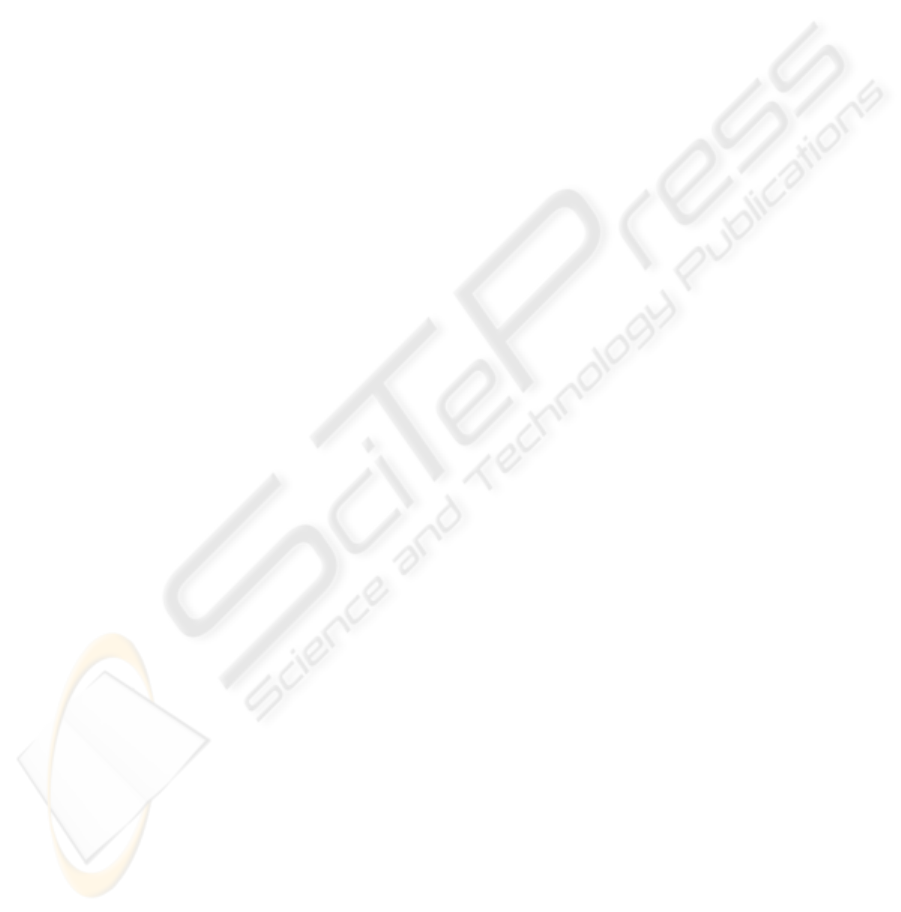
reduced probability of match between descriptions
for conceptually similar but lexically or syntactically
dissimilar linguistic expressions, and thereby lead to
a decreased recall. Thus, we need a set of relations
to express semantics, but we aim at keeping this set
at a manageable size in order to obtain the best
possible match.
5 QUERYING INFORMATION
AND KNOWLEDGE
Given a domain ontology as shown above and a set
of documents in which concepts have been
identified, the task is to provide means for query
interpretation and evaluation that draws on
conceptual content and exploits the conceptualis-
ation in the ontology.
In the present approach, query evaluation relies
on comparison of a conceptual description of the
query with conceptual descriptions of texts from the
database. A conceptual description is a set of
conceptual feature structures providing a mapping
from the text or the query to the ontology. Search in
a text collection indexed by concepts can employ
concept similarity-measures so that conceptual
reasoning can be replaced by simple similarity
computation, thereby allowing for a scaling to very
large information bases. Thus, a major challenge is
to define conceptual description similarity in terms
of the structure and relations in the ontology.
One obvious way to measure similarity in
ontologies is to evaluate the distance in the graphical
representation between the concepts being
compared, where shorter distance implies higher
similarity. A number of different ontological
similarity measures have been proposed along these
lines, for instance, Shortest Path Length (Rada,
1989), Information Content (Resnik, 1999), see also
(Budanitsky and Hirst, 2006).
An essential part of document querying is to
establish a mapping that, given a description for the
query, indicates matching – or similar – descriptions
for texts. One option is to let similarity reflect the
skeleton ontology by deriving it from the syntactic
derivation relation for conceptual feature structures,
where longer derivation paths correspond to smaller
degree of similarity. However, the comparison of
conceptual descriptions should not be merely
syntactic. Rather, description resemblance can be
measured in terms of similarity derived from all
concept relations in the ontology. Initially, in the
processing of a query, a description is generated.
Then this query description is compared, in
principle, to every conceptual description of every
document appearing in the database. Finally,
documents are ranked by the degree to which their
respective descriptions resemble the conceptual
description of the query. The query answer is a
ranking of the documents that are most similar to the
query.
In a framework where the domain of texts is
reflected in a knowledge base, as comprised by the
ontology, obviously not only the texts, but also the
domain ontology may in some cases be the target of
interest for queries. Knowledge about existence of
concepts, how concepts are related and about
similarities between concepts is also relevant. In
addition knowledge about the actual content of texts
can be viewed through the ontology simply by
means of revealing only concepts that exist in the
texts. In other words, the ontology plays a specific
role here, since it constitutes the means by which we
can obtain a conceptual view of the texts content.
Thus as an additional functionality, the user may
browse the generative ontology directly and then
follow the links to the relevant text parts by
descending to an ontological level of specialisation
with a manageable number of links to the target text.
6 CONCLUSIONS
We have presented an approach to representing,
organizing, and accessing conceptual content of
biomedical texts using a formal ontology. In
particular, we have presented the key ideas
addressing exploitation of ontologies for carrying
out content-based text search within a scientific
domain recognising not only synonyms but also
more general paraphrasations. Presently, we have
working prototypes. However, the viability of the
approach remains to be validated on a large scale, in
particular whether the devised ontological text
processing prototypes afford a
significant improvement compared with
conventional keyword search.
REFERENCES
Andreasen, T., Fischer Nilsson, J., 2004. Grammatical
Specification of Domain Ontologies. In Data &
Knowledge Engineering, 48, p. 221-230
Budanitsky, A., Hirst, G., 2006. Evaluating WordNet-
based measures of semantic distance. Computational
Linguistics, 32(1).
SIABO - Semantic Information Access through Biomedical Ontologies
175