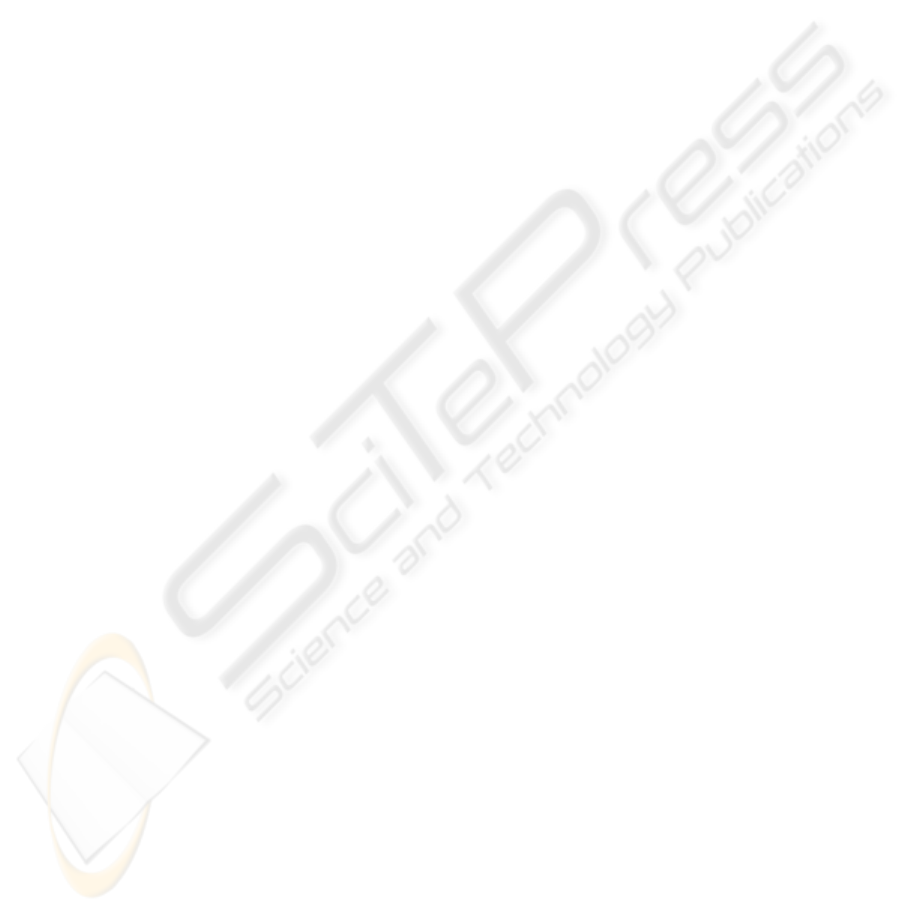
considering this method’s results. The given
capacities of the involved departments are
represented by constraints in the form of
inequations. In general, a constraint for a capacity
l=1,...,u is defined as shown in formula (5).
(5)
K is the limit of capacity l. The variant j exploits
units of capacity l. We call the capacity driver
of variant j. This inequation assures that the chosen
variants (x=1) do not exceed the capacity limit.
Inequations can be defined by the experts of each
department for all kinds of limited capacity
(e.g. storage capacity, machine time). To enhance
transparency, the data and the capacity constraints
should be published. The constraint that affects all
involved business processes is human ressource.
Hence, we introduce the manpower-constraint
exemplarily.
Increasing diversity strains human resources.
That is why the given manpower resources are a
very important constraint.
The mathematical definition of the manpower-
constraint is defined as follows:
(6)
For human resources both, time and transaction
drivers can be taken into account:
and
.
Normally a work step is characterized by the time
that is needed to accomplish it. Sometimes,
especially for administrative tasks, this cannot be
detected separately. A time lump-sum per
transaction is needed. Potential units for the capacity
drivers as well as the capacity limit K are man-day,
man-month or man-year.
In the same manner we defined transaction and
stock constraints for the purchasing and logistics
department. A special stock constraint for the
production area considers the limited space for
different variants in an assembly area to avoid
special picking areas:
·
(7)
For assembling, variant j is provided in a
standard box that occupies the space d. In
conjunction with the number of boxes (z) that have
to be provided for variant j and the total space
available K, this constraint assures that the
production area does not need cost-intensive picking
areas.
And finally we defined a production constraint to
take machine hours and set-up time into account.
Many more are imaginable for different problems.
3.2 Solving IVOM
At this stage the variant decision problem is split and
formulated in the objective function and the
constraining inequations. In the next step the
described model of the variant decision problem has
to be solved by an applicable algorithm.
Algorithms were developed for different types of
problems. First of all we have to characterize IVOM
as a specific problem type. Secondly we select one
of the possible algorithms for this type of problem
that promises the best and efficient solution.
The variant decision problem as it is represented
by IVOM is a special knapsack problem. It has more
than one constraint; strictly it might have multiple
constraints for every department and all kinds of
capacities. This type of problem is called a
multidimensional knapsack problem
(Kellerer, 2004).
Concerning its computing time, a study by
Kennedy and Spears (Kennedy, 1998) came to the
conclusion that particle swarm algorithms are most
suitable for complex multidimensional binary
problems. Hence, to find the optimum solution of
IVOM we used a particle swarm algorithm.
3.3 Qualitative Aspects of the Variant
Decision
After IVOM is properly defined for a given variant
decision problem the swarm algorithm is able to
identify its optimal solution. The found quantitative
optimal solution might be in conflict with qualitative
constraints, e.g. the brand image.
The proceeding of the particle swarm algorithm
allows saving several very good solutions, delivering
optimal valid solutions within the defined constrains.
In every step of the algorithm the best position of
each particle is saved. This arises the opportunity to
add a qualitative analysis made by the
interdisciplinary team after the algorithm identified a
set of the best solutions, giving optimal decision
support.
3.4 Decision Support through IVOM
The result of the IVOM approach is a list, consisting
of all valid, optimum solutions found by the particle
KEOD 2009 - International Conference on Knowledge Engineering and Ontology Development
410