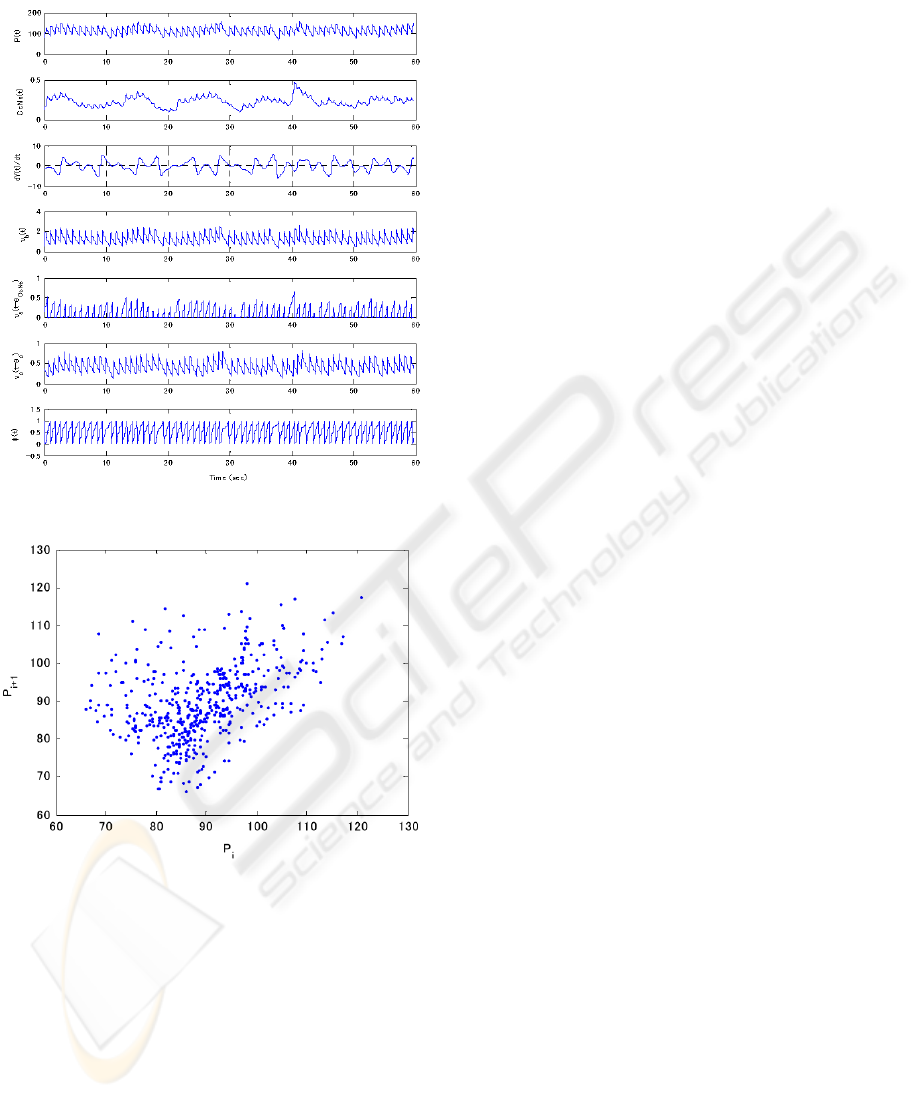
complexity and random-like patterns, characterizing
a chaotic behavior.
Figure 3: Output with influence of higher cerebral center.
Figure 4: Return maps for peak value of pressure P
i
.
Additionally the reconstructed orbits (called
attractor)
exhibited complex, non-periodic, diverse
orbiting patterns, strongly suggesting deterministic
chaotic behaviors. Lyapunov exponents of the
chaotic attractors were also calculated, as described
in next section, and showed positive values, again
being consistent with chaotic behaviors.
Higher cerebral center influence, therefore, was
found to play an important role and largely
responsible for emergence of chaos in finger
plethysmograms.
Furthermore, effects of chaoticity in higher
cerebral center were studied by simulation to
understanding how it affects the baroreflex
controlled cardiovascular system. Keeping coupling
coefficient γ =0.1 in constant, while changing
parameter B to 6.6, 7.0, and 7.5, we obtained a
changing chaoticity of higher center activity
described by Duffing equation (19), which was
characterized by changing Lyapunov exponents.
The largest Lyapunov exponents of (19) were
λ
1
=0.066, 0.105, 0.078, corresponding to three
parameters of B. Putting these parameters into the
model, we obtained time series of blood pressure p.
The largest Lyapunov exponent was then computed
using Sano and Sawada algorithm (Sano and
Sawada, 1985). Fig. 5 plotted these results, showing
a well linear relationship between the largest
Lyapunov exponents of higher cerebral center and
ones of blood pressure p.
This relation shown in Fig.5 explains
theoretically the causes for an increase in chaos of
finger plethysmogram come from higher Lyapunov
exponent in higher cerebral center. In other words,
there is higher information processing in central
nervous system, leading to increasing complexity of
finger plethysmograms.
6 CONCLUSIONS
Chaotic dynamics in finger plethysmogram system
was studied in relation to anesthesia processes. The
largest Lyapunov exponent of the plethysmograms
was found to be significant and can be used to
characterize the changed in mental/physical status
for the experimental processes. There were lower
values o f Lyapunov exponents, indicating a blocked
or depressed effect of anesthesia on central neural
system. We found there a further smaller values
estimated during the laparotomy and change O2 to
50%, showing the effect of cam down on mental
status. Whereas there was a highly Lyapunov
exponent in recovery consciousness from anesthesia,
even higher than the period of time before
preparation of the surgery.
To understand how the chaos arises and to explain
the changes in the Lyapunov exponent in finger
plethysmograms in experiments, a mathematical
model consisting of baroreflex feedback and
autonomous interactions was proposed and studied
numerically. By using of the model, the decrease of
the largest Lyapunov exponent in plethysmograms
was explained in relation to the decreased chaoticity,
and hence the depressed or blocked central nervous
ACT4SOC-EHST 2009 - 4th International Conference on Software and Data Technologies
404