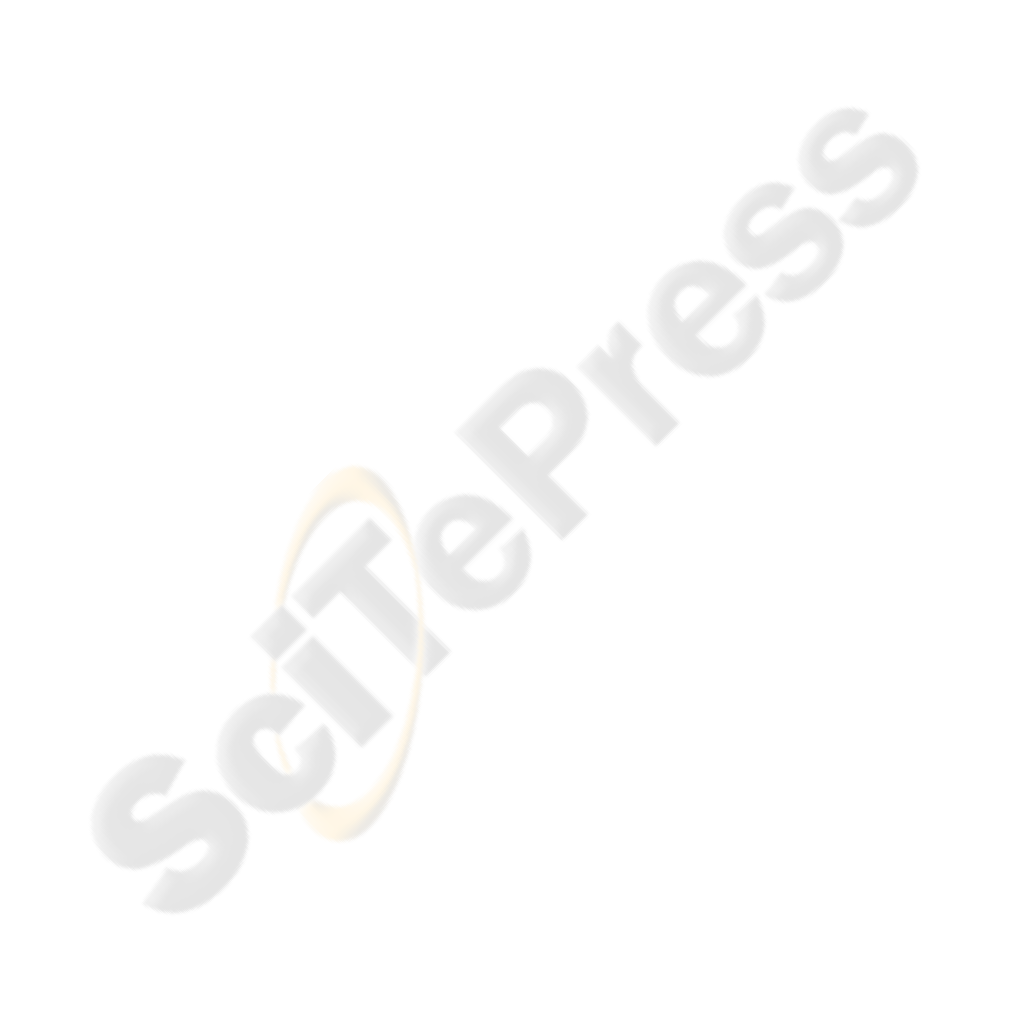
ontology users, skills of OEs, available economical
resources, etc.).
The proposed method is supported by detailed
usage procedures, relies on modellers’ knowledge
(by means of with DEs and KEs involved in the
building process), and specifies quantitative and
qualitative measurements. The former measures
assure more objectivity whereas the latter involve
matters of perception (i.e., human judgements based
on the experience of OEs and ontology users).
The proposed evaluation method grounds on a
benchmarking process, it is based on the quality of
results (defect detection), and it also considers how
to improve them (defect correction). This allows
future improvements of the methodology.
The idea presented provides a ready-on-hand
procedure for ontology developers to assess different
methodologies. As future work, we intend to adopt
the BSCs-based method to evaluate other OBMs and
to compare them. The benchmarking results will
support ontology engineers in selecting the most
appropriate OBM for a particular application.
REFERENCES
Boehm, B.M., Abts, C., Chulani, S. (2000). Software
development cost estimation approaches–A survey. In
Annals of Software Engineering, 10, 177-205.
Buglione, L., Abran, A., Meli, R. (2001). How functional
size measurement supports the balanced scorecard
framework for ICT. In Proceedings of European
Conference on Software Measurement and ICT
Control, 259-272.
Burton-Jones, A., Storey, V.C., Sugumaran, V.,
Ahluwalia, P. (2005). A semiotic metrics suite for
assessing the quality of ontologies. In Data &
Knowledge Engineering, 55(1), 84-102.
Chimienti, M., Dassisti, M., De Nicola, A., Missikoff, M.
(2006) Benchmarking Criteria to Evaluate Ontology
Building Methodologies. In Proceedings of the EMOI-
INTEROP’06 Luxembourg.
Corcho, O., Fernández-López, M., Gomez-Perez, A.
(2003). Methodologies, tools and languages for
building ontologies. Where is the meeting point? In
Data & Knowledge Engineering, 46(1), 41–64.
Dam, K.H., Winikoff, M. (2004). Comparing Agent-
Oriented Methodologies. In Agent-Oriented
Information Systems (AOIS 2003), Springer LNAI
3030, 78–93.
De Nicola, A., Navigli, R., Missikoff, M. (2009). A
software engineering approach to ontology building.
In Information Systems, 34 (2), 258-275.
Fernández-López, M. (1999). Overview of Methodologies
for Building Ontologies. In Proceedings of the IJCAI-
99 Stockholm, Sweden.
Geerts, G.L., McCarthy, W.E. (2000). The Ontological
Foundations of REA Enterprise. Information Systems.
Annual Meeting of the American Accounting
Association, Philadelphia, PA, USA.
Gruber, T.R. (1993). A translation approach to portable
ontology specifications. In Knowledge Acquisition,
5(2), 199–220.
Grüninger, M., Fox, M.S. (1995). Methodology for the
design and evaluation of ontologies. In Proceedings of
the Workshop on Basic Ontological Issues in
Knowledge Sharing.
Guarino, N., Welty, C. (2002). Evaluating ontological
decisions with Ontoclean. In Communications of the
ACM, 45 (2), 61–65.
Haarslev, V., Möller R. (2001). Description of the
RACER system and its applications. In Proceedings of
the International Workshop on Description Logics.
Hakkarainen, S., Strasunskas, D., Hella, L., Tuxen, S.
(2005). Choosing Appropriate Method Guidelines for
Web-Ontology Building. In ER 2005. Lecture Notes in
Computer Science, 3716, 270-287.
Holsapple, C.W., Joshi, K.D. (2002). A collaborative
approach to ontology design. In Communications of
the ACM, 45(2), 42-47.
Ibáñez, M. (1998). Balanced IT Scorecard Generic Model
Version 1.0. In Technical Report of European
Software Institute, ESI-1998-TR-009.
Ide, N., Véronis, J. (1993). Extracting knowledge bases
from machine-readable dictionaries: have we wasted
our time? In Proceedings of KB&KS workshop,
Tokyo, 257–266.
IEEE (1996). IEEE Std. 1074-1995 Standard for
Developing Software Life Cycle Processes. Standards
Coordinating Committee of the IEEE Computer
Society, April 26, 1996, New York, USA.
Kaplan, R., Norton, D. (1996). The balanced scorecard:
translating strategy into action. Harvard Business
School Press, Boston Massachusettes.
Likert, R. (1932). A technique for the measurement of
attitudes. In Archives of Psychology, 140, 1–55.
Noy, N.F., McGuinness, D.L. (2001). Ontology
Development 101: a guide to creating your first
ontology, Knowledge Systems Laboratory, Stanford.
Paslaru Bontas Simperl, E., Tempich, C., Sure Y. (2006).
ONTOCOM: a cost estimation model for ontology
engineering. In Proceedings of ISWC.
Sure, Y., Studer, R. (2002). On-To-Knowledge
Methodology. On-To-Knowledge deliverable D-17,
Institute AIFB, University of Karlsruhe.
Swartout, B., Ramesh, P., Knight, K., Russ, T. (1997).
Toward Distributed Use of Large-Scale Ontologies. In
Proceedings of the Symposium on Ontological
Engineering of AAAI, Stanford, California, 138-148.
Tempich, C., Pinto, H.S., Staab, S. (2006). Ontology
engineering revisited: an iterative case study. In
Proceedings of the European Semantic Web
Conference ESWC, Springer LNCS 4011, 110–124.
KEOD 2009 - International Conference on Knowledge Engineering and Ontology Development
146