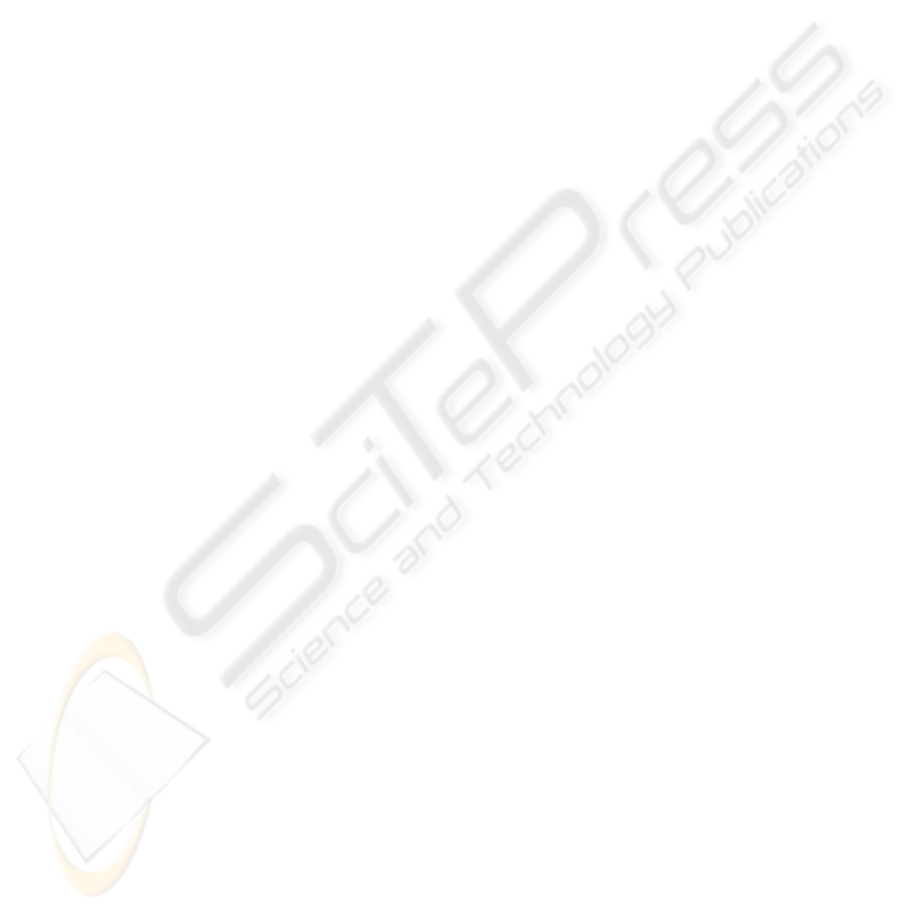
A general problem in the context of scene classifica-
tion is the processing of images that only show parts
of a larger scene. Essentially, this means that it is
not possible to reason on the basis of an object class’
absence. While the explicit representation of uncer-
tainty reduces the severity of the problem in practice,
there is always a chance of miss-classifying a scene
due to critical objects being out of view. A possible
solution to this problem could be to have the system
analyze images taken at different view points in the
scene.
In the future, we plan to integrate the presented
scene classification system into a mobile agent (Zet-
zsche et al., 2008). Not only does this provide the
system with a strong prior due to the agent’s past ob-
servations, the mobility would also ease the problem
of only sensing parts of a scene. In particular, this will
require the detection of objects to be performed with-
out any pre-segmentation, which is why we are cur-
rently working on providing the system with a more
sophisticated vision module. This will also allow us
to produce more conclusive experimental results on
other data sets. Finally, we think it would be inter-
esting to see whether the generic approach of reason-
ing based on ontologies and statistics in a belief-based
framework could be applied to other domains beyond
scene classification.
ACKNOWLEDGEMENTS
This work was supported by DFG, SFB/TR8 Spa-
tial Cognition, projects A5-[ActionSpace] and I1-
[OntoSpace].
REFERENCES
Baader, F., Calvanese, D., McGuinness, D., Nardi, D., and
Patel-Schneider, P. (2003). The Description Logic
Handbook. Cambridge University Press.
Dubois, D. and Prade, H. (1986). On the unicity of Demp-
ster’s rule of combination. International Journal of
Intelligent Systems, 1(2):133–142.
Henderson, J. and Hollingworth, A. (1999). High-level
scene perception. Annual Review of Psychology,
50(1):243–271.
Horridge, M. and Patel-Schneider, P. F. (2008). Manchester
OWL syntax for OWL 1.1. OWL: Experiences and
Directions (OWLED 08 DC), Gaithersberg, Mary-
land.
Horrocks, I., Kutz, O., and Sattler, U. (2006). The Even
More Irresistible SROIQ. In Knowledge Representa-
tion and Reasoning (KR). AAAI Press.
Kollar, T. and Roy, N. (2009). Utilizing object-object and
object-scene context when planning to find things. In
International Conference on Robotics and Automation
(ICRA).
Konev, B., Lutz, C., Walther, D., and Wolter, F. (2009). For-
mal properties of modularisation. In Stuckenschmidt,
H., Parent, C., and Spaccapietra, S., editors, Modular
Ontologies. Springer.
Maillot, N. E. and Thonnat, M. (2008). Ontology based
complex object recognition. Image and Vision Com-
puting, 26(1):102–113.
Mart
´
ınez Mozos,
´
O., Triebel, R., Jensfelt, P., Rottmann, A.,
and Burgard, W. (2007). Supervised semantic label-
ing of places using information extracted from sensor
data. Robotics and Autonomous Systems, 55(5):391–
402.
Masolo, C., Borgo, S., Gangemi, A., Guarino, N., and
Oltramari, A. (2003). Ontologies library. WonderWeb
Deliverable D18, ISTC-CNR.
Oliva, A. and Torralba, A. (2001). Modeling the shape
of the scene: A holistic representation of the spatial
envelope. International Journal of Computer Vision,
42(3):145–175.
Pal, N., Bezdek, J., and Hemasinha, R. (1993). Uncertainty
measures for evidential reasoning II: A new measure
of total uncertainty. International Journal of Approxi-
mate Reasoning, 8(1):1–16.
Russell, B., Torralba, A., Murphy, K., and Freeman, W.
(2008). LabelMe: a database and web-based tool for
image annotation. International Journal of Computer
Vision, 77(1–3):157–173.
Schill, K., Umkehrer, E., Beinlich, S., Krieger, G., and
Zetzsche, C. (2001). Scene analysis with saccadic
eye movements: Top-down and bottom-up modeling.
Journal of Electronic Imaging, 10(1):152–160.
Schill, K., Zetzsche, C., and Hois, J. (2009). A belief-
based architecture for scene analysis: From sensori-
motor features to knowledge and ontology. Fuzzy Sets
and Systems, 160(10):1507–1516.
Schneiderman, H. and Kanade, T. (2004). Object detection
using the statistics of parts. International Journal of
Computer Vision, 56(3):151–177.
Shafer, G. (1976). A Mathematical Theory of Evidence.
Princeton University Press, Princeton, NJ.
Sirin, E., Parsia, B., Grau, B. C., Kalyanpur, A., and Katz,
Y. (2007). Pellet: A practical OWL-DL reasoner. Web
Semantics: Science, Services and Agents on the World
Wide Web, 5(2):51–53.
Smets, P. (1992). The nature of the unnormalized beliefs
encountered in the transferable belief model. In Un-
certainty in Artificial Intelligence, pages 292–297.
Smets, P. and Kennes, R. (1994). The transferable belief
model. Artificial intelligence, 66(2):191–234.
Vernon, D. (2008). Cognitive vision: The case for embodied
perception. Image and Vision Computing, 26(1):127–
140.
Zetzsche, C., Wolter, J., and Schill, K. (2008). Sensorimo-
tor representation and knowledge-based reasoning for
spatial exploration and localisation. Cognitive Pro-
cessing, 9:283–297.
EVIDENTIAL COMBINATION OF ONTOLOGICAL AND STATISTICAL INFORMATION FOR ACTIVE SCENE
CLASSIFICATION
79