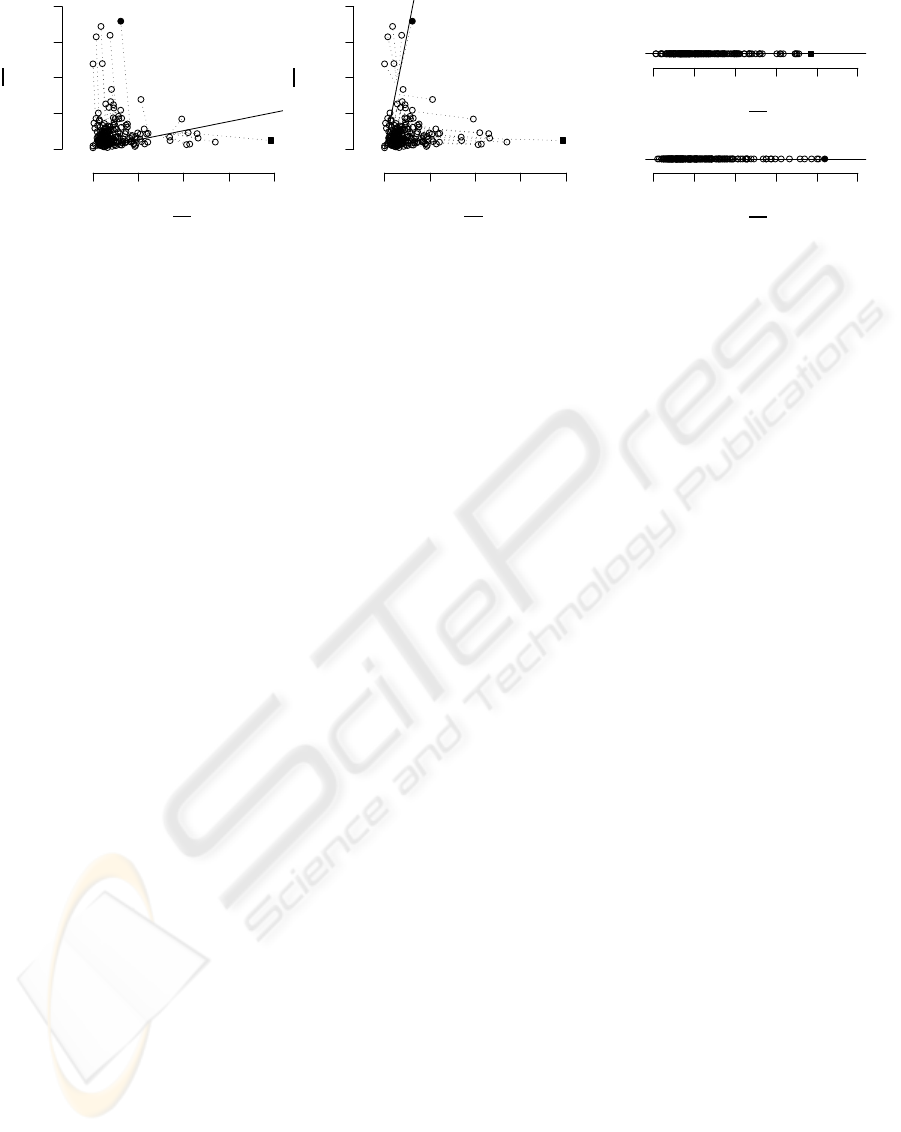
0.0 0.1 0.2 0.3 0.4
0.0 0.1 0.2 0.3 0.4
√
θ
1
√
θ
2
0.0 0.1 0.2 0.3 0.4
0.0 0.1 0.2 0.3 0.4
√
θ
1
√
θ
2
0.00 0.05 0.10 0.15 0.20 0.25
√
φ
n
0.00 0.05 0.10 0.15 0.20 0.25
√
φ
n
Figure 10: Preference maps based on the BookCrossing data set. The left figure shows a user preference of those who rely on
θ
1
, the middle one shows that of those who rely on θ
2
and the right figures show user preference levels φ
n
of these users.
given as lines, and user’s own preference levels are
given by projections onto the corresponding line. We
experimentally compared two types of projections,
linear and BT, and verified that there was no big dif-
ference in results of tau-b metric. As a criterion to vi-
sualize the K-dimensional preference map and a user
preference in a low dimensional space, we focus on
the user weight p(M
k
|x
n
). However, when we com-
pare two users, the low dimensional map which accu-
rately shows difference between their preferences is
expected, though it remains as a future work.
REFERENCES
Akaike, H. (1974). A new look at the statistical model iden-
tification. IEEE Trans. Automatic Control, 19(6):716–
723.
Barron, A., Rissanen, J., and Yu, B. (1998). The minimum
description length principle in coding and modeling.
IEEE Trans. Information Theory, 44(6):2743–2760.
Bennett, J. F. and Hays, W. L. (1960). Multidimensional
unfolding: Determining the dimensionality of ranked
preference data. Psychometrika, 25(1):27–43.
Bradley, R. A. and Terry, M. E. (1952). Rank analysis of in-
complete block designs: I. the method of paired com-
parisons. Biometrika, 39(3/4):324–345.
Croon, M. and Luijkx, R. (1993). Probability Models
and Statistical Analyses for Ranking Data, chapter 4,
pages 53–74. Lecture Notes in Statistics. Springer.
Davidson, R. R. and Beaver, R. J. (1977). On extending the
Bradley-Terry model to incorporate within-pair order
effects. Biometrics, 33(4):693–702.
Hastie, T. and Tibshirani, R. (1998). Classification by pair-
wise coupling. Annals of Statistics, 26(2):451–471.
Hastie, T., Tibshirani, R., and Friedman, J. (2009). The Ele-
ments of Statistical Learning: Data Mining, Inference
and Prediction. 2nd Ed. Springer Series in Statistics.
Springer.
Hayashi, C. (1993). Quantitative social research -belief sys-
tems, the way of thinking and sentiments of five na-
tions -. Behaviormetrika, 19(2):127–170.
Hino, H., Fujimoto, Y., and Murata, N. (2009). Item prefer-
ence parameters from grouped ranking observations.
In Proc. 13th Pacific-Asia Conference on KDD.
Huang, T.-K., Weng, R. C., and Lin, C.-J. (2006). General-
ized Bradley-Terry models and multi-class probabil-
ity estimates. Journal of Machine Learning Research,
7:85–115.
Joe, H. (1990). Extended use of paired comparison models,
with application to chess rankings. Applied Statistics,
39(1):85–93.
Kuk, A. Y. C. (1995). Modeling paired comparison data
with large numbers of draws and large variability of
draw percentages among players. The Statistician,
44(4):523–528.
Luce, R. D. (1959). Individual Choice Behavior. John Wi-
ley & Sons, Inc.
McLachlan, G. J. and Krishnan, T. (1996). The EM Algo-
rithm and Extensions. Wiley-Interscience.
Mei, G. and Shelton, C. R. (2006). Visualization of collab-
orative data. In Proc. 22nd International Conference
on UAI, pages 341–348. AUAI Press.
Murphy, T. B. and Martin, D. (2003). Mixtures of distance-
based models for ranking data. Computational Statis-
tics & Data Analysis, 41:645–655.
Plackett, R. L. (1975). The analysis of permutations. Ap-
plied Statistics, 24(2):193–202.
Rao, P. V. and Kupper, L. L. (1967). Ties in paired-
comparison experiments: A generalization of the
Bradley-Terry model. Journal of the American Sta-
tistical Association, 62(317):195–204.
Riedl, J. and Konstan, J. (2000). Movielens data set.
http://www.grouplens.org/.
Zenebe, A. and Norcio, A. F. (2007). Visualization of item
features, customer preference and associated uncer-
tainty using fuzzy sets. In Proc. Annual Meeting of
the North American Fuzzy Information Processing So-
ciety, pages 22–32.
Ziegler, C.-N., McNee, S. M., and Konstan, J. A. (2005).
Improving recommendation lists through topic diver-
sification. In Proc. 14th International World Wide Web
Conference (WWW ’05), pages 22–32.
ITEM-USER PREFERENCE MAPPING WITH MIXTURE MODELS - Data Visualization for Item Preference
111