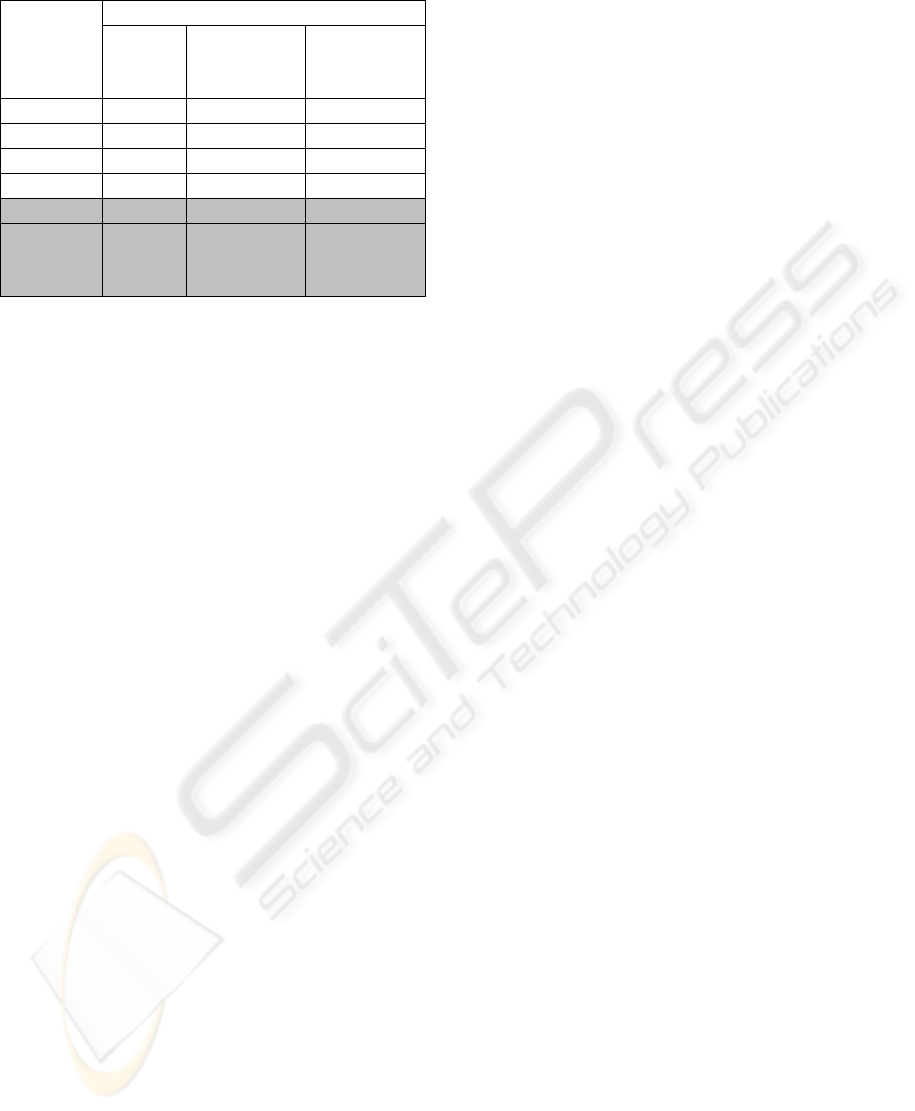
Table 1: Result of Implementing Proposed Method.
The
Number Of
Classes
The Correct of Percentage Classification (%)
KNN
Classifier
SVM
Classifier(Kernel
1)
SVM
Classifier(Kernel
2)
20 96.6 100 100
40 88.3 94.3 96.3
60 90.8 91.6 95.6
80 89.3 90.1 95.8
100(GLCM) 88.5 90.07 94.2
100(GLCM
(Combining
Sub bands)
87.5 91.3 96.3
4 CONCLUSIONS
In this paper we proposed an effective algorithm for
iris feature extraction using contourlet transform Co-
occurrence Matrix have been presented. The GLCM
proved to be a good technique as it provides
reasonable accuracy and is invariant to iris rotation.
For Segmentation and normalization we use
Daugman methods .Feature extraction in our
proposed method includes: sub bands proper
composition from Contuorlet pyramid and co-
occurrence calculations and finally selecting a set of
Haralick‘s properties that form the Maximum
distance between inter classes and Minimum
distance between intra classes. Our proposed method
can classify iris feature vector properly. The rate of
expected classification for the fairly large number of
experimental date in this paper verifies this claim. In
the other words our method provides a less feature
vector length with an insignificant reduction of the
percentage of correct classification.
REFERENCES
Bamberger, R.H., and Smith M. J. T, 1992. A filter bank
for the directional decomposition of images: Theory
and design, IEEE Trans. Signal Proc., vol. 40, no. 4,
pp. 882–893.
Boles, W.W., and Boashash B,1998.A Human
Identification Technique Using Images of the Iris and
Wavelet Transform, IEEE Trans. Signal Processing,
Vol.46, No.4, 1998, pp.1185-1188.
Burt P. J., and Adelson E. H., 1983. The Laplacian
pyramid as a compact image code, IEEE Trans.
Commun., vol. 31, no. 4, pp. 532–540.
Daugman, J., 1993. High confidence visual recognition of
persons by a test of statistical independence, IEEE
Transactions on Pattern Analysis and Machine
Intelligence, Vol. 15, No. 11, pp: 1148-1160.
Daugman, J., 2004. How Iris Recognition works.IEEE
Transactions on Circuits and systems for video
Technology, Vol.14, No.1, pp: 21-30.
Do M. N., and Vetterli, M, 2004. The contourlet
transform: an Efficient directional multi-resolution
image representation, IEEE Transactions on Image
Processing, vol. 14, issue 12, pp. 2091-2106.
Haralick, R.M, et al., 1973.Textural features for image
classification, IEEE Transactions on Systems, Man,
and Cybernetics, vol.3, no.6, and pp.610-621.
Karen P. Hollingsworth, Kevin W. Bowyer, 2008. The
Best Bits in an Iris Code, IEEE Transactions on
Pattern Analysis and Machine Intelligence (PAMI).
Ma, L, et al. 2003. Personal identification based on iris
texture analysis, IEEE Trans. Patt. Analy. & Mach.
Intel vol. 25, No. 12, pp. 1519-1533.
Sanchez-Avila, C. et al.,2002.Iris-based biometric
recognition using dyadic wavelet transform,IEEE
Aerospace and Electronics Systems Magazine ,
Volume: 17 Issue 10, pp. 3-6.
Wildes, R. P, 1996, et al., A machine-vision system for iris
recognition, Machine Vision and Applications,
Springer-Vela.
Zhenan Sun et al., 2005.Improving Iris Recognition
Accuracy via Cascaded Classifier, IEEE Transactions
on systems, Man and Cybernetics-part C: Applications
reviews, Vol.35, No.3.
CASIA, 2003.Chinese Academy of Sciences – Institute of
Automation, Database of 756 Grayscale Eye Images.
http://www.sinobiometrics.com Version 1.0.
Jafar M. H. Ali, Aboul Ella Hussanien,2003.An Iris
Recognition System to Enhance E-security
Environment Based on Wavelet Theory, AMO -
Advanced Modeling and Optimization, Volume 5,
Number 2.
Ma, L, et al., 2002. Iris Recognition Based on
Multichannel Gabor Filtering, Proc. Fifth Asian Conf.
Computer Vision, vol. I, pp. 279-283.
Zhu, Y. et al., 2000. Biometric Personal Identification
Based on Iris Patterns, Proc. Int’l Conf. Pattern
Recognition, vol. II,pp. 805-808.
A NOVEL TECHNIQUE FOR IRIS RECOGNITION SYSTEM
533