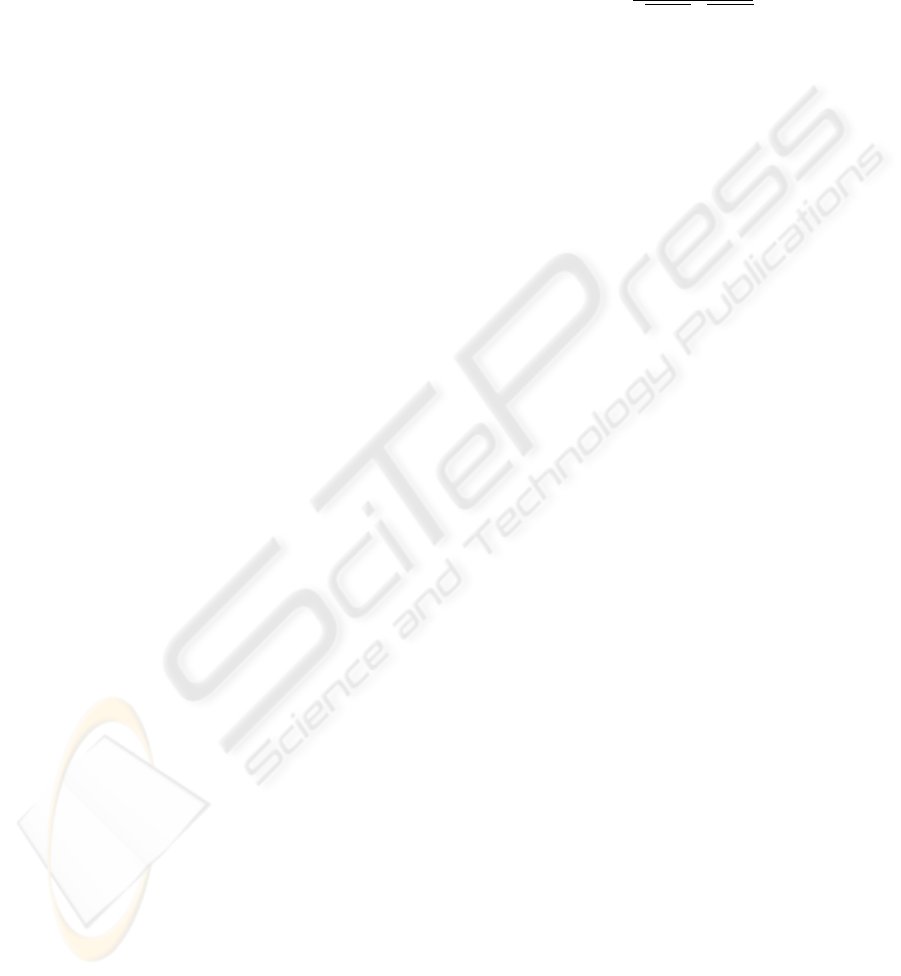
2.2 The Gender Regulation Profile
of Two Samples on a Roach
Microarray
Table 1 lists the genes which are selected to
represent the regulation profile between male and
female roach. Based on this, a gene regulation
profile reflecting the sample’s genders on a
microarray can be described by the measured log-
ratios at these gene spots. Therefore, for given a
roach microarray, the log-ratio values on
corresponding probes provide the information about
the genders of the roaches measured on this array.
2.3 Test Statistic and Test Method
Up to now, we have a gene regulation profile for
male vs female; we also can extract a gene
regulation profile for roaches measured on a
microarray. The task now is how to judge the
samples’ genders based on the two profiles. For
simplicity, the gene regulation profile built on the
720 days data will be referred as the reference
profile and the gene regulation profile extracted
from a target microarray will be called a query
profile.
Two statistical test methods are proposed and
applied. The first method is sign test which takes the
number of data points of same sign in a query profile
as test statistic. A positive value in a query profile
means this gene is up-regulated in cy5 sample
against cy3 sample; and the opposite is true for a
negative value. There are 20 genes in a profile, i.e.
20 log-ratio values, if two samples measured on a
microarray have same sex, the number of the data
points with positive (‘+’) sign and that with negative
sign (‘-‘) in the query profile are expected to be
equal. This can be taken as the null case and the
corresponding null distribution is formulated by a
binomial distribution function: b(20,0.5). Now to
take the number of negative signs in a query profile
as the test statistic, then if this statistic significantly
differs from 10, the profile can be judged to be
similar or opposite to reference profile. This can be
easily achieved by one side test of the statistic based
on b(20,0.5). If the profile is tested similar to
reference profile, then assigns male as the gender for
cy5 sample and female as the gender of cy3 sample,
vice versa. If the test is not significant in both sides,
the genders of cy3 sample and cy5 sample are same,
though we do not know that they are either male or
female.
The second method employs t-test of the
concordance between reference profile and query
profile. The concordance coefficient is defined to be
similar but not the same as Pearson’s Correlation
Coefficient. Denoting by P
r
and P
q
the reference
profile and query profile respectively and C(P
r
,P
q
)
the concordance coefficient of the two profiles is
formulated as:
,
(1)
Based on formula (1), the major difference
between concordance coefficient and Pearson’s
correlation coefficient is that: the mean of P
r
and
mean of P
q
impact on the value of concordance
coefficient, but they will to do nothing with the
value of Pearson’s correlation coefficient, because,
in the computation of Pearson’s correlation
coefficient, they are simply removed. Therefore, the
only case which allows the concordance coefficient
and Pearson’s correlation coefficient to have the
same value is that P
r
and P
q
have zero mean.
The use of the concordance instead of correlation
to assess the relationship between two gene
regulation profiles is vitally important, and for
obvious reasons. Because a value in a profile reflects
how a gene is regulated in contrast of cy5 sample
against cy3 sample: positive value for up-regulation
while negative value for down regulation. When
two profiles P
r
and P
q
are assessed, it should
guarantee a element to have positive contribution
when the element has the same sign in P
r
and P
q
, and
the opposite is true when the element has different
sign in P
r
and P
q
. This demand is satisfied in using
concordance coefficient. However, this might not
retain in the case when Pearson’s correlation
coefficient is used. For example, if the vector mean
for both P
r
and P
q
is 2, and the dispersion within
profiles are normal noises of small values, that is
2
,
|
|
1 and
2
,
1.
Due to each gene in query profile is about equally
regulated as corresponding gene in reference profile,
the two gene regulation profiles should be judged as
closely the same. However, Pearson’s correlation
coefficient of the two profiles will be around 0,
because
,
,
in this case. The
judgement based on Pearson’s correlation coefficient
will consequently be: the two profiles are nothing in
common, which is wrong and definitely
unreasonable. In contrast, from formula (1) the
concordance coefficient of the two profiles will be
,
1. Consequently, to conclude that the
two profiles are almost identical is reasonable and
correct.
KDIR 2009 - International Conference on Knowledge Discovery and Information Retrieval
266