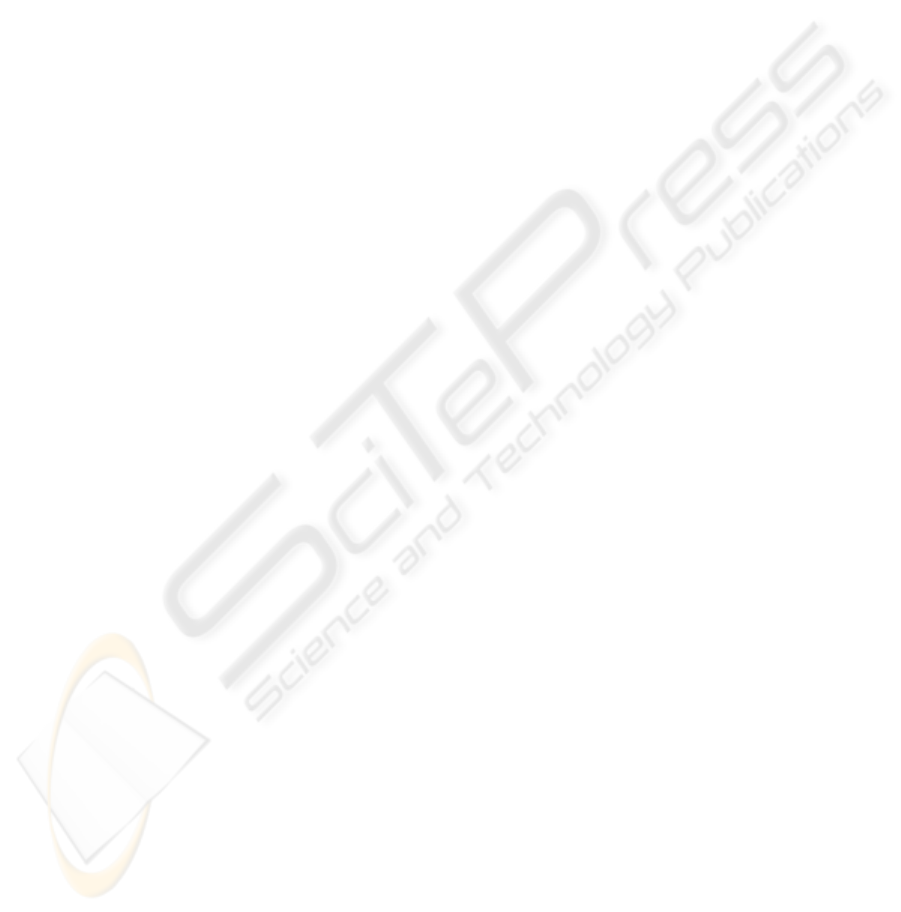
region may contracts or expands. On the other hand,
varying the cutoff in the wavelet approach, the area
correspondent to the heart region practically does
not change. In this sense, the fuzzy model is
dependent of the cutoff value, which clearly is not
desirable in an automatic process. Other fact of great
importance in an automatic process is that the fuzzy
model is dependent of the PEEP settings, i.e., for
each PEEP its parameters need to be changed. This
limitation does not have in the wavelet model; its
configuration is not dependent on PEEP value.
Comparing the heart maps generated from wavelet
and fuzzy models with the image of the cardiac
region obtained by the saline method, we elaborated
ROC curves for each PEEP value. Table 2 presents
these results. We can note that both models
presented excellent agreements with the saline
injection. ROC curves of fuzzy and wavelet models
were practically equivalent. In general, the best
results were found with the wavelet algorithm 2.
Due to the movement of the heart in the
longitudinal axis and to the fact that the lungs should
be more closed in the ZEEP condition, it is expected
that the identification of the pixels as belonging or
not to each anatomical structure becomes more
difficult in this situation. In this sense, we expected
that the agreement would be larger for PEEP18,
followed by smaller values of agreement for
PEEP12 and ZEEP, respectively. However, this
monotonic behavior was not observed. The possible
hypothesis for this fact is that the ventricle was
dislocated to a more central region due to the heart
movement.
4 CONCLUSIONS
The wavelet methodology proposed was able to
identify the heart region from EIT data of perfusion
when compared with the saline method and fuzzy
model. Both methodologies present limitations to be
overcome. In the wavelet approach, we should have
extended our analysis to other cardiac chambers
beside the ventricular region. In the fuzzy model, the
limitations consist in the cutoff and PEEP
dependences in the generation of the heart maps.
These limitations should be understood in a more
deep work.
Finally, the results presented here were
encouraging and indicate that the modeling of EIT
images pos data acquisition, besides the
reconstruction algorithm, is a good way that should
be certainly explored
REFERENCES
Tanaka, H., Ortega, N. R. S., Galizia, M. S., Borges, J. B.,
& Amato, M. B. P., (2008). Fuzzy modeling of
electrical impedance tomography images of the lungs,
Clinics, 2008, vol.63, no.3, p.363-370. ISSN 1807-
5932.
Polikar R. (1999). The Engineer's Ultimate Guide to
Wavelet Analysis: The Wavelet Tutorial, 1999.
Retrieved March, 2009 from Web site
http://engineering.rowan.edu/~polikar/WAVELETS/
WTtutorial.html.
Adler, A., Amyot, R., Guardo, R., Bates, J.
H. T. &
Berthiaume, Y., (1997). Monitoring
changes in lung
air and liquid volumes with electrical impedance
tomography. J. Appl. Phys. 83: 1762-1767
Brown, B.H. (2003). Electrical impedance tomography
(EIT): a review, Journal of Medical Engineering &
Technology, 2003, vol. 27, n
o
3, pp. 97-108.
Noor, J.A. E. (2007). September) Electrical Impedance
Tomograph at low frequencies [Thesis of Doctor],
Faculty of Science at University of New South Wales.
Retrieved October 2008 from
http://unsworks.unsw.edu.au/vital/access/services/Do
wnload/unsworks:1359/SOURCE02?view=true.
Lima, R. G. , Aya, J. C. C. , Moura, F. S. , Schweder, R.
K. , Mirandola, L. A. S. & Pai, C. N. , (2005). A
black-box back-projection algorithm for electrical
impedance tomography, in Proceedings of the 18th
International Congress of Mechanical Engineering,
Ouro Preto, Minas Gerais, Brazil.
IDENTIFYING THE CARDIAC REGION IN IMAGES OF ELECTRICAL IMPEDANCE TOMOGRAPHY THROUGH
WAVELET TRANSFORM
383