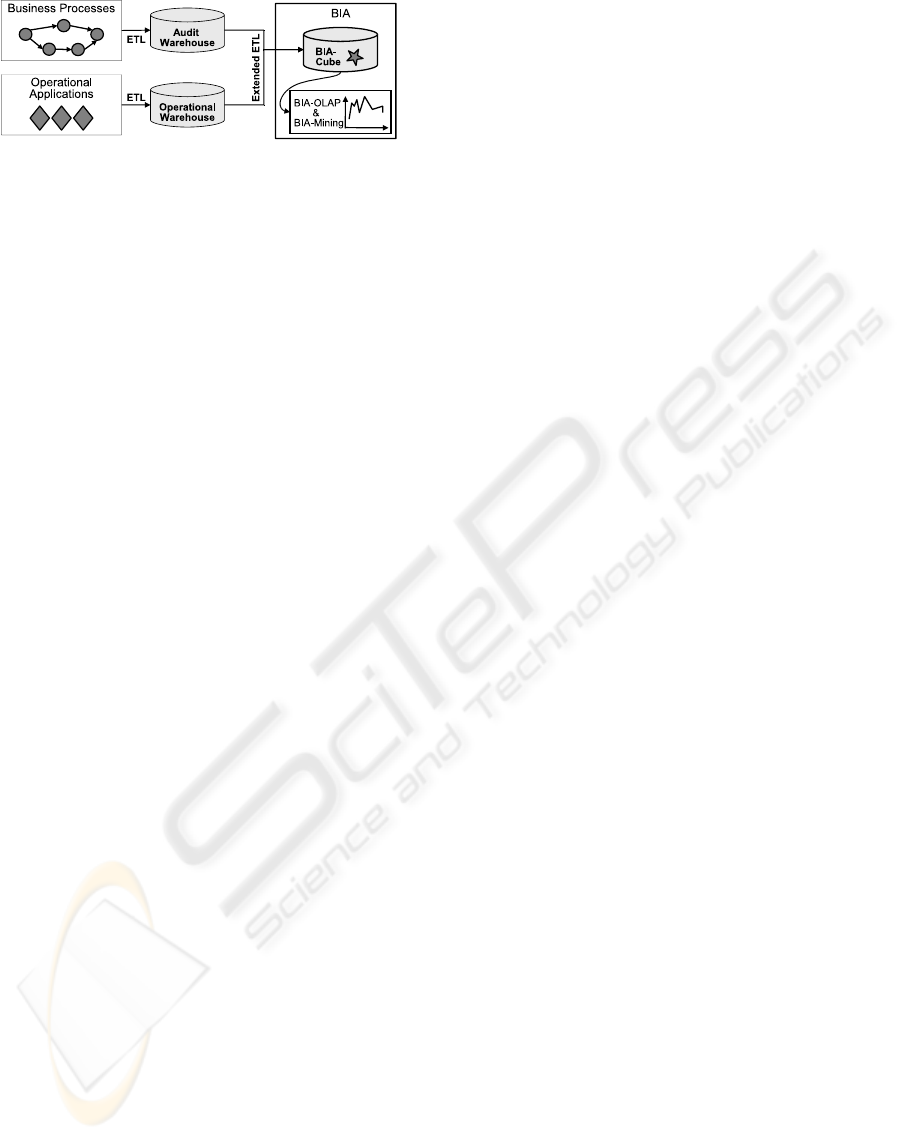
Figure 7: BIA Prototype.
enployees to guarantee a fast processing.
6 EXPERIENCE &
CONCLUSIONS
Fig.7 shows our prototype system that consists of
an integrated data warehouse system as well as ex-
tended BIA analysis and ETL facilities. These ex-
tended ETL facilities enable the integration of oper-
ational and process data based on their correlations
which are introduced in (Radesch¨utz et al., 2008).
For performing the extended analysis techniques
BIA-OLAP and BIA-Mining, we developed a BIA-
Cube Model that holds both operational data and pro-
cess data dimensions. We extended the usual OLAP
operators by a new function BIASUB in order to sup-
port the right query abstraction and efficiency. Early
experience shows the usefulness of the architecture
and the benefits obtained by the BIA approach. A
practical example that sketches the assets behind our
BIA approach is given in Section 4.4.
In our future work we will further explore the
adaption of data and process mining algorithms for
process optimization in order to develop concrete
BIA-OLAP and BIA-Mining algorithms for realizing
the analysis examples introduced in this paper.
REFERENCES
Agrawal, R., Gunopulos, D., and Leymann, F. (1998). Min-
ing process models from workflow logs. In Proc. of
Extending Database Technology, London, UK.
Agrawal, R. and Srikant, R. (1994). Fast algorithms for
mining association rules in large databases. In Proc.
of Very Large Data Bases, Chile.
Bruckner, R. M., List, B., and Schiefer, J. (2002). Striving
towards near real-time data integration for data ware-
houses. In Proc. of Data Warehousing and Knowledge
Discovery, France.
Casati, F., Castellanos, M., Dayal, U., and Salazar, N.
(2007). A generic solution for warehousing business
process data. In Proc. Very Large Data Bases, Austria.
Ceglar, A., Roddick, J. F., and Powers, D. M. W. (2007).
Curio: a fast outlier and outlier cluster detection algo-
rithm for large datasets. In Proc. of Integrating artifi-
cial intelligence and data mining, Australia.
Han, J. (2005). Data Mining: Concepts and Techniques.
Morgan Kaufmann Publishers Inc., CA, USA.
IBM (a). Infosphere Warehouse. Available: http://www-
01.ibm.com/software/data/infosphere/warehouse/.
ISO/IEC 9075-2 (2003). Information technology – database
languages – SQL – part 2: Foundation.
Jain, A. K., Murty, M. N., and Flynn, P. J. (1999). Data
clustering: a review. ACM Comput. Surv., 31(3):264–
323.
McCoy, D. (2002). Business activity monitoring: Calm be-
fore the storm. Technical Report LE15-9727, Gartner.
Michie, D., Spiegelhalter, D. J., Taylor, C. C., and Camp-
bell, J., editors (1994). Machine learning, neural and
statistical classification. Ellis Horwood, Upper Sad-
dle River, NJ, USA.
Microsoft (a). Analysis Services. Available:
http://www.microsoft.com/sql/technologies/analysis.
Oracle (a). Business Activity Monitoring. Available: http://
oracle.com/technology/products/integration/bam/.
Oracle (b). JDeveloper 11g. Available:
http://oracle.com/technology/software/products/jdev/.
Parsons, L., Haque, E., and Liu, H. (2004). Subspace clus-
tering for high dimensional data: a review. SIGKDD
Explor. Newsl., 6(1):90–105.
Radesch¨utz, S., Mitschang, B., and Leymann, F. (2008).
Matching of process data and operational data for a
deep business analysis. In Proc. of I-ESA, Germany.
Rubin, V., G¨unther, C. W., van der Aalst, W. M. P., Kindler,
E., van Dongen, B. F., and Sch¨afer, W. (2007). Process
mining framework for software processes. In Proc. of
International Conference on Software Process, USA.
Sayal, M., Casati, F., Dayal, U., and Shan, M.-C. (2002).
Business process cockpit. In Proc. of Very Large Data
Bases, China.
Uysal, I. and G¨uvenir, H. A. (1999). An overview of re-
gression techniques for knowledge discovery. Knowl.
Eng. Rev., 14(4):319–340.
Weerawarana, S., Curbera, F., Leymann, F., Storey, T., and
Ferguson, D. F. (2005). Web Services Platform Archi-
tecture. Prentice Hall PTR.
zur Muehlen, M. (2004). Workflow-based Process Con-
trolling. Foundation, Design, and Application of
workflow-driven Process Information Systems. Logos.
KMIS 2009 - International Conference on Knowledge Management and Information Sharing
82