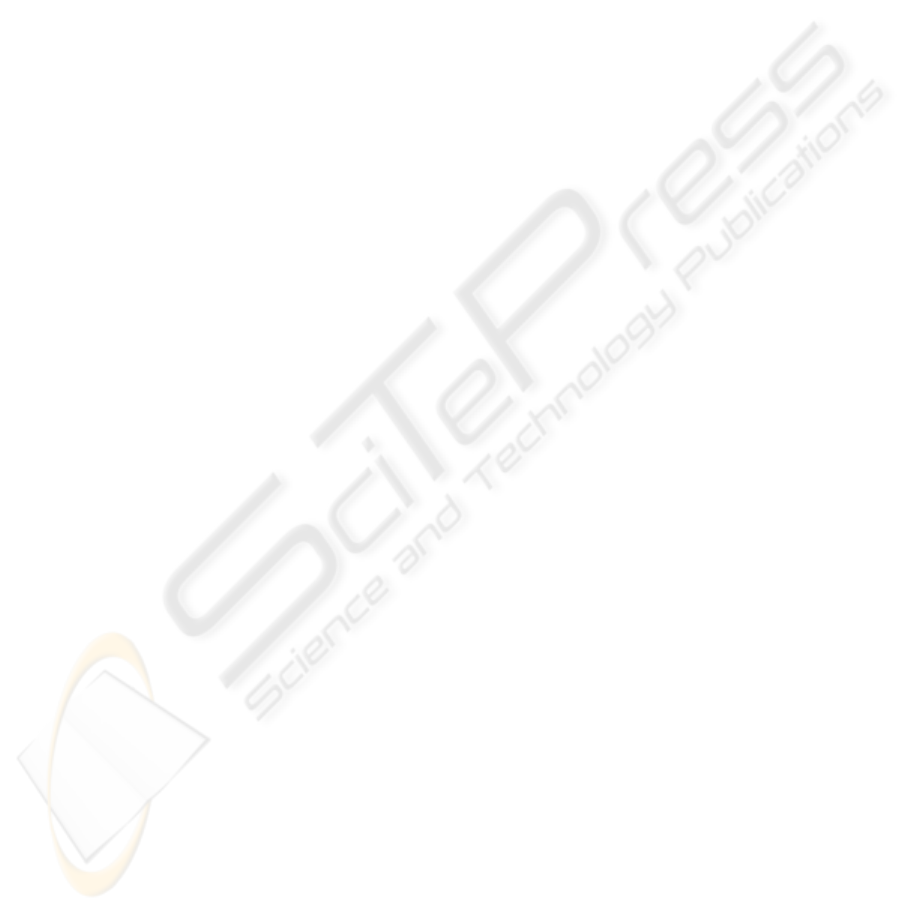
strings. For this type of strings, LEVENSHTEIN and
JARO-WINKLER measures are more adapted to com-
pute the linguistic similarity. Comments are strings
composed with many words. For this type strings, the
Q-GRAM measure gives the best linguistic similarity
values. Columns 2-3 of Table 1 of the Appendix show
precision and recall values for the best combination.
The next subsection will compare OACAS vs other
related methods.
3.3 Comparative Study
In order to evaluate the results obtained by OACAS,
Tables 1 and 2 of the Appendix show the obtained pre-
cision and recall values. They also represent values
respectively obtained by FALCON-AO, ONTODNA
and RIMOM methods. With respect to Tables 1 and
2, the OACAS method produces better results than
the three others methods, in particular in the 30x fam-
ily tests. Theses tests represent real ontologies. The
OACAS method gives worse results in the 26x family
tests. In this family of tests, ontological entities do not
have properties (names and comments). Interestingly
enough, these ontological components are the main
factors in computing the alignment score using the
OACAS method. The experimental results also high-
light that the performances of the OACAS method are
highly linked to the different characteristics of onto-
logical components (names, comments and labels).
In the case where the descriptors of the entities are
dropped, the quality (precision and recall values) of
the alignment is degraded. For example, in the tests
257 and 260 the ontological entities are not described
neither by names nor by comments. In addition, rela-
tions and properties are absent. Whenever the name
and comments descriptors are not present, the values
of linguistic similarity measures are lowered and con-
sequently the value of the composed linguistic simi-
larity will follow the same tendency. Moreover, when
the considered ontologies to be aligned do not contain
relations nor properties, the values of neighborhood
similarities decrease. The local (linguistic similarity
composition and neighborhood similarity) similarity
computation in the OACAS method reduces the ag-
gregation similarity value. For this reason, precision
and recall values are degraded.
4 CONCLUSIONS
In this paper, we introduced a new alignment method
of OWL-DL ontologies. The new proposed method
OACAS, allows to exploit at most the informative
present within in an ontology described in OWL-DL.
The process of alignement in the OACAS method,
contains two phases: a local phase and a phase of
aggregation. The local phase allows to calculate the
linguistic similarity consisted as well as the neighbor-
hood similarity. This two similarities are combined
during the second phase to determine the aggregation
similarity. The results obtained by the method OA-
CAS are very encouraging, compared with the results
obtained by the methods FALCON-AO, ONTODNA
and RiMOM. The method OACAS shows more suc-
cessful results compared to the other methods in par-
ticular on the real ontologies (the family of the tests
30x). In order to improve the OACAS method, some
improvements can be brought. Indeed, the method
OACAS have to deal with ontologies of huge sizes.
The integration of the API WORDNET (Miller, 1995)
is necessary, to improve the values of the measures of
the linguistic similarity.
REFERENCES
Euzenat, J. (2001). Towards a principled approach to se-
mantic interoperability. In Proceedings of the IJ-
CAI Workshop on Ontology and Information Sharing,
pages 19–25, Seattle, US.
Euzenat, J., Mochol, M., Shvaiko, P., Stuckenschmidt, H.,
Svab, O., Svatek, V., van Hage, W. R., and Yatskevich,
M. (2006). Results of the ontology alignment evalua-
tion initiative 2006. In Proceedings of the First ESWC
2006 international workshop on ontology matching.
Euzenat, J. and Shvaiko, P. (2007). Ontology Matching.
Springer-Verlag, Heidelberg (DE).
Gruber, T. (1993). A translation approach to portable ontol-
ogy specifications. Knowledge Acquisition, 5(2):199–
220.
Hu, W., Zhao, Y., Li, D., Cheng, G., Wu, H., and Qu, Y.
(2007). Falcon-AO: results for OAEI 2007. In Pro-
ceedings of the 2
nd
International Workshop on On-
tology Matching (OM-2007), pages 170–178, Busan,
Korea.
Kiu, C. and Lee, C. (2007). OntoDNA: ontology align-
ment results for OAEI 2007. In Proceedings of the 2
nd
International Workshop on Ontology Matching (OM-
2007), pages 227–235, Busan, Korea.
Li, Y., Zhong, Q., Li, J., and Tang, J. (2007). Result of on-
tology alignment with RiMOM at OAEI’07. In Pro-
ceedings of the 2
nd
International Workshop on On-
tology Matching (OM-2007), pages 196–205, Busan,
Korea.
Miller, G. A. (1995). WORDNET: a Lexical Database for
English. Communications of the ACM, 38(11):39–41.
Ukkonen, E. (1992). Approximate string-matching with q-
grams and maximal matches. Theoretical Computer
Science, 92(1):191–211.
OACAS - Ontologies Alignment using Composition and Aggregation of Similarities
237