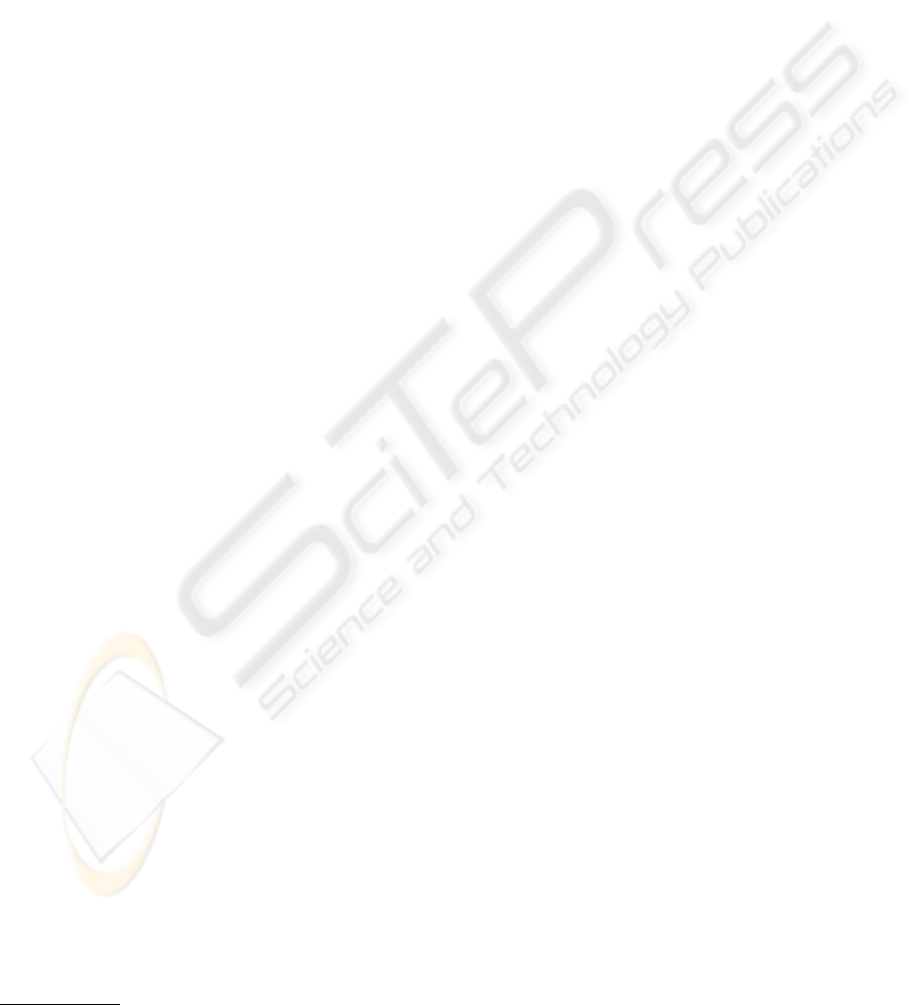
et al., 2006) no experiments are presented. Never-
theless, it’s worth mentioning that in (Meilicke et al.,
2007; Meilicke et al., 2008a) the average gain in pre-
cision is 16% but the error rate is omitted, while in
(Meilicke et al., 2008b) the average gain in precision
is 16% (with average error rate 1%) and 18% (with
average error rate 5%), using reference disjointness
and learned disjointness respectively.
5 CONCLUSIONS AND FUTURE
WORK
This paper focuses on a new automatic approach for
repairing ontology alignments based on WSD tech-
niques, in particular on the Adapted Lesk algorithm,
and on upper ontologies used to enrich the context
necessary to compute the meaning disambiguation of
concepts and thus process the repairing task.
Our future work will focus on the extension of the
context window for each concept of a mapping by ex-
ploiting the local context available in the input on-
tologies, such as for example sub- and super concepts
structure as well as other related concepts. Free text
comments attached to the concept can also contribute
to the context construction.
Another extension we wish to implement, in order
to lower the error rate, is the refinement of the scores
computation, considering all the possible overlaps be-
tween two glosses in the final score composition, as
well as introducing a threshold to filter more relevant
winner glosses.
A comparative evaluation between our approach
and the other existing completely automatic method-
ologies for mappings repair on the same test set is an-
other activity that we are going to accomplish.
We also wish to exploit other WSD techniques for
both mappings repair and ontology matching tasks.
The design and implementation of an ontology match-
ing procedure based on Adapted Lesk and the ex-
ploitation of local context as well as upper ontologies
is on its way.
ACKNOWLEDGEMENTS
The work of the first author was partly supported
by the KPLab project funded by EU 6th FP. The
work of the second author was partly supported by
the Italian research project Iniziativa Software CINI-
FinMeccanica.
Dataset, with 39 concepts on average.
REFERENCES
Agirre, E. and Edmonds, P. (2007). Word Sense Disam-
biguation - Algorithms and Applications. Springer.
Banerjee, S. and Pedersen, T. (2002). An adapted Lesk al-
gorithm for word sense disambiguation using Word-
Net. In Gelbukh, A. F., editor, CICLing 2002, volume
2276 of LNCS, pages 136–145. Springer.
Do, H. H., Melnik, S., and Rahm, E. (2002). Compar-
ison of schema matching evaluations. In Chaudhri,
A. B., Jeckle, M., Rahm, E., and Unland, R., editors,
NODe 2002, volume 2593 of LNCS, pages 221–237.
Springer.
Euzenat, J. and Shvaiko, P. (2007). Ontology Matching.
Springer.
Fellbaum, C., editor (1998). WordNet – An Electronic Lexi-
cal Database. The MIT Press.
Gangemi, A., Guarino, N., Masolo, C., Oltramari, A., and
Schneider, L. (2002). Sweetening ontologies with
DOLCE. In G
´
omez-P
´
erez, A. and Benjamins, V. R.,
editors, EKAW 2002, volume 2473 of LNCS, pages
166–181. Springer.
Haeri, S. H., Hariri, B. B., and Abolhassani, H. (2006).
Coincidence-based refinement of ontology matching.
In SCIS+ISIS 2006.
Lenat, D. and Guha, R. (1990). Building large knowledge-
based systems. Addison Wesley.
Lesk, M. (1986). Automatic sense disambiguation using
machine readable dictionaries: how to tell a pine cone
from an ice cream cone. In SIGDOC ’86, pages 24–
26. ACM.
Lin, F. and Sandkuhl, K. (2008). A survey of exploiting
wordnet in ontology matching. In Bramer, M., ed-
itor, IFIP AI, volume 276 of IFIP, pages 341–350.
Springer.
Mascardi, V., Locoro, A., and Rosso, P. (2009). Automatic
ontology matching via upper ontologies: A systematic
evaluation. IEEE Trans. Knowl. Data Eng., to appear.
Meilicke, C., Stuckenschmidt, H., and Tamilin, A. (2007).
Repairing ontology mappings. In AAAI 2007, pages
1408–1413. AAAI Press.
Meilicke, C., Stuckenschmidt, H., and Tamilin, A. (2008a).
Reasoning support for mapping revision. J. Logic and
Computation.
Meilicke, C., V
¨
olker, J., and Stuckenschmidt, H. (2008b).
Learning disjointness for debugging mappings be-
tween lightweight ontologies. In Gangemi, A. and
Euzenat, J., editors, EKAW, volume 5268 of LNCS,
pages 93–108. Springer.
Niles, I. and Pease, A. (2001). Towards a standard upper
ontology. In Welty, C. and Smith, B., editors, FOIS
2001, pages 2–9. ACM.
KEOD 2009 - International Conference on Knowledge Engineering and Ontology Development
246