ory with the vision procedures has been presented
here and the feasibility of using a 3D simulator as a
spatial memory is introduced. The area of integration
of vision and spatial memory, their interaction, and
cooperation needs to be explored further as there are
many issues to be resolved. For example the phys-
ical system can gain error of localization and orien-
tation over time due to inaccuracy in its sensors and
wheel slippages that lead to synchronization problem
between the real robot and its agent.
Using the spatial memory can increase the poten-
tials of vision in 3D world and intelligence in au-
tonomous decision making. Work needs to be done
for handling further complexities in the scenario. For
example, activation of the 3D models of objects will
be more useful when positions of movable objects
are not known in advance. Using the visual informa-
tion from the camera, the robot could recognize an
object and activate its whole model there. This can
be helpful in navigation planning while roaming in
known environments in which a bunch of known ob-
jects are moving around or located at arbitrary loca-
tions, for example 3D models of different types of ve-
hicles could be used for intelligent autonomous drive
on a known road map.
ACKNOWLEDGEMENTS
We gratefully acknowledge the funding of this work
by the German Research Foundation (DFG) under
grant Me 1289/12-1(AVRAM).
REFERENCES
Aivar, M. P., Hayhoe, M. M., Chizk, C. L., and Mruczek, R.
E. B. (2005). Spatial memory and saccadic targeting
in a natural task. Journal of Vision, 5:177–193.
Aziz, M. Z. and Mertsching, B. (2006). Color segmenta-
tion for a region-based attention model. In Workshop
Farbbildverarbeitung (FWS06), pages 74–83, Ilme-
nau - Germany.
Aziz, M. Z. and Mertsching, B. (2007). Color saliency and
inhibition using static and dynamic scenes in region
based visual attention. Attention in Cognitive Systems,
LNAI 4840, pages 234–250.
Aziz, M. Z. and Mertsching, B. (2008a). Fast and robust
generation of feature maps for region-based visual at-
tention. Transactions on Image Processing, 17:633–
644.
Aziz, M. Z. and Mertsching, B. (2008b). Visual search in
static and dynamic scenes using fine-grain top-down
visual attention. In ICVS 08, LNCS 5008, pages 3–12,
Santorini - Greece. Springer.
Burns, D. and Osfield, R. (2004). Tutorial: Open scene
graph. In Proceedings Virtual Reality, pages 265–265.
Cutzu, F. and Tsotsos, J. K. (2003). The selective tun-
ing model of attention: Psychophysical evidence for
a suppressive annulus around an attended item. Vision
Research, pages 205–219.
Hoshino, E., Taya, F., and Mogi, K. (2008). Memory forma-
tion of object representation: Natural scenes. R. Wang
et al. (eds.), Advances in Cognitive Neurodynamics,
pages 457–462.
Kutter, O., Hilker, C., Simon, A., and Mertsching, B.
(2008). Modeling and simulating mobile robots envi-
ronments. In 3rd International Conference on Com-
puter Graphics Theory and Applications (GRAPP
2008), Funchal - Portugal.
Mertsching, B., Aziz, M. Z., and Stemmer, R. (2005). De-
sign of a simulation framework for evaluation of robot
vision and manipulation algorithms. In International
Conference on System Simulation and Scientific Com-
puting, Beijing-China.
Moscovitch, M., Rosenbaum, R. S., Gilboa, A., Addis,
D. R., Westmacott, R., Grady, C., McAndrews, M. P.,
Levine, B., Black, S., Winocur1, G., and Nadel, L.
(2005). Functional neuroanatomy of remote episodic,
semantic and spatial memory: a unified account based
on multiple trace theory. Journal of Anatomy, pages
35–66.
Oman, C. M., Shebilske, W. L., Richards, J. T., Tubr
´
e, T. C.,
Bealli, A. C., and Natapoffi, A. (2000). Three dimen-
sional spatial memory and learning in real and virtual
environments. Spatial Cognition and Computation,
2:355–372.
Shelton, A. L. and Mcnamara, T. P. (2004). Spatial mem-
ory and perspective taking. Memory & Cognition,
32:416–426.
Smith, R. (last accessed March 2009). Open Dynamics En-
gine, Version 0.8. http://www.ode.org.
Treisman, A. M. and Gelade, G. (1980). A feature-
integration theory of attention. Congnitive Psychol-
ogy, 12:97–136.
Wolfe, J. M. and Horowitz, T. S. (2004). What attributes
guide the deployment of visual attention and how do
they do it? Nature Reviews, Neuroscience, 5:1–7.
ICINCO 2009 - 6th International Conference on Informatics in Control, Automation and Robotics
474