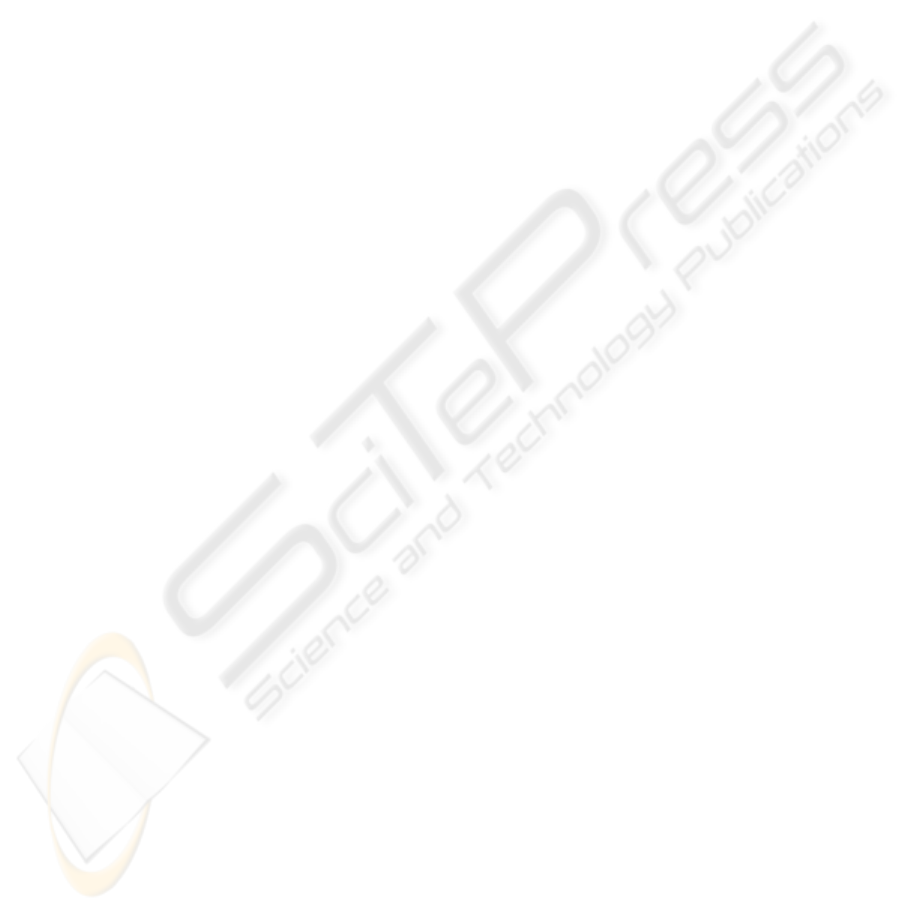
Dunn, J. (1974). Well separated clusters and optimal fuzzy
partitions. J. Cybern, 4:95–104.
Ester, M., Kriegel, H.-P., J
¨
org, S., and Xu, X. (1996).
A density-based algorithm for discovering clusters in
large spatial databases with noise.
Fern, X. and Brodley, C. (2004). Solving cluster ensem-
ble problems by bipartite graph partitioning. In ICML
’04: Proceedings of the twenty-first international con-
ference on Machine learning, page 36, New York, NY,
USA. ACM.
Fred, A. L. N. (2001). Finding consistent clusters in data
partitions. In MCS ’01: Proceedings of the Second In-
ternational Workshop on Multiple Classifier Systems,
pages 309–318, London, UK. Springer-Verlag.
Fred, A. L. N. and Jain, A. K. (2005). Combining multiple
clusterings using evidence accumulation. IEEE Trans.
Pattern Anal. Mach. Intell., 27(6):835–850.
Guha, S., Rastogi, R., and Shim, K. (1998). Cure: an ef-
ficient clustering algorithm for large databases. In
SIGMOD ’98: Proceedings of the 1998 ACM SIG-
MOD international conference on Management of
data, pages 73–84, New York, NY, USA. ACM.
Hadjitodorov, S. T., Kuncheva, L. I., and Todorova, L. P.
(2006). Moderate diversity for better cluster ensem-
bles. Inf. Fusion, 7(3):264–275.
Halkidi, M., Batistakis, Y., and Vazirgiannis, M. (2001).
Clustering algorithms and validity measures. In Tu-
torial paper in the proceedings of the SSDBM 2001
Conference.
Hubert, L. and Arabie, P. (1985). Comparing partitions.
Journal of Classification.
Hubert, L. and Schultz, J. (1975). Quadratic assignment
as a general data-analysis strategy. British Journal
of Mathematical and Statistical Psychology, 29:190–
241.
Jouve, P. and Nicoloyannis, N. (2003). A new method
for combining partitions, applications for distributed
clustering. In International Workshop on Paralell
and Distributed Machine Learning and Data Mining
(ECML/PKDD03), pages 35–46.
Karypis, G., Eui, and News, V. K. (1999). Chameleon: Hi-
erarchical clustering using dynamic modeling. Com-
puter, 32(8):68–75.
Kaufman, L. and Roussesseeuw, P. (1990). Finding groups
in data: an introduction to cluster analysis. Wiley.
King, B. (1973). Step-wise clustering procedures. Journal
of the American Statistical Association, (69):86–101.
Kuncheva, L. and Hadjitodorov, S. (2004). Using diver-
sity in cluster ensembles. volume 2, pages 1214–1219
vol.2.
Macqueen, J. B. (1967). Some methods of classification
and analysis of multivariate observations. In Proceed-
ings of the Fifth Berkeley Symposium on Mathemtical
Statistics and Probability, pages 281–297.
Maulik, U. and Bandyopadhyay, S. (2002). Performance
evaluation of some clustering algorithms and validity
indices. IEEE Transactions on Pattern Analysis and
Machine Intelligence, 24(12):1650–1654.
Ng, R. T. and Han, J. (2002). Clarans: A method for clus-
tering objects for spatial data mining. IEEE Trans. on
Knowl. and Data Eng., 14(5):1003–1016.
Sneath, P. and Sokal, R. (1973). Numerical taxonomy. Free-
man, London, UK.
Strehl, A. and Ghosh, J. (2003). Cluster ensembles — a
knowledge reuse framework for combining multiple
partitions. J. Mach. Learn. Res., 3:583–617.
Topchy, A., Jain, A. K., and Punch, W. (2003). Combining
multiple weak clusterings. pages 331–338.
Topchy, A., Minaei-Bidgoli, B., Jain, A. K., and Punch,
W. F. (2004a). Adaptive clustering ensembles. In
ICPR ’04: Proceedings of the Pattern Recognition,
17th International Conference on (ICPR’04) Volume
1, pages 272–275, Washington, DC, USA. IEEE Com-
puter Society.
Topchy, A. P., Jain, A. K., and Punch, W. F. (2004b). A mix-
ture model for clustering ensembles. In Berry, M. W.,
Dayal, U., Kamath, C., and Skillicorn, D. B., editors,
SDM. SIAM.
Wang, W., Yang, J., and Muntz, R. R. (1997). Sting: A sta-
tistical information grid approach to spatial data min-
ing. In VLDB ’97: Proceedings of the 23rd Interna-
tional Conference on Very Large Data Bases, pages
186–195, San Francisco, CA, USA. Morgan Kauf-
mann Publishers Inc.
Ward, J. H. (1963). Hierarchical grouping to optimize an
objective function. Journal of the American Statistical
Association, 58(301):236–244.
Xie, X. and Beni, G. (1991). A validity measure for fuzzy
clustering. IEEE Transactions on Pattern Analysis
and Machine Intelligence, 13:841–847.
CLUSTER ENSEMBLE SELECTION - Using Average Cluster Consistency
95