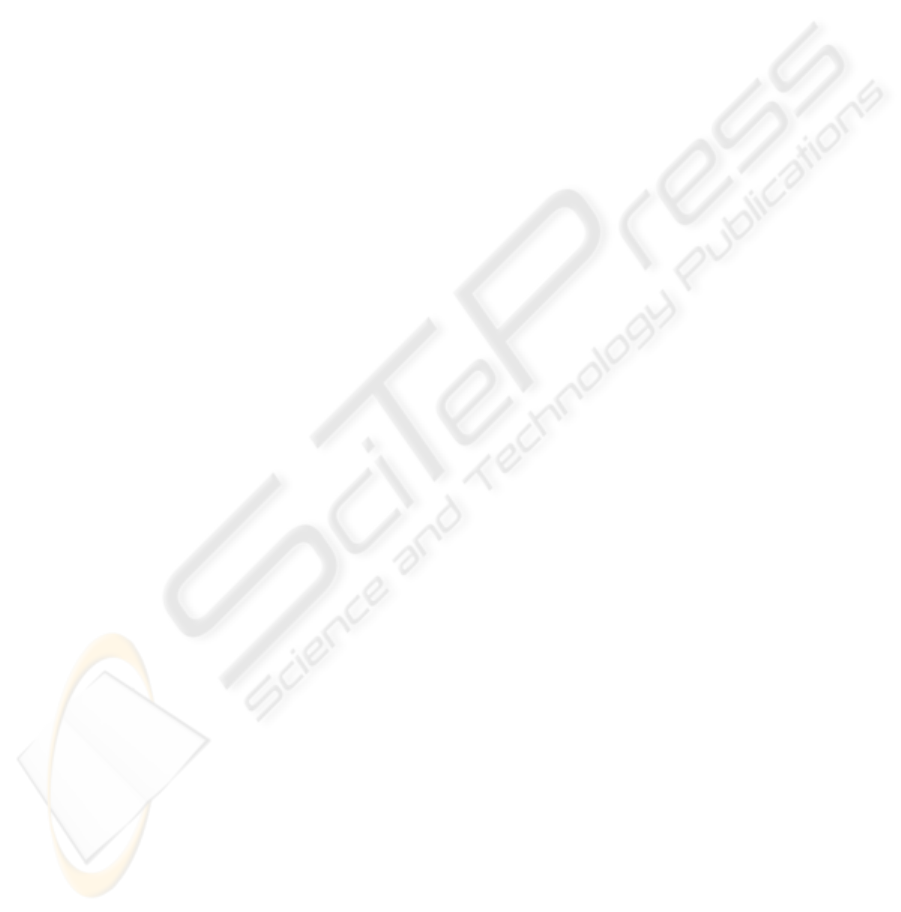
6 CONCLUSIONS
Word usage in context often defies our best attempts
to exhaustively enumerate all the possible senses of
a word (e.g., see Cruse, 1986). Though resources
like WordNet are generally very useful for language-
processing tasks, it is unreasonable to assume that
WordNet – or any print dictionary, for that matter –
offers a definitive solution to the problem of lexical
ambiguity. As we have seen here, the senses that
words acquire in specific contexts are sometimes at
great variance to the official senses that these words
have in dictionaries (Kilgarriff, 1997). It is thus
unwise to place too great a reliance on dictionaries
when acquiring ontological structures from corpora.
We have described here a lightweight approach
to the acquisition of ontological structure that uses
WordNet as little more than an inventory of nouns
and adjectives, rather than as an inventory of senses.
The insight at work here is not a new one: one can
ascertain the semantics of a term by the company it
keeps in a text, and if enough inter-locking patterns
are employed to minimize the risk of noise, real
knowledge about the use and meaning of words can
be acquired (Widdows and Dorow, 2002). Because
words are often used in senses that go beyond the
official inventories of dictionaries (e..g., recall our
examples of Playboy, Penthouse, Apollo, Mercury,
Sun and Apple), resources like WordNet can actually
be an impediment to achieving the kinds of semantic
generalizations demanded by a domain ontology.
A lightweight approach is workable only if other
constraints take the place of lexical semantics in
separating valuable ontological content from ill-
formed or meaningless noise. In this paper we have
discussed two such inter-locking constraints, in the
form of clique structures and analogical mappings.
Clique structures winnow out coincidences in the
data to focus only on patterns that have high internal
consistency. Likewise, analogical mappings enforce
a kind of internal symmetry on an ontology, biasing
a knowledge representation toward parallel
structures that recur in many different categories.
We have focused here on our own ontology,
NameDropper, created to annotate online newspaper
content. Our subsequent focus will expand to
include other, larger ontologies extracted from web-
content, including DBpedia and other Wikipedia-
derived resources (see Auer et al., 2007; Fu and
Weld, 2008). The category structure of Wikipedia is
sufficiently similar to that of NameDropper (in its
use of complex labels with internal linguistic
structure) that the analogical techniques described
here should be readily applicable. We shall see.
REFERENCES
Auer, S., Bizer, C., Lehmann, J., Kobilarov, G., Cyganiak,
R., Ives, Z., 2007. Dbpedia: A nucleus for a web of
open data. In Proc. of the 6
th
International Semantic
Web Conference, ISWC07.
Barsalou, L. W., 1983. Ad hoc categories. Memory and
Cognition, 11:211–227.
Brants, T., Franz, A., 2006. Web 1t 5-gram version 1.
Linguistic Data Consortium, Philadelphia.
Bron, C., Kerbosch, J., 1973. Algorithm 457: Finding all
cliques of an undirected graph. Communications of the
ACM 16(9). ACM press, New York.
Budanitsky, A., Hirst, G., 2006. Evaluating WordNet-
based Measures of Lexical Semantic Relatedness.
Computational Linguistics, 32(1):13-47.
Croitoru, M., Hu, B., Srinandan, D., Lewis, P., Dupplaw,
D., Xiao, L., 2007. A Conceptual Graph-based
Approach to Ontology Similarity Measure. In Proc. Of
the 15
th
International Conference On Conceptual
Structures, ICCS 2007, Sheffield, UK.
Cruse, D. A., 1986. Lexical Semantics. Cambridge, UK”
Cambridge University Press.
De Leenheer, P., de Moor, A., 2005. Context-driven
Disambiguation in Ontology Elicitation. In Shvaiko P.
& Euzenat J. (eds.), Context and Ontologies: Theory,
Practice and Applications, AAAI Technical Report
WS-05-01:17–24. AAAI Press.
Dong, Z., Dong, Q., 2006. HowNet and the Computation
of Meaning. World Scientific. Singapore.
Euzenat, J., Shvaiko, P., 2007. Ontology Matching.
Springer Verlag. Heidelberg.
Falkenhainer, B., Forbus, K., Gentner, D., 1989. Structure-
Mapping Engine: Algorithm and Examples. Artificial
Intelligence, 41:1-63
Fellbaum, C., (ed.). 1998. WordNet: An Electronic Lexical
Database. The MIT Press, Cambridge, MA.
Gruber, T., 1993. A translation approach to portable
ontologies. Knowledge Acquisition, 5(2):199-220.
Guarino, N., (ed.) 1998. Formal Ontology and Information
Systems. Amsterdam: IOS Press. Proceedings of
FOIS1998, June 6-8, Trento, Italy.
Hearst, M., 1992. Automatic acquisition of hyponyms
from large text corpora. Proc. of the 14
th
International
Conference on Computational Linguistics, pp 539–
545.
Kilgarriff, A., 1997. I don’t believe in word senses.
Computers and the Humanities, 31(2), 91-113.
Veale, T., Li, G., Hao, Y., 2009. Growing Finely-
Discriminating Taxonomies from Seeds of Varying
Quality and Size. In Proc. of EACL 2009, Athens.
Widdows, D., Dorow, B., 2002. A graph model for
unsupervised lexical acquisition. In Proc. of the 19
th
Int. Conference on Computational Linguistics.
Wu, F., Weld, D. S., 2008. Automatically Refining the
Wikipedia Infobox Ontology. In Proc. of the 17
th
World Wide Web Conference, WWW 2008.
ONTOLOGICAL CLIQUES - Analogy as an Organizing Principle in Ontology Construction
41