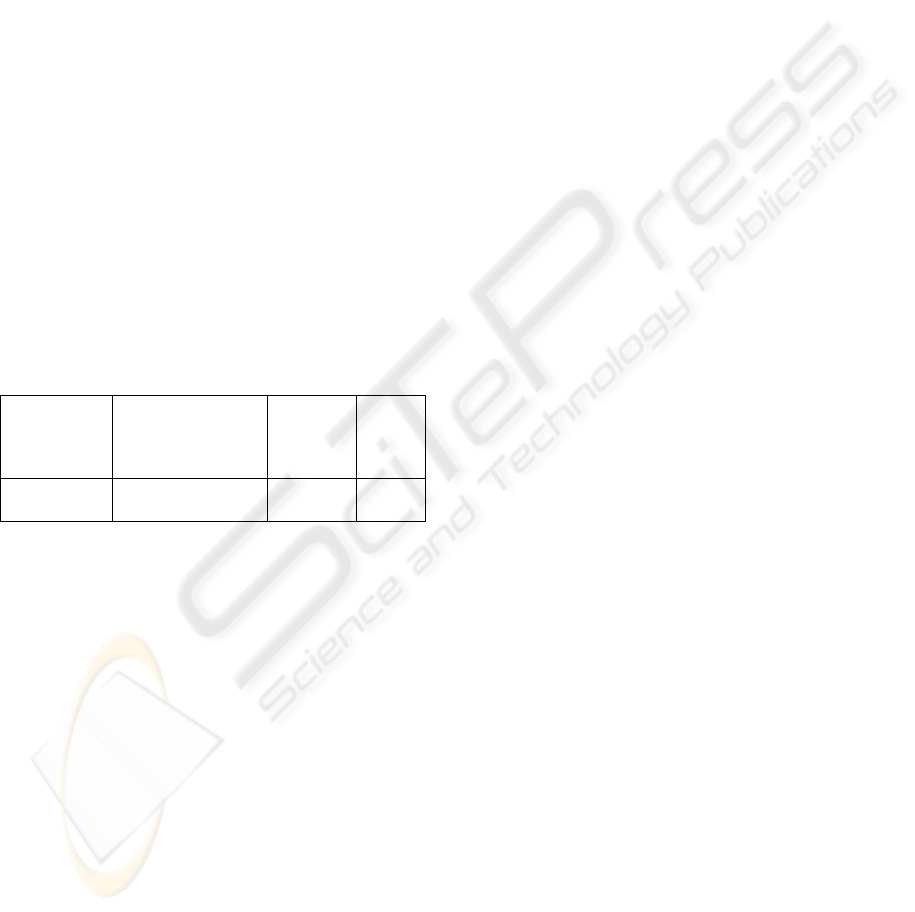
minimum number of four input features selected by
the non-overlap area distribution measurement
method (Lim and Gupta, 2004) and seven numbers
of dimensional data reduced by PCA. Stochastic %D,
Momentum, Disparity 5 days, and Disparity 10 days
that are used in Kim (Kim, 2003) are selected as the
minimum number of four input features with the
highest performance result; and are used to generate
the fuzzy rules for forecasting changes in the daily
KOSPI.
Even though the highest performance results using
the non-overlap area distribution measurement
method and PCA are the same result, there are two
merits in the non-overlap area distribution
measurement method. The first merit is that it takes
the less time to make input features because the
number of input features can be reduced. The second
merit is that the non-overlap area distribution
measurement method can realize real-time stocks
system. In case in PCA, if new data comes, new
dimensional data that are reduced by PCA are
changed, therefore PCA can’t realize real-time
stocks system.
Table 1: Comparisons of Performance Results for Kim
with NEWFM Using the Non-overlap Area Distribution
Measurement Method and PCA.
NEWFM
(Non-overlap area
distribution
measurement)
NEWFM
(PCA)
SVM
Performance
(%)
58.35 58.35 57.83
5 CONCLUSIONS
This paper proposes a new feature selection
methodology for financial forecasting using the non-
overlap area distribution measurement method based
on the neural network with weighted fuzzy
membership functions (NEWFM). This paper
compares the forecasting performance of the feature
extraction using PCA with the feature selection
using the non-overlap area measurement method.
NEWFM is a new model of neural networks to
improve forecasting performance results by using
self-adaptive weighted fuzzy membership functions.
The degree of classification intensity is obtained by
the bounded sum of weighted fuzzy membership
functions selected by NEWFM.
In this paper, the non-overlap area distribution
measurement method selects
the minimized
number
features by removing the worst input
features one by one. PCA is used for reducing twelve
numbers of initial input features to twelve numbers
of dimensions that consist of first principal
component to twelfth principal component. Four
minimum input features selected by the non-overlap
area distribution measurement method (Lim et al.,
2005) and seven numbers of dimensional data
reduced by PCA are presented to forecast the higher
or lower changes of the daily KOSPI. The
performance results of the non-overlap area
distribution measurement method and PCA are
58.35%.
Even though the highest performance results using
the non-overlap area distribution measurement
method and PCA are the same result, there are two
merits in the non-overlap area distribution
measurement method. The first merit is that it takes
the less time to make input features because the
number of input features can be reduced. The second
merit is that the non-overlap area distribution
measurement method can realize real-time stocks
system. In case in PCA, if new data comes, new
dimensional data that are reduced by PCA are
changed, therefore PCA can’t realize real-time
stocks system.
REFERENCES
T. D. Sanger, “Optimal Unsupervised Learning in a
Single-Layer Linear Feedforward Neural Network,”
Neural Networks, vol. 12, pp. 459-473, 1989.
Kyoung-jae Kim, “Financial time series forecasting using
support vector machines,” Neurocomputing 55, pp.
307-309, 2003
J. S. Lim, T-W Ryu, H-J Kim, and Sudhir Gupta, “Feature
Selection for Specific Antibody Deficiency Syndrome
by Neural Network with Weighted Fuzzy Membership
Functions,” FSKD 2005 (LNCS 3614), pp. 811-820,
Springer-Verlag, Aug. 2005.
H. Ishibuchi and T. Nakashima, “Voting in Fuzzy Rule-
Based Systems for Pattern Classification Problems,”
Fuzzy Sets and Systems, Vol.103, pp.223~238, 1999.
J. S. Lim and S. Gupta, “Feature Selection Using
Weighted Neuro-Fuzzy Membership Functions,” The
2004 International Conference on Artificial
Intelligence(IC-AI'04), June 21-24, 2004, Vol. 1, pp.
261-266, Las Vegas, Nevada, U.S.A.
Joon S. Lim, "Finding Features for Real-Time Premature
Ventricular Contraction Detection Using a Fuzzy
Neural Network System", IEEE Trans. on Neural
Networks, VOL. 20, No. 3, pp. 522-527, MARCH
2009.
ICSOFT 2009 - 4th International Conference on Software and Data Technologies
356