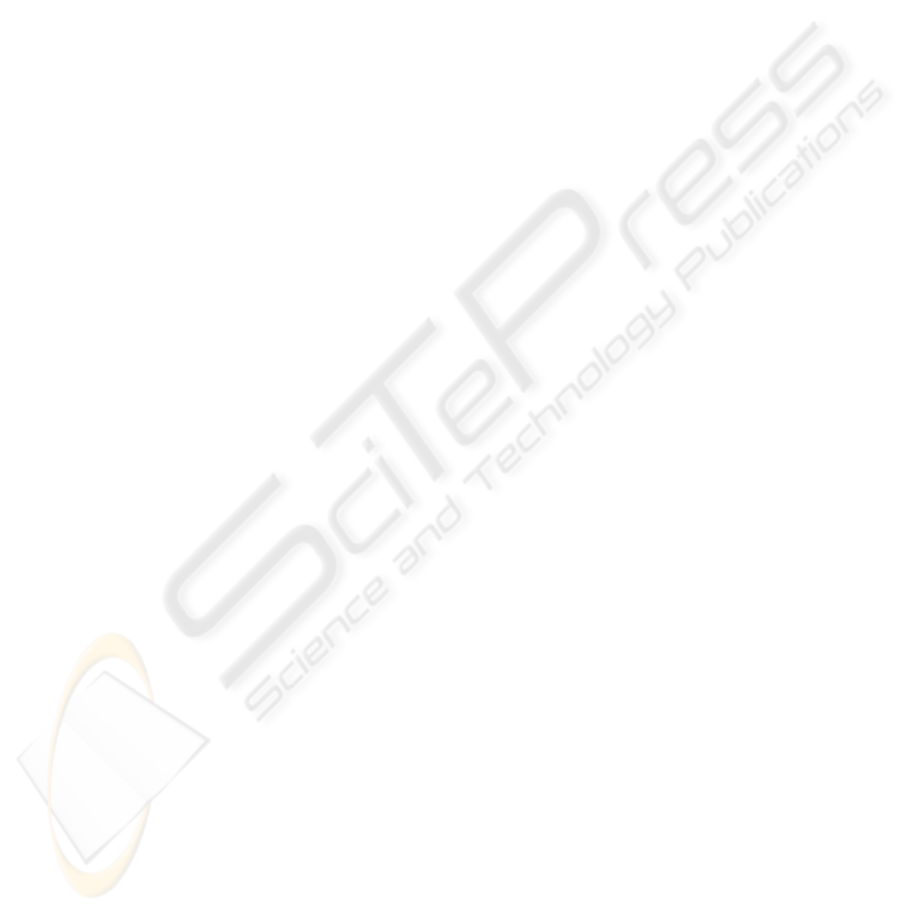
unknown attacks detection or to be adapted to an
evolutionary environment.
Machine learning approaches provide a potential
solution to adaptation and correctness problems in
intrusion detection context. Many classification
approaches try to construct an explicit function from
common set of features values to obtain instances
labels (category of attacks or normal).
Since (Denning D. E., 1987), several approaches
based on statistical learning were proposed for
intrusion detection. Among works which use TCP
packets analysis that of (Bykova, M. et al., 2001)
who have used simple statistics and (Ben Amor et
al., 2004) who have compared performances of
Bayesian networks and decision trees. (Valdes, A.,
Skinner, K., 2000) have used directly Bayesian
networks to model attacks and their temporal
evolution. Concerning Bayesian networks learning
for systems resources and users logs analyses we can
mention works of (Kruegel, C. et al, 2003, Scott, S.
L., 2004, John G., 1997).
The most adapted Bayesian classification model
for intrusion detection is naive Bayes. They present
several advantages due to their simple structure.
Bayesian naive networks construction is very
simple; it is always easy to consider new scenarios
(updates facility). Inference is polynomial, while
inference in Bayesian networks with general
structures is known to be a hard problem (Cooper,
G. F., 1990). However, naive Bayes networks
consider a very strong features independence
assumption: detection features are independent in a
session class context. Such hypothesis is not always
true in real applications.
This paper proposes an event classification
which uses TAN classifiers. This will enable us to
represent dependences between variables and to
integrate additional data, in order to improve
decision and detection process performances.
Section 2 shows how general expert information
can help in improving the detection rate of attacks,
while Section 3 presents comparative studies
between TAN and other classification approaches.
Finally, section 4 concludes the paper.
2 HANDLING EXPERT
INFORMATION
This section suggests a new procedure to deal with
this problem is to use additional information on
connections type. For example, we have information
that, on normal connections, usually there is X % of
these connections which are actually attacks and we
have to determine these connections.
In Bayesian networks, in order to determine
these connections, we need to sort classified
connections as normal according to probability that
they represent attacks (or according to another sort
function such difference between probability that
they represent attacks and probability that they are
normal), then the X % first connections will be taken
in order to be considered as attacks.
This information can be also related to several
attacks classes (Normal, Dos, R2L, U2R and
Probing in KDD’99 data set case), by making for
example assumption that on obtained normal
connections, there is X % of connections which are
actually DOS attacks and Y % which are R2L
attacks, thus it remains to determine normal
connections who represent these attacks. To do this
operation, we precede similarly, by sorting classified
connections as normal according to the probability
that they represent DOS attacks, then we take X %
first connexions.
The same thing for R2L attacks, but the sorting
function will be related to the probability that they
represent R2L attacks then the first Y % connections
will be taken.
The main remark drawn from the additional
information experiments results Table 1 is the
considerable PCC improvement, because this rate
have reached 96.69 % for five connections classes
case against 92 90 % without using additional
information and 97.40 % for two connections classes
case against 94.07 % without using additional
information.
As in (Ben Amor et al., 2004) we have used 10%
of KDD' 99 set (KDD cup 99, 1999), which
corresponds to 494019 training connections and
311029 test connections, with 18729 new attacks
which do not appear in training set.
Each connection is described by 41 discrete and
continuous features (for example connection
duration, protocol type, etc.) and marked to be
normal, or an attack, with only one attack type per
line (for example Smurf, Perl, etc.).
Attacks are grouped in four classes:
Denial of Service (DOS).
Make some machine
resources unavailable or too busy to answer to
legitimate users requests.
User to Root (U2R).
Exploit vulnerability on a
system to obtain a root access.
SECRYPT 2009 - International Conference on Security and Cryptography
62