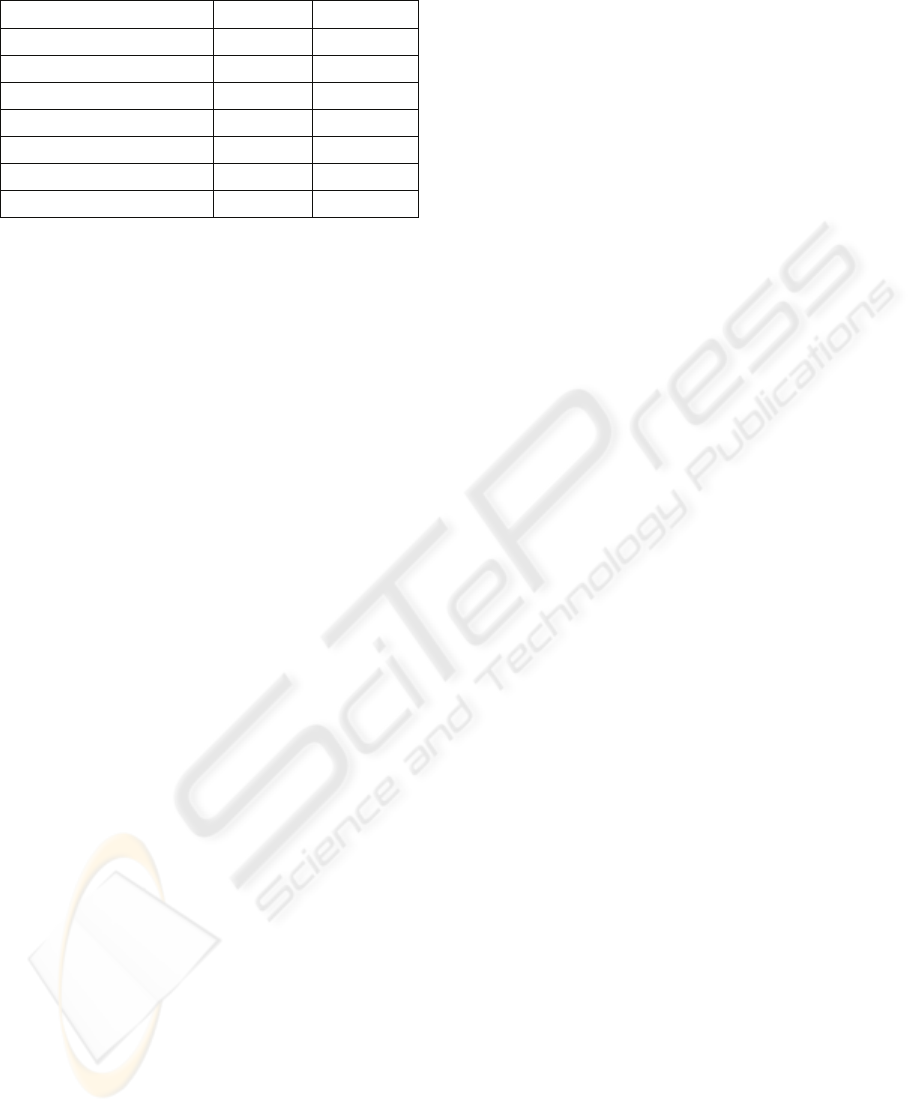
Table 1: Features of the two ontologies.
Onto_SV Onto_ST
Number of concepts 615 1251
Depth 6 6
Hierarchical IS_A relations yes yes
Properties No yes
Meronymy relation No yes
Other semantic relations No yes
Learning process Supervised Unsupervised
but it is the closer one to the domain knowledge
as expressed in the specification document.
4.2 Limitations and Advantages of our
Approach
The quality of the resulting ontology depends
entirely on the quality of the specification
document: when inconsistencies appear in the
specification file, human interpretation is required to
correct their consequences in the ontology. This is
one of the advantages of formalization: it helps
localize any fuzzy information or inconsistency
within highly structured documents like these
specifications. Whatever the effort made by their
authors, meaning variations (whether lexical,
syntactical or related to the text material
presentation) are one of the features of natural
language in text. While processing the document,
several such cases occurred: either the semantics of
the relation was not the expected one, or one of the
items of an enumeration had a different status from
others, etc. A detailed study is given in (Kamel and
Aussenac, 2009).
5 CONCLUSIONS AND FUTURE
WORK
We have shown that, in the very positive context
where texts are structured with well-defined tags
with a clear semantics, it is possible to define a text
processing chain that results efficient for the
automatic construction of an ontology. This chain,
implemented with the GATE platform, includes
rules that exploit together several features of the
document: its explicit structure through available
tags and its content in natural language. The
ontology obtained with this automatic process
results rich in concepts and relations, and each of its
element is precisely connected to the text from
which it originates. This method is applicable to all
XML documents referring database specifications
and validated by the INSPIRE standard.
We are aware that this ontology contains
inconsistencies that should be manually corrected.
In the scope of the GEONTO project, ontology
manual cleaning is planned.
For the time being, we feel like enriching the
ontology automatically built up, in particular thanks
to a more systematic analysis of definitions
(especially when they contain conjunctions or
disjunctions) and the text material presentation (we
have identified several kinds of typographic marks
that were not considered yet).
REFERENCES
Ahmad, K., Holmes-Higgin, P.R., 1995. SystemQuick : A
unified approach to text and terminology. In
Terminology in Advanced Microcomputer
Applications. Proceedings of the 3
rd
TermNet
Symposium.. 181-194. Vienna, Austria.
Asher, N., Busquet, J., Vieu, L., 2001. La SDRT: une
approche de la cohérence du discours dans la tradition
de la sémantique dynamique. Verbum 23, 73-101.
Auger, A., Barriere, C., 2008. Pattern based approaches to
semantic relation extraction: a state-of-the-art.
Terminology, John Benjamins, 14-1,1-19.
Aussenac-Gilles, N., Despres, S., Szulman, S. 2008. The
TERMINAE Method and Platform for Ontology
Engineering from texts. Bridging the Gap between
Text and Knowledge - Selected Contributions to
Ontology Learning and Population from Text. P.
Buitelaar, P. Cimiano (Eds.), IOS Press, p. 199-223.
Barrière, C., Agbado, A. 2006. TerminoWeb: a software
environment for term study in rich contexts.
International Conference on Terminology,
Standardization and Technology Transfert (TSTT
2006), Beijing (China), p. 103-113.
Bourigault, D., 2002. UPERY: un outil d’analyse
distributionnelle étendue pour la construction
d’ontologies à partir de corpus. TALN 2002, Nancy,
24-27 juin 2002
Buitelaar, P., Olejnik, D., Sintek, M., 2004. A Protégé
plug-in for ontology extraction from text based on
linguistic analysis. In Proceedings of the 1
st
European
Semantic Web Symposium (ESWS), p. 31-44.
Buitelaar, P., Cimiano, P., Magnini, B., 2005. Ontology
Learning From Text: Methods, Evaluation and
Applications. IOS Press.
Charolles, M., 1997. L’encadrement du discours:
Univers, Champs, Domaines et Espaces. Cahier de
Recherche Linguistique, LANDISCO, URA-CNRS
1035, Univ. Nancy 2, n°6, 1-73.
Daoust, F ., 1996. SATO (Système d’Analyse de Texte
par Ordinateur). Version 4.0. Manuel de référence,
Service d’Analyse de Texte par Ordinateur (ATO).
Montréal : Université du Québec
Giuliano, C., Lavelli, A., Romano, L., 2006. Exploiting
Shallow Linguistic Information for Relation
KEOD 2009 - International Conference on Knowledge Engineering and Ontology Development
164