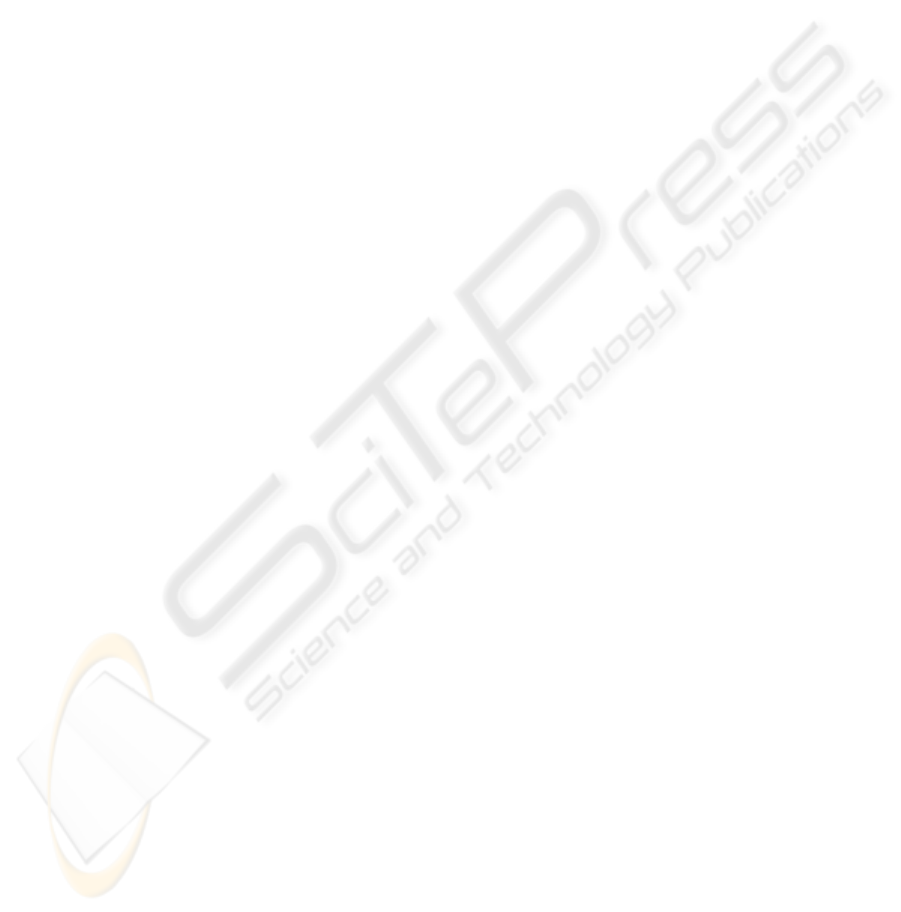
6 CONCLUSIONS
We have presented here a new activation function
for a multi-valued neuron. This l- multiple activation
function makes it possible to learn nonlinearly
separable problems and non-threshold multiple-
valued functions using a single multi-valued neuron.
This significantly increases the MVN’s functionality
and makes the MVN more efficient in applications.
The learning algorithm for the MVN with the l-
multiple activation function was also presented.
ACKNOWLEDGEMENTS
The author appreciates the assistance of the UC
Irvine machine Learning Repository (Asuncion and
Newman, 2007), from where two data sets were
downloaded for the simulation purposes.
REFERENCES
Aizenberg, N.N and Aizenberg, I.N. (1992). CNN Based
on Multi-Valued Neuron as a Model of Associative
Memory for Gray-Scale Images, In Proceedings of the
Second IEEE Int. Workshop on Cellular Neural
Networks and their Applications, Technical University
Munich, 36-41
Aizenberg, N.N. Ivaskiv, Yu. L., and Pospelov, D.A.
(1971). About one Generalization of the Threshold
Function Doklady Akademii Nauk SSSR (The Reports
of the Academy of Sciences of the USSR), 196, 1287-
1290, (in Russian).
Aizenberg, N.N. and Ivaskiv, Yu.L. (1977). Multiple-
Valued Threshold Logic. Naukova Dumka Publisher
House, Kiev (in Russian).
Aizenberg. I.N. (1991). The Universal Logical Element
over the Field of the Complex Numbers, Kibernetika
(Cybernetics and Systems Analysis) 27,, 116-121 (in
Russian, journal is translated into English by
Consultants Bureau, An Imprint of Springer Verlag
New York LLC, Vol. 27, 467-473).
Aizenberg, I., Aizenberg, N., and Vandewalle, J. (2000).
Multi-valued and universal binary neurons: theory,
learning, applications. Kluwer Academic Publishers,
Boston Dordrecht London.
Aizenberg, I. and Butakoff, C. (2002). Image Processing
Using Cellular Neural Networks Based on Multi-
Valued and Universal Binary Neurons, Journal of
VLSI Signal Processing Systems for Signal, Image and
Video Technology, 32, 169-188.
Aizenberg, I., Moraga, C., and Paliy D. (2005). A
Feedforward Neural Network based on Multi-Valued
Neurons, In Computational Intelligence, Theory and
Applications. Advances in Soft Computing, XIV, (B.
Reusch - Ed.), Springer, Berlin, Heidelberg, New
York, 599-612.
Aizenberg, I. and Moraga, C. (2007). Multilayer
Feedforward Neural Network Based on Multi-Valued
Neurons (MLMVN) and a Backpropagation Learning
Algorithm", Soft Computing, 11, 169-183.
Aizenberg, I., Paliy, D. V., Zurada, J.M., and Astola J. T.
(2008). Blur Identification by Multilayer Neural
Network based on Multi-Valued Neurons, IEEE
Transactions on Neural Networks, 19, 883-898.
Aizenberg, I. (2008). Solving the XOR and Parity n
Problems Using a Single Universal Binary Neuron,
Soft Computing, 12, 215-222.
Asuncion, A. and Newman, D.J. (2007). UCI Machine
Learning Repository
[http://www.ics.uci.edu/~mlearn/MLRepository.html].
Irvine, CA: University of California, School of
Information and Computer Science.
Aoki, H. and Kosugi, Y. (2000). An Image Storage
System Using Complex-Valued Associative Memory,
In Proceedings. of the 15
th
International Conference
on Pattern Recognition. 2, 626-629.
Aoki, H., Watanabe, E., Nagata, A., and Kosugi Y. (2001).
Rotation-Invariant Image Association for Endoscopic
Positional Identification Using Complex-Valued
Associative Memories. In Bio-inspired Applications of
Connectionism, Lecture Notes in Computer Science
(Mira J., Prieto A. -eds) Springer, Berlin Heidelberg
New York, 2085, 369-374.
Chen, J.-H and Chen, C.-S. (2002). Fuzzy Kernel
Perceptron. IEEE Transactions on Neural Networks
13, 1364-1373.
Jankowski, S., Lozowski, A., and Zurada J.M. (1996).
Complex-Valued Multistate Neural Associative
Memory. IEEE Tranactions. on Neural Networks 7,
1491-1496.
Lee, D.L. (2001). Improving the Capacity of Complex-
Valued Neural Networks with a Modified Gradient
Descent Learning Rule IEEE Transactions on Neural
Networks 12, 439–443.
Lee, D.L. (2004). "Complex-valued Neural Associative
Memories: Learning Algorithm and Network
Stability", in book "Complex-Valued Neural
Networks: Theories and Applications" (Hirose A.–
Ed.), World Scientific, 29-56.
Muezzinoglu, M. K., Guzelis, C., and Zurada J. M, (2003).
A New Design Method for the Complex-Valued
Multistate Hopfield Associative Memory, IEEE
Transactions on Neural Networks 14, 891-899.
IJCCI 2009 - International Joint Conference on Computational Intelligence
354