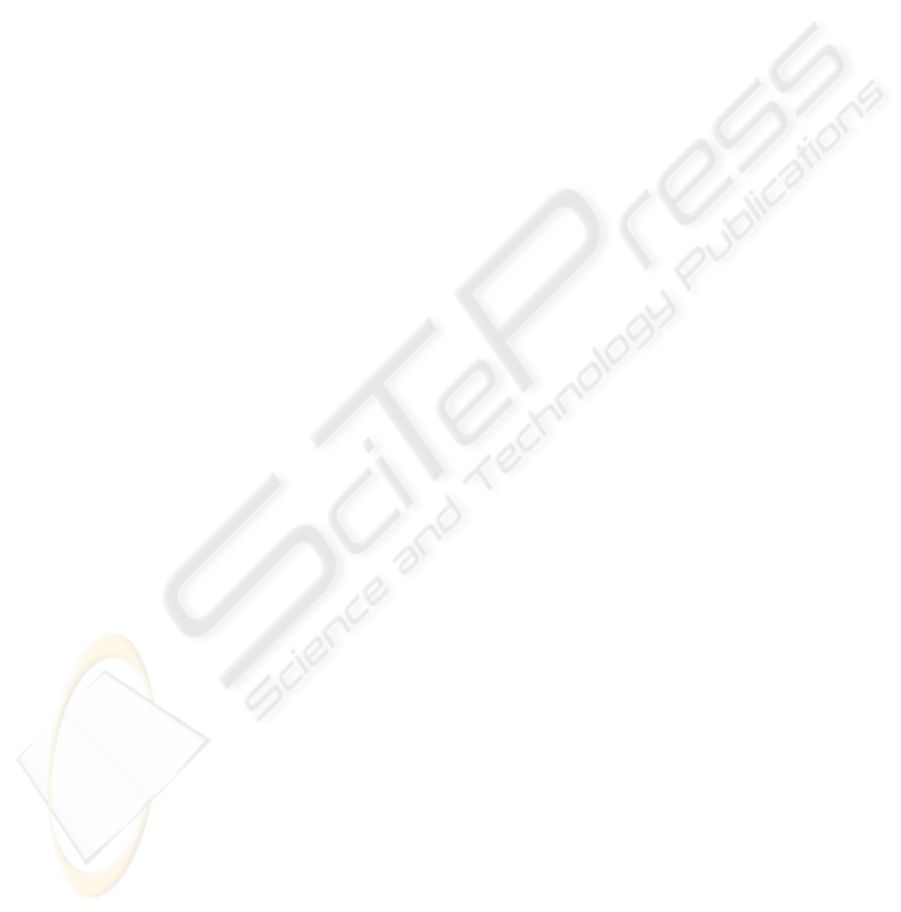
8 CONCLUSIONS
In this paper we presented an approach, which yielded
outstanding results for lexical knowledge acquisition.
Even though the lexico-syntactic patterns performed
competitively with the first test runs of the statistical
approach, some adjustments could outperform them.
The first run utilized a semi-automatically created
training data set containing 300.000 tokens, which
resulted in F
1
≈ .78 . It appeared that a fractional
amount of the data (around 4%), which was manually
corrected, outperformed those results with F
1
≈ .89.
A suffix length=5 and an adjustment of the λ val-
ues for linear interpolation only gave slight improve-
ments on the third position of the decimal point. How-
ever, for the bootstrapping appoach these minimal im-
provements became apparent and resulted in an F
1
value of over .91.
Future Work involves the deployment of other sta-
tistical models to the given data. One choice is to ap-
ply conditional random fields (Lafferty et al., 2001).
Here, the evaluation of the performance of Hidden
Markov Models and other statistical models on an un-
seen domain is an important step towards generaliza-
tion. The transfer of the model (initially adjusted to
persons) to e.g. general processes or things will not
be a challenge as the first sentences in Wikipedia are
mostly identically structured.
ACKNOWLEDGEMENTS
This research was only possible with the financial
support of the Klaus Tschira Foundation and the
CONTENTUS Use Case of the THESEUS Program
funded by the German Federal Ministry of Economics
and Technology (BMWi).
REFERENCES
Abney, S. (2002). Bootstrapping. In Proceedings of the
40th Annual Meeting of the Association for Compu-
tational Linguistics, pages 360–367, Morristown, NJ,
USA. Association for Computational Linguistics.
Amsler, R. A. (1981). A taxonomy for english nouns
and verbs. In Proceedings of the 19th Annual Meet-
ing on Association for Computational Linguistics,
pages 133–138, Morristown, NJ, USA. Association
for Computational Linguistics.
Brants, T. (2000). TnT – A statistical Part-of-Speech tag-
ger. In Proceedings of the Sixth Applied Natural
Language Processing (ANLP-2000), pages 224–231,
Seattle, Washington.
Choi, S. and Park, H. R. (2005). Finding taxonomical rela-
tion from an mrd for thesaurus extension. In Dale, R.,
Wong, K.-F., Su, J., and Kwong, O. Y., editors, Nat-
ural Language Processing - IJCNLP, volume 3651 of
Lecture Notes in Computer Science, pages 357–365.
Springer.
Etzioni, O., Cafarella, M., Downey, D., Popescu, A.-M.,
Shaked, T., Soderland, S., Weld, D. S., and Yates,
A. (2005). Unsupervised named-entity extraction
from the web: an experimental study. Artif. Intell.,
165(1):91–134.
Hearst, M. A. (1992). Automatic acquisition of hyponyms
from large text corpora. In Proceedings of COLING,
Nantes, France.
Kazama, J. and Torisawa, K. (2007). Exploiting wikipedia
as external knowledge for named entity recognition.
In Joint Conference on Empirical Methods in Natu-
ral Language Processing and Computational Natural
Language Learning, pages 698–707.
Lafferty, J., McCallum, A., and Pereira, F. (2001). Con-
ditional random fields: Probabilistic models for seg-
menting and labeling sequence data. In ICML-01.
Rabiner, L. R. (1989). A tutorial on hidden markov models
and selected applications in speech recognition. Pro-
ceedings of the IEEE, 77.
Snow, R., Jurafsky, D., and Ng, A. Y. (2005). Learning
syntactic patterns for automatic hypernym discovery.
In Saul, L. K., Weiss, Y., and Bottou, L., editors, Ad-
vances in Neural Information Processing Systems 17,
pages 1297–1304. MIT Press, Cambridge, MA.
Tufis, D. and Mason, O. (1998). Tagging romanian texts:
a case study for qtag, a language independent prob-
abilistic tagger. In Proceedings of the 1st Interna-
tional Conference of Language Resources and Eval-
uation (LREC-98), Granada, Spain.
Van Rijsbergen, C. J. K. (1979). Information Retrieval,
2nd edition. Dept. of Computer Science, University
of Glasgow.
TRIGRAMS'N'TAGS FOR LEXICAL KNOWLEDGE ACQUISITION
25