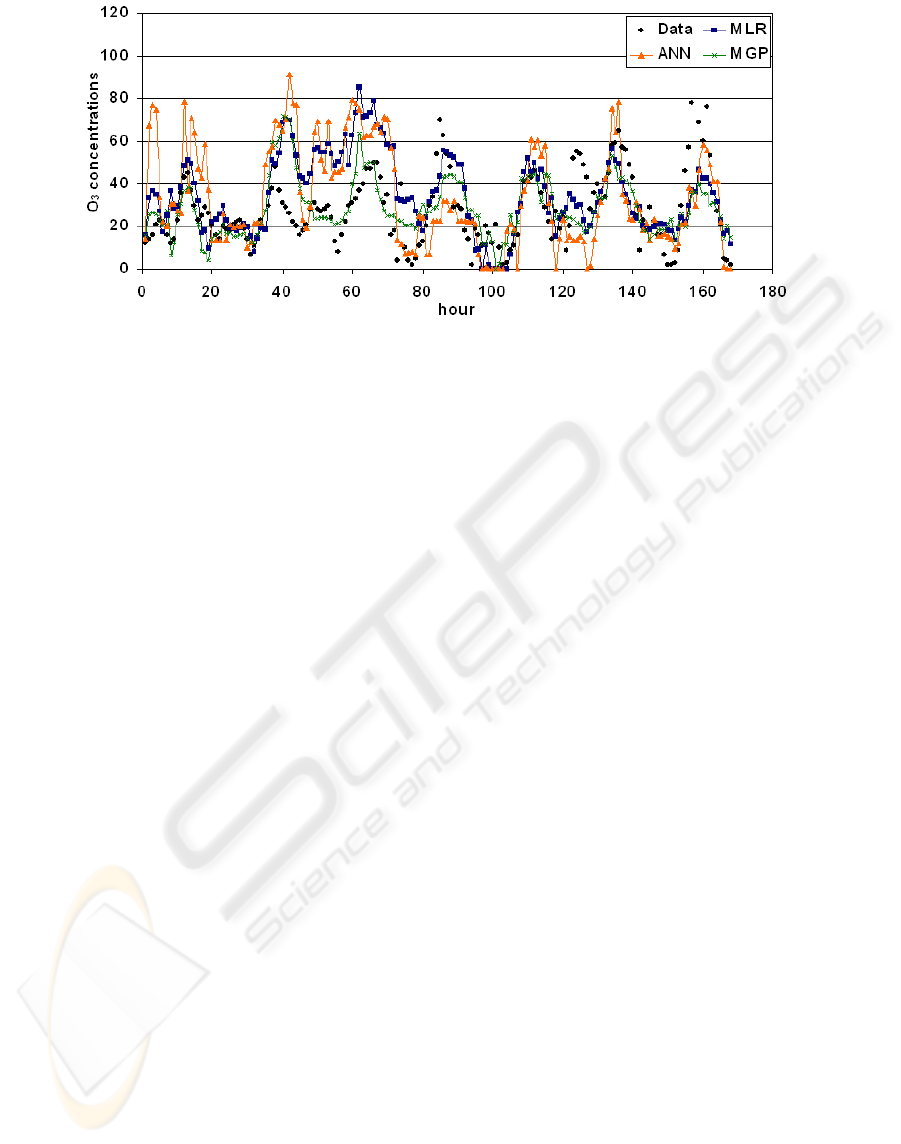
Figure 4: Prediction of O
3
concentrations for the test period.
5 CONCLUSIONS
Aiming the prediction of the next day hourly
average O
3
concentrations, the performances of
MLR, ANN and MGP were compared. The
prediction of seven consecutive days tested the
consistence of the models. ANN models presented
better results in the training step. However, with
regards to the aim of this study, MGP presented the
best predictions of O
3
concentrations (test set). The
good performances of the models showed that MGP
is a useful tool to public health protection as it can
provide early warnings to the population about O
3
concentrations episodes.
ACKNOWLEDGEMENTS
J.C.M. Pires also thanks the FCT for the fellowship
SFRH/BD/23302/2005.
REFERENCES
Al-Alawi, S.M., Abdul-Wahab, S. A., Bakheit, C. S.,
2008. Combining principal component regression and
artificial neural networks for more accurate
predictions of ground-level ozone. Environmental
Modelling & Software 23(4), 396-403.
Chiang, Y. M., Chang, L. C., Chang, F. J., 2004.
Comparison of static-feedforward and dynamic-
feedback neural networks for rainfall–runoff
modelling. Journal of Hydrology 290 (3-4), 297-311.
Dueñas, C., Fernández, M.C., Cañete, S., Carretero, J., and
Liger, E., 2002. Assessment of ozone variations and
meteorological effects in an urban area in the
Mediterranean Coast. Science of the Total
Environment 299 (1-3), 97-113.
Guerra, J.-C., Rodríguez, S., Arencibia, M.-T., García M.-
D., 2004. Study on the formation and transport of
ozone in relation to the air quality management and
vegetation protection in Tenerife (Canary Islands).
Chemosphere 56, 1157-1167.
Koza, J. R., 1992. Genetic Programming I – On the
Programming of Computers be Means of Natural
Selection, Cambridge, MA, MIT Press.
Nguyen, M. H., Abbass, H. A., McKay, R. I., 2005.
Stopping Criteria for Ensemble of Evolutionary Artifi-
cial Neural Networks. Applied Soft Computing 6 (1),
100-107.
Ozdemir, H., Demir, G., Altay, G., Albayrak, S., Bayat,
C., 2008. Prediction of Tropospheric Ozone Concen-
tration by Employing Artificial Neural Networks.
Environmental Engineering Science 25(9), 1249-1254.
Özesmi, S. L., Tan, C. O., Özesmi, U., 2006.
Methodological issues in building, training, and
testing artificial neural networks in ecological
applications. Ecological Modelling 195 (1-2), 83-93.
Pires, J.C.M., Martins, F.G., Sousa, S.I.V., Alvim-Ferraz,
M.C.M., Pereira, M.C., 2008b. Selection and
Validation of Parameters in Multiple Linear and
Principal Component Regressions. Environmental
Modelling & Software 23 (1), 50-55.
Pires, J.C.M., Sousa, S.I.V., Pereira, M.C., Alvim-Ferraz,
M.C.M., Martins, F.G. 2008a. Management of air
quality monitoring using principal component and
cluster analysis – Part II: CO, NO
2
and O
3
.
Atmospheric Environment 42(6), 1261-1274.
Sousa, S.I.V., Martins, F.G., Alvim-Ferraz, M.C.M.,
Pereira, M.C., 2007. Multiple linear regression and
artificial neural networks based on principal
components to predict ozone concentrations.
Environmental Modelling & Software 22(1), 97-103.
Zolghadri, A., Monsion, M., Henry, D., Marchionini, C.,
Petrique, O., 2004. Development of an operational
model-based warning system for tropospheric ozone
concentrations in Bordeaux, France. Environmental
Modelling & Software 19(4), 369-382.
IJCCI 2009 - International Joint Conference on Computational Intelligence
554