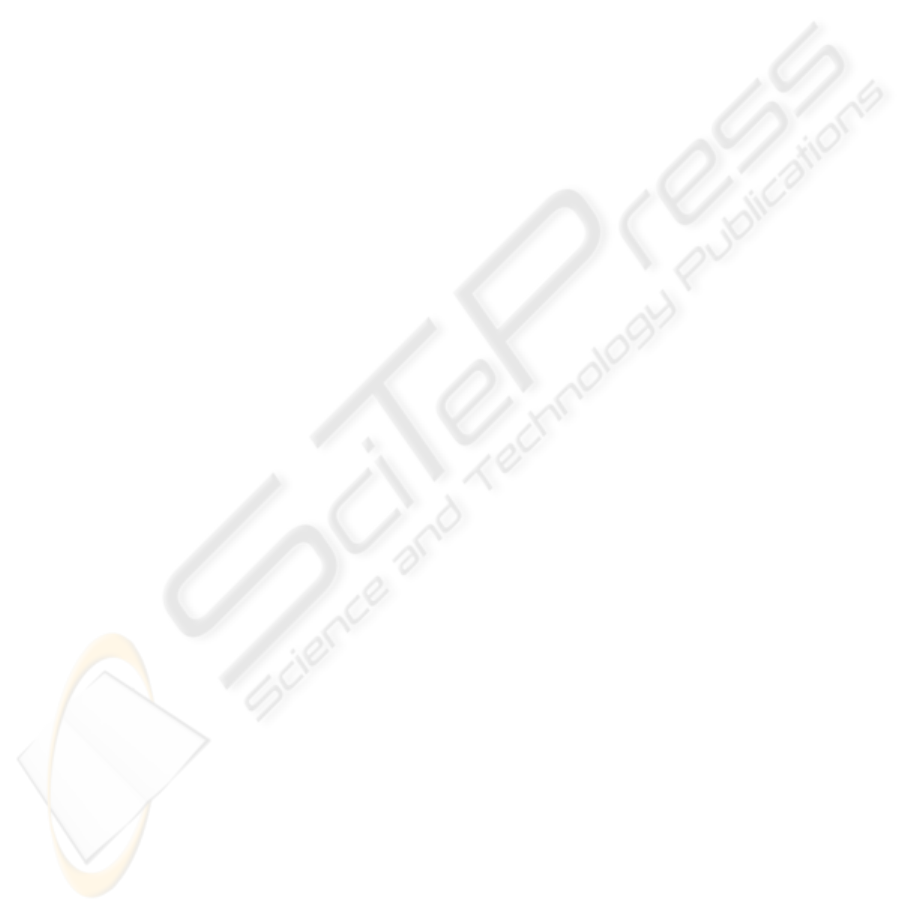
without expert skills for parallel and distributed sys-
tems. MapReduce programs are automatically paral-
lelized and executed on a large cluster of machines.
The run-time system takes care of the details of par-
titioning the input data, scheduling the program’s ex-
ecution across a set of machines, handling machine
failures, and managing the required inter-machine
communication.
3.4 Hadoop Streaming
Hadoop, open source software written in Java, is a
software framework implementing MapReduce pro-
gramming model(Hadoop, ). While we write map-
per and reducer functions in Java by default in this
Hadoop framework, Hadoop distribution contains a
utility, Hadoop streaming. The utility allows us to
create and run Map/Reduce jobs with any executable
or script as the mapper and/or the reducer. The util-
ity will create a Map/Reduce job, submit the job to an
appropriate cluster, and monitor the progress of the
job until it completes. When an executable is spec-
ified for mappers, each mapper task will launch the
executable as a separate process when the mapper is
initialized. When an executable is specified for reduc-
ers, each reducer task will launch the executable as a
separate process then the reducer is initialized.
This Hadoop streaming is useful in invoking in-
terpreter of VDM languages and executing functional
programs with MapReduce framework.
4 PRELIMINARY EVALUATION
We consider the impact of Cloud with MapReduce
framework on leveraging light-weight formal meth-
ods. We focus on the productivity in preparing the
platform and the scalability of the platform in increas-
ing its size.
4.1 Platform
According to (Armbrust et al., 2009), Cloud Comput-
ing is the sum of SaaS and Utility Computing, but
does not normally include Private Clouds, which is
the term to refer to internal datacenters of a business
or other organization that are not made available to
the public. However, we use a private cloud comput-
ing platform, which is a small version of IBM Blue
Cloud, for our preliminary evaluation. Figure 2 shows
the outline of our cloud. In our Cloud platform, we
can dynamically add or delete servers which com-
pose the Cloud if the machines are x86 architecture
and able to run Xen. We can add a new server as
the resource of Cloud by automatically installing and
setting up Domain 0 (Dom0) through network boot.
When a user requests a computing platform from the
Web page of Cloud portal, the user can specify the vir-
tual OS image (Domain U (DomU) of Xen in our plat-
form) and applications from registered ones as well as
the number of virtual CPUs (VCPUs), the amount of
memory and storage within the resource of Cloud. In
our Cloud, the number of VCPUs is limited within
the number of physical CPUs to guarantee the perfor-
mance of DomU. When the request is admitted, the
requested computing platform is automatically pro-
vided.
Our Cloud supports an automatic set up of Hadoop
programming environment in provisioning requested
platforms. We need the following steps to set up
Hadoop environment:
1. Installing base machines into nodes
2. Installing Java
3. Mapping IP address and hostname of each ma-
chine
4. Permitting non-password login from the master
machine to all the slave machines
5. Configuring Hadoop on the master machine
6. Copying the configured Hadoop environment to
all slave machines from the master machine
Setting up Hadoop Platform (Step 2 - 6). Our
Cloud is able to perform the step 2, 3, 5, and 6 au-
tomatically to set up the Hadoop environment by se-
lecting Hadoop as the application in provisioning the
platform.
Addition of Base Machine. For the step 1, we only
have to set the machine network bootable in the BIOS
configuration when adding the machine to our Cloud.
4.2 Scalability in Cloud Platform
We evaluate the scalability of our approach when in-
creasing the number of the slave machine nodes of in
running Hadoop streaming on our Cloud. We mea-
sure the elapsed time of simple program with the data
of 1GB and 5GB. We change the number of the slave
machines from 2 to 9. All the slave machines have
1GB of memory and 20GB of storage. Users cannot
control the allocation of the master and the slave ma-
chines on the physical machines in our Cloud.
We show the result in Figure 3. As we see in Fig-
ure 3, the increase of the number of nodes does not
always reduce the elapsed time in both data size. For
example, the elapsed time increases when we increase
the number of DomUs from 2 to 3 with the data of
1GB and from 4 to 5 with the data of 5GB.
LEVERAGING LIGHT-WEIGHT FORMAL METHODS WITH FUNCTIONAL PROGRAMMING APPROACH ON
CLOUD
267