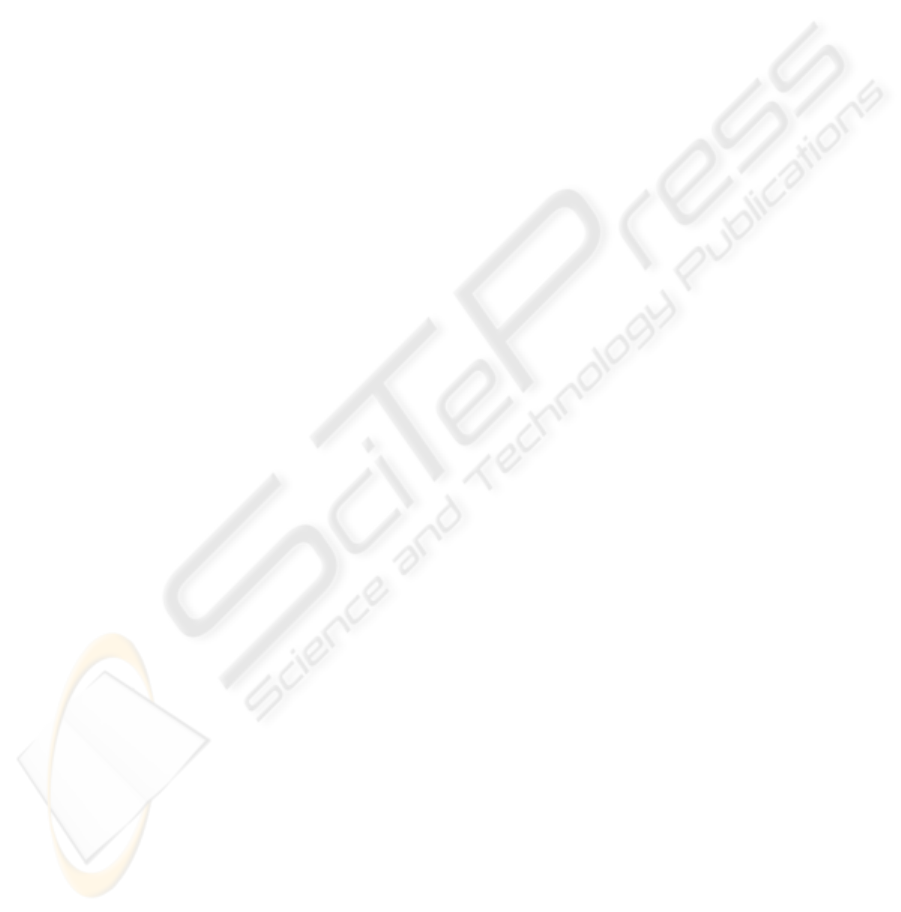
confirms that our approach was able to correctly
identify the subset of measurements that is of
importance, which needs to be incorporated into
current practices to streamline clothing design.
5 CONCLUSIONS
One of the biggest challenges for the apparel
industry is to produce garments that fit the
customers properly and are aesthetically pleasing.
Better characterizations of our populations are thus
needed. Furthermore, the different sizes must
correspond to real body shapes, i.e. one or more
archetypes should represent the individuals
belonging to the same size accurately. In the context
of tailoring, however, the optimal scenario is to
cover the largest number of people with the fewest
number of sizes. Here, it is preferred to have only
one archetype, since each new size increases the
complexity in the manufacturing.
Our approach satisfies the aforementioned
requirements, since we were able to group the
individuals into clusters with a well-defined
Centroid. Our verification, when using the Cleopatra
system, indicates that the cluster membership
corresponds to the reality. Our results show that the
number of body measurements may be significantly
reduced by applying interestingness measure-based
feature selection and feature extraction. Moreover,
these new sets of reduced body measurements
improve the predictive accuracy. These sets contain
the most important body measurements for defining
the body sizes, and may be used in garment design
to identify those body measurements that require
special attention, when tailoring clothes for a
specific population and gender.
REFERENCES
Aldrich, W. (2001). Pattern Cutting for Women's Tailored
Jackets: Classic and Contemporary, Wiley-Blackwell
Publishing, Oxford.
Ashdown. S., Loker, S. and Rucker, M. (2007). Improved
Apparel Sizing: Fit and Anthropometric 3-D Scan
Data, Annual Report NTC Project: S04-CR01-07,
National Textile Center.
Cunningham, P. (2007). Dimension Reduction, Technical
Report UCD-CSI-2007-7, University College Dublin,
1–24.
DesMarteau K. (2000). CAD: Let the Fit Revolution
Begin, Bobbin, 42, 42-56.
Geng, L. And Hamilton, H. J. (2006). Interestingness
Measures for Data Mining: A Survey, ACM Comput.
Surv., 38 (3), 1-32.
Han, J. and Kamber, M. (2006). Data Mining: Concepts
and Techniques, Morgan Kaufmann, San Francisco.
Hsu, C.-H., Lin, H.-F. and Wang, M.-J. (2007).
Developing Female Size Charts for Facilitating
Garment Production by Using Data Mining, Journal of
Chinese Institute of Industrial Engineers, 24 (3), 245–
251.
Kim, Y. Street, W. N. and Menczer, F. (2003). Feature
Selection in Data Mining, Data mining: Opportunities
and Challenges, IGI Publishers, USA, 80–105.
McGarry, K. (2005). A Survey of Interestingness
Measures for Knowledge Discovery, Knowl. Eng.
Rev., 20 (1), 39–61.
Paquet, E., Robinette, K. M., and Rioux, M. (2000).
Management of three-dimensional and anthropometric
databases: Alexandria and Cleopatra. Journal of
Electronic Imaging, 9, 421–431.
Robinette, K. M., Blackwell, S., Daanen, H., Fleming, S.,
Boehmer, M., Brill, T., Hoeferlin, D., and Burnsides,
D. (2002). Civilian American and European Surface
Anthropometry Resource (CAESAR), Final Report,
Volume I: Summary. AFRL-HE-WP-TR-2002-0169,
United States Air Force Research Laboratory, Human
Effectiveness Directorate, Crew System Interface
Division, 2255 H Street, Wright-Patterson AFB OH
45433-7022.
Schofield, N. A. and LaBat, K. L. (2005). Exploring the
Relationships of Grading, Sizing and Anthropometric
Data, Clothing and Textiles Research Journal, 23 (1),
13–27.
SizeUSA (2009). The US National Size Survey,
http://www.sizeusa.com/.
SizeChina (2009). China National Sizing Survey,
http://www.sizechina.com/.
A Suit that Fits (2009), http://www.asuitthatfits.com.
Veitch, D., Veitch, L. and Henneberg, M. (2007). Sizing
for the Clothing Industry Using Principal Component
Analysis - An Australian Example, Journal of ASTM
International (JAI), 4 (3), 12 pp.
Viktor, H. L., Paquet, E., and Guo, H. (2006). Measuring
to fit: Virtual tailoring through cluster analysis and
classification. In PKDD 2006: Knowledge Discovery
in Databases, 395–406.
Witten, I. H. and Frank, E. (2005). Data Mining: Practical
Machine Learning Tools and Techniques, Morgan
Kaufmann, San Francisco.
Zadrozny, B., Weiss, G. and Saar-Tsechansky, M. (2006).
UBDM 2006: Utility-Based Data Mining 2006
Workshop Report, SIGKDD Explor. Newsl., 8(2), 98–
102.
EXPLORATIVE DATA MINING FOR THE SIZING OF POPULATION GROUPS
159