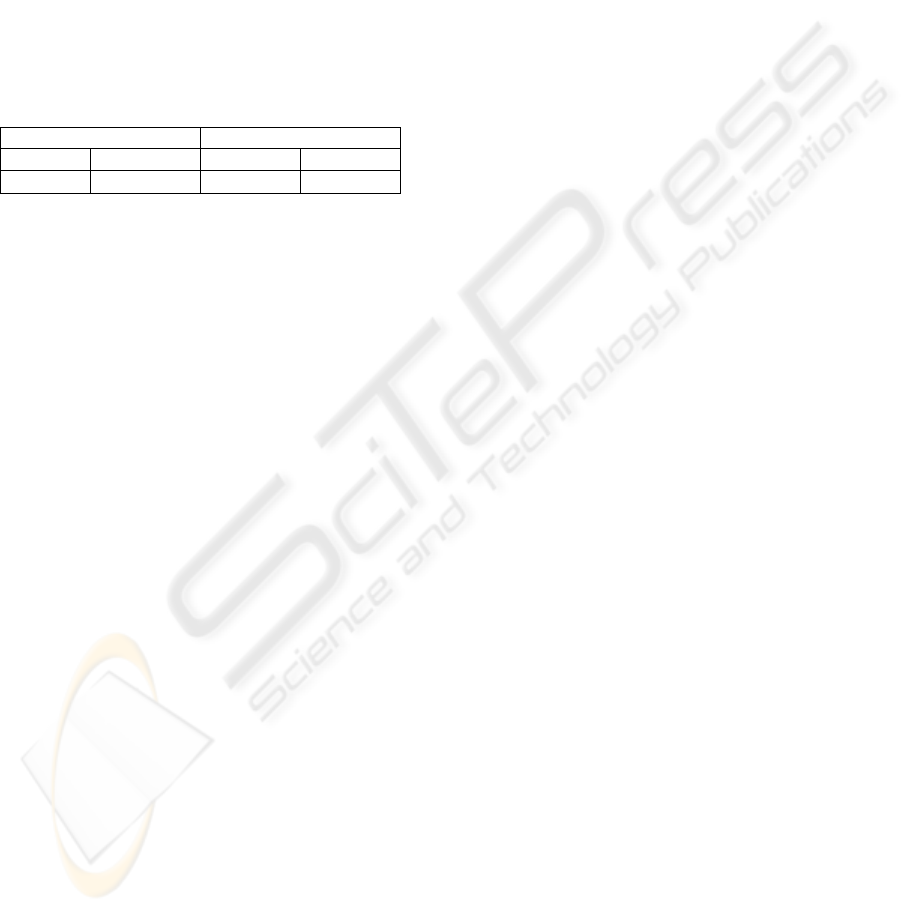
changes in CO
2
, the test shows that the SVMs are
significantly better than the ANNs (p=0.0004).
The reactivity curves for both types of models
show an acceptable physiological response, with
those obtained from training with changes in 5%
CO
2
always better.
The average results of the standard reactivity test
of the 16 subjects was 4.05±1.38%/mmHg,
(average±SD).
Entering a normalized step response between [0-
1] into the EtCO
2
input it is possible to measure each
subject’s reactivity. The average values of each
model are shown in Table 3.
Table 3: Reactivity of the models (%/mmHg).
SVM ANN
Baseline 5% CO
2
Baseline 5% CO
2
4.32 ±4.2 4.44 ±1.9 2.22 ±3.0 3.13
1.4
When conducting a hypothesis test between the
standard reactivity test and the reactivities obtained
by the models, it is seen that there are no differences
with the reactivities extracted from the SVMs in
both cases. Compared to ANNs, the test is
significantly different in the baseline case (p=0.002)
and has values very close to the limit for CO
2
changes (p=0.07)
4 CONCLUSIONS
The results not only show the superiority of SVMs
in terms of precision and calculation of reactivity,
but it is also seen that they show a smaller variance,
particularly in the case of CO
2
changes.
The baseline mean square error of the SVM
model is 3%, which is much better than the 20%
reached in the work of Mitsis et al. (2004).
We believe that both the global optimization and
the slack-variable properties of SVMs are
responsible for the better results in comparison with
the Artificial Neural Networks.
The main future challenges involve new studies
in the field of biomedical signals that may allow the
evaluation of the other properties of the SVM, such
as the ability to represent time varying phenomena.
ACKNOWLEDGEMENTS
This work was supported by a research grant from
FONDECYT, Government of Chile, Project
1070070.
REFERENCES
Acir, N., and Guzelis, C., 2004, Automatic spike detection
in EEG by a two-stage procedure based on support
vector machines. Comput Biol Med. Vol. 7, 561-75.
Demuth, H., Beale. M., 2001, Neural network toolbox
user’s guide, The MathWorks Inc.
Espinoza, M., Suykens, J.K.A., Belmans, R., and De
Moor, B., 2007, Electric load forecasting - using
kernel based modeling for nonlinear system
identification,” IEEE Control Systems Magazine
(Special Issue on Applications of System
Identification), 43-57.
Martínez-Ramón, M., Rojo-Álvarez, JL., Camps-Valls, G.
Navia-Vázquez, A., Soria-Olivas, E., and Figueiras-
Vidal, A., 2006, Support vector machines for
nonlinear kernel ARMA system identification, IEEE
Trans. on Neural Net., Vol. 17, 1617-1622.
Mitsis, G., Poulin, M., Robbins, P., Marmarelis, V., 2004,
Nonlinear modeling of dinamic effects of arterial
pressure and CO
2
variations on cerebral blood flow in
healthy humans, IEEE Trans. Biomed. Eng., Vol. 51,
1932-1943.
Panerai, R., Simpson, D., Deverson, S., Mahony, P.,
Hayes, P., Evans, D., 2000, Multivariate Dynamic
Analysis of Cerebral Blood Flow Regulation in
Humans”, IEEE Trans. Biomed. Eng., Vol 47, 419-
423.
Rojo-Álvarez, JL, Martínez-Ramón, M. Prado-Cumplido,
M Artés-Rodríguez, Figueiras-Vidal, A., 2004,
Support Vector Method for Robust ARMA System
Identification, IEEE Tran. Signal Process. Vol. 52,
155-164.
Simpson, D., Panerai, R., Evans, D., Garnham, J., Naylor,
A., Bell, P., 2000, Estimating normal and pathological
dynamic responses in cerebral blood flow velocity to
step changes in end-tidal pCO
2
, Med. Biol. Eng.
Comp., Vol. 38, 535-539.
Schölkopf, B., Smola, A., Williamson R.C., and Bartlett,
P.L., 1998, New support vector algorithms, Neural
Computation, Vol. 12, 1083-1121,.
Suykens, JAK., Vandewalle J., 2000, Recurrent least
squares support vector machines, IEEE Trans. on
Circuits and Systems I, Vol. 47, 1109–1114.
Widder, B., Paulat, K., Hackspacher, J. Mayr, E. 1986,
Trascranial Doppler CO
2
test for the detection of
hemodynamically critical carotid artery stenoses and
occlusions, Eur. Arch. Psych. Neurol. Sci., Vol 236,
162-168.
COMPARISON BETWEEN SVM AND ANN FOR MODELING THE CEREBRAL AUTOREGULATION BLOOD
FLOW SYSTEM
525