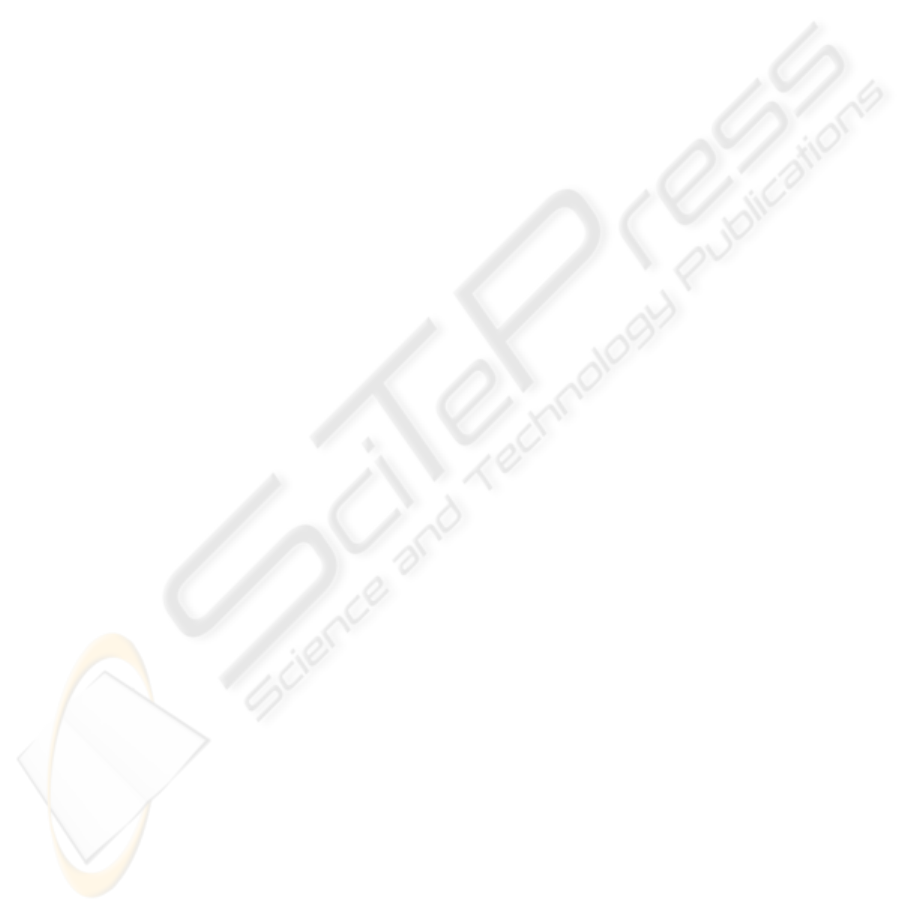
6 CONCLUSIONS
In the paper, we introduce the notion of an
assortment, suggest an auxiliary MOP whose
solution may aid decision makers in choosing an
optimal assortment. Furthermore, an EMO to solve
the auxiliary MOP is suggested. The paper
contributions are: a. A new kind of a set (the
assortment) is represented and motivated, b. A need
to choose a set based on a set of sets has been
encountered here for the first time by using EC, c.
New motivation to correlate nature and sociology to
engineering design has been suggested d. A new
motivation for variability within engineering design
has been highlighted, e. Yet another use of MOEA's
has been explored.
Future work should consider searching for
assortments based on the auxiliary MOP directly
from the beginning without relying on an
a priori
search of the PL. Furthermore, some more examples
and test cases should be explored. Among the
investigated cases, problems with more objectives,
both in the original and the auxiliary MOPs should
be interesting. Finally, robustness, while choosing an
assortment, should be an important issue. Gaining
more robustness may call for a need for overlapping
of RSFs, which may reduce variability.
REFERENCES
Avigad G., Eisenstadt, E., and Goldvard A., Report no
Br.11709, at http://mech.braude.ac.il/gideonavigad/
Avigad, G., Moshaiov, A., Set-based Concept Selection in
Multi-objective Problems: Optimality versus
Variability Approach, Journal of Engineering Design,
Vol. 20(3) pp: 217 – 242, 2009
Bensaid, N. and Matheieu, P. An Autonomous Agent
System to Simulatea Set of Robots Exploring a
Labyrinth, In the proceedings of 11th International
FLAIRS Conference, pp: 384-388, Sanibel, Florida
May, 17-20, 1998.
Boer, P., Szyszko, K., and Vermeulen, R. Spreading the
risk of extinction by genetic diversity in populations of
the carabid beetle, Netherlands Journal of Zoology, 43,
242-259, 1993.
Booy, G. et al., Genetic Diversity and the Survival of
Populations. J. Planet Biology 2, 379-395, 2000.
Deb, K., Pratap, A., Agarwal, S., and Meyarivan, T. A
Fast and elitist multiobjective genetic algorithm:
NSGA–II, IEEE Transactions on Evolutionary
Computation, 6(2):182–197, April 2002.
Deb K., Multi-objective optimization using evolutionary
algorithms. J. Wiley & Sons, Ltd, 2001.
Goldberg, D.E., Genetic algorithms in search,
optimization and machine learning, Addison-Wesley,
1989.
Marler, R.T., and Arora, J.S. Survey of multi-objective
optimization methods for engineering, Structural
Multidisciplinary Optimization, 26: 369–395, 2004.
Mattson, C. A., and Messac, A. Pareto frontier Based
concept selection under uncertainty, with
visualization, Optimization and Engineering, 6: 85–
115, 2005.
Mayr E. 1982. The growth of biological thought: diversity,
evolution and inheritance. Harvard, Cambs. p567 et
seq.
Pareto, V. 1906: Manuale di Economica Politica, Societa
Editrice Libraria. Milan; translated into English by
A.S. Schwier as Manual of Political Economy, edited
by A.S. Schwier and A.N. Page, 1971. New York:
A.M. Kelley
Van Veldhuizen, D.A., and Lamont, G.B. Multiobjective
evolutionary algorithms: analyzing the State-of-the-
Art, Evolutionary Computation, 8(2): 125-147, 2000.
Zitzler, E., Thiele, L.: Multiobjective Optimization Using
Evolutionary Algorithms—A Comparative Study. In
Eiben, A.E., ed.: Parallel Problem Solving from
Nature V, Amsterdam, Springer-Verlag 292–301,
1998.
Zitzler, E., L. Thiele, M. Laumanns, C. M. Fonseca, and
V. G. da Fonseca (2003). Performance assessment of
multiobjective optimizers: An analysis and review.
IEEE Transactions on Evolutionary Computation 7(2),
117–132, 2003.
Zitzler, E., and K¨unzli, S. Indicator-Based Selection in
Multiobjective Search, Proceedings of the 8th
International Conference on Parallel Problem Solving
from Nature (PPSN VIII) September 2004,
Birmingham, UK
IJCCI 2009 - International Joint Conference on Computational Intelligence
276