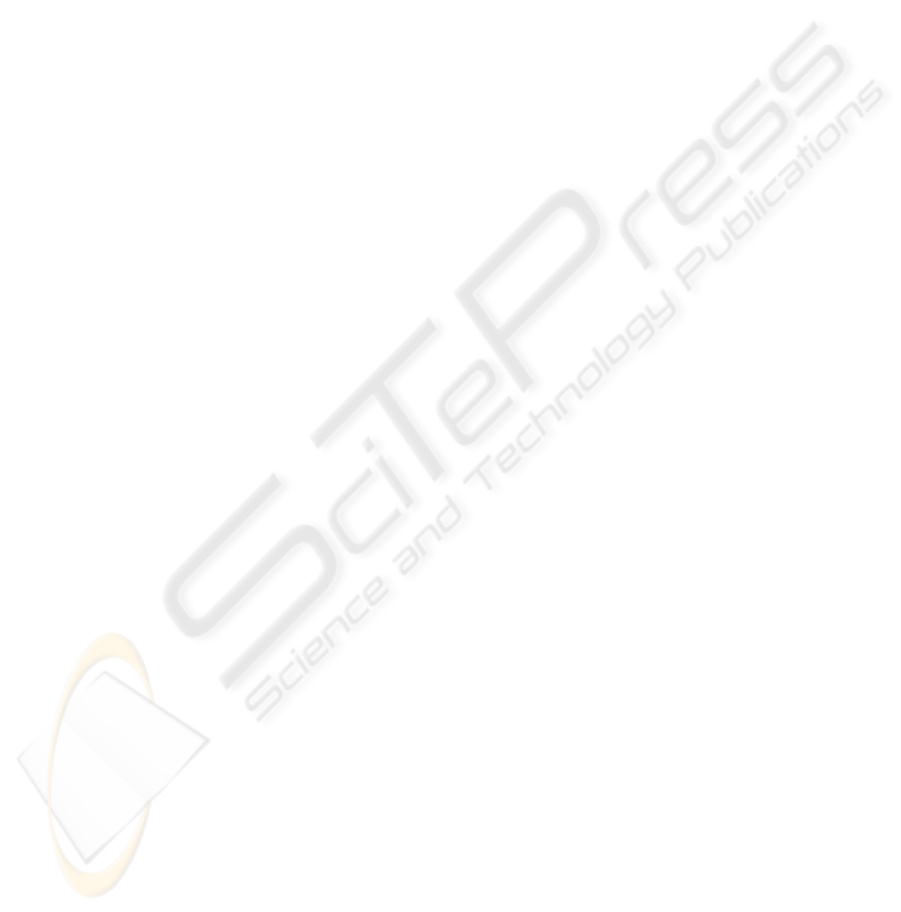
shown in Section 5 that the system is able to create an
ontology draft containing both ontological and termi-
nological elements and enriches several dimensions
of the previous prototype (see Section 6):
1. It is able to deal with richer linguistic information
as long as agents take into account lexical rela-
tions found by matching patterns on texts.
2. The result is much richer: DYNAMO builds up a
TOR which includes a hierarchy of concepts with
their related terms, and labelled semantic relations
between concepts. A set of terms denotes each
particular concept, which is useful for the docu-
ment annotation activity.
3. The current DYNAMO system is able to deal
either with French or English language text,
whereas the first prototype was previously limited
to French language.
According to the project schedule we need to improve
the software during the next year. To do so we plan
to:
• introduce the cooperative behaviour of ConceptA-
gents (specification of NCS and their treatment);
• provide an adaptive patterns learning process
based on the AMAS theory;
• provide specific interfaces to enable ontologists
collaboration;
• apply the DYNAMO MAS to all the project do-
mains (archeology, car diagnosis, software bug re-
ports).
REFERENCES
Barri`ere, C. and Akakpo, A. (2006). Terminoweb: A soft-
ware environment for term study in rich contexts. In
Proceedings of International Conference on Terminol-
ogy, Standardization and Technology Transfer, pages
103–113, Beijing. Encyclopedia of China Publishing
House.
Bernon, C., Camps, V., Gleizes, M.-P., and Picard, G.
(2005). Engineering Adaptive Multi-Agent Systems:
The ADELFE Methodology . In Henderson-Sellers,
B. and Giorgini, P., editors, Agent-Oriented Method-
ologies, volume ISBN1-59140-581-5, pages 172–202.
Idea Group Pub, NY, USA.
Buitelaar, P., Cimiano, P., and Magnini, B. (2005). On-
tology Learning From Text: Methods, Evaluation and
Applications. IOS Press.
Capera, D., Georg´e, J.-P., Gleizes, M.-P., and Glize, P.
(2003). The AMAS Theory for Complex Problem
Solving Based on Self-organizing Cooperative Agents
. In TAPOCS 2003 at WETICE 2003, Linz, Austria,
09/06/03-11/06/03. IEEE CS.
Chagnoux, M., Hernandez, N., and Aussenac-Gilles, N.
(2008). An interactive pattern based approach for ex-
tracting non-taxonomic relations from texts. In Buite-
laar, P., Cimiano, P., Paliouras, G., and Spilliopoulou,
M., editors, Workshop on Ontology Learning and
Population (associated to ECAI 2008) (OLP), Patras
(Greece), 22/07/08, pages 1–6.
Cimiano, P. (2006). Ontology Learning and Population
from Text: Algorithms, Evaluation and Applications.
Springer.
Cimiano, P. and V¨olker, J. (2005). Text2onto - a framework
for ontology learning and data-driven change discov-
ery. In Montoyo, A., Munoz, R., and Metais, E., ed-
itors, Proceedings of the 10th International Confer-
ence on Applications of Natural Language to Informa-
tion Systems (NLDB), volume 3513 of Lecture Notes
in Computer Science, pages 227–238, Alicante, Spain.
Springer.
Gruber, T. R. (1993). A Translation Approach to Portable
Ontology Specifications. Knowledge Acquisition,
5(2):199–221.
Haase, P. and Sure, Y. (2004). D3.1.1.b state-of-the-art on
ontology evolution. Technical report, Institute AIFB,
University of Karlsruhe.
Harris, Z. S. (1968). Mathematical Structures of Language.
Wiley, New York.
Hearst, M. A. (1992). Automatic acquisition of hyponyms
from large text corpora. In COLING, pages 539–545.
Maedche, A. (2002). Ontology learning for the Semantic
Web, volume 665. Kluwer Academic Publisher.
Malaise, V. (2005). M´ethodologie linguistique et
terminologique pour la structuration d’ontologies
diff´erentielles `a partir de corpus textuels. PhD thesis,
Paris 7 Denis Diderot University.
Morin, E. (1999). Using lexico-syntactic patterns to extract
semantic relations between terms from technical cor-
pus. In 5th International Congress on Terminology
and Knowledge Engineering (TKE), pages 268–278,
Innsbruck, Austria. TermNet.
Ottens, K., Hernandez, N., Gleizes, M.-P., and Aussenac-
Gilles, N. (2008). A Multi-Agent System for Dynamic
Ontologies. Journal of Logic and Computation, Spe-
cial Issue on Ontology Dynamics, 19:1–28.
Reymonet, A., Thomas, J., and Aussenac-Gilles, N. (2007).
Modelling ontological and terminological resources in
OWL DL. In Buitelaar, P., Choi, K.-S., Gangemi,
A., and Huang, C.-R., editors, OntoLex07 - From Text
to Knowledge: The Lexicon/Ontology Interface Work-
shop at ISWC07 6th International Semantic Web Con-
ference, Busan (South Korea), 11/11/07.
Velardi, P., Navigli, R., Cucchiarelli, A., and Neri, F.
(2005). Evaluation of OntoLearn, a Methodology for
Automatic Learning of Domain Ontologies. In Buite-
laar, P., O. Cimiano & B. Magnini (eds.). IOS Press,
Amsterdam.
DYNAMIC ONTOLOGY CO-CONSTRUCTION BASED ON ADAPTIVE MULTI-AGENT TECHNOLOGY
63