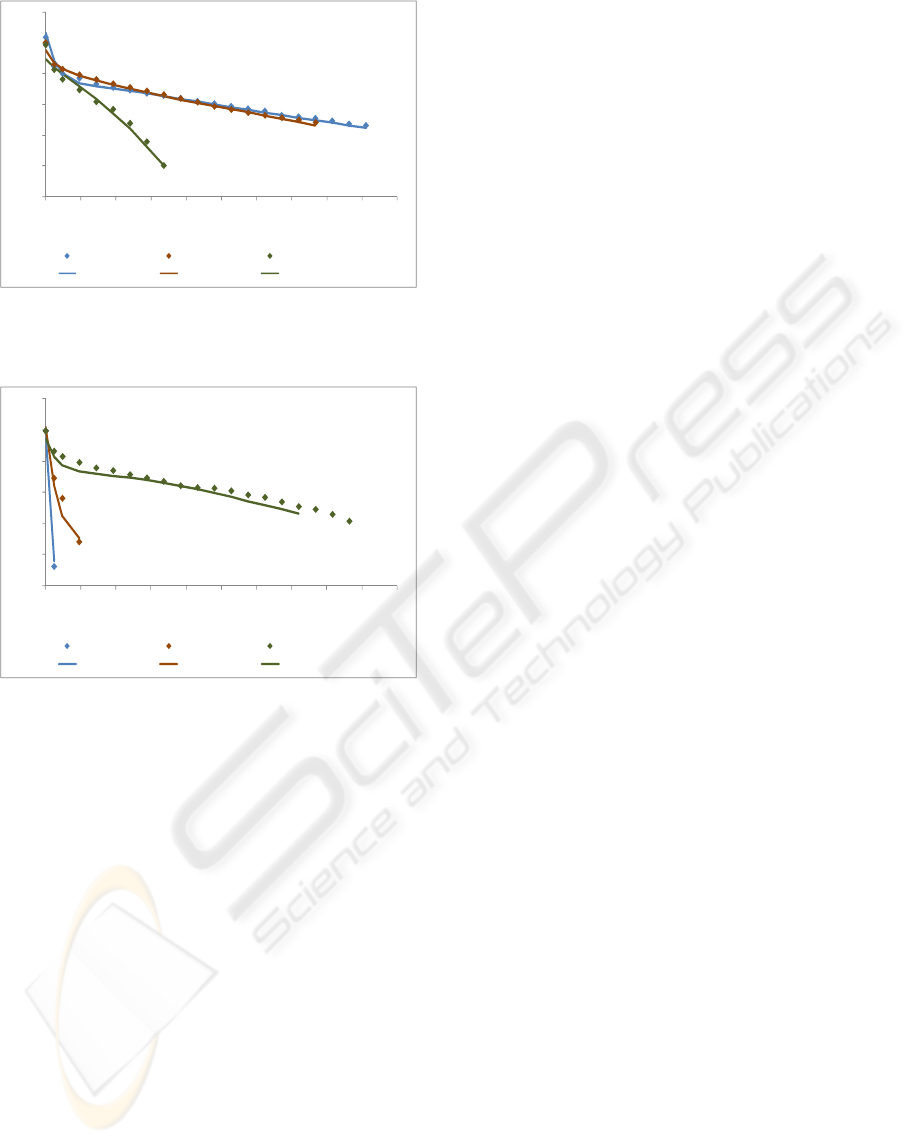
Figure 7: Experimental and modelling data for different
gases temperatures (fully humidified), for a cell
temperature of 298 K.
Figure 8: Experimental and modelling data for different
gases temperatures (fully humidified), for a cell
temperature of 333 K.
5 CONCLUSIONS
The effect of the relative humidity of the gases, and
of the temperature of the reactant gases and cell on
fuel cell performance was studied. It was concluded
that the fuel cell works better with both anode and
cathode humidified and that the temperature of the
gases and of the fuel cell should be the same. The
model developed in this work predicts very well the
experimental results. This kind of models could be
used with success for quick predictions of fuel cell
behaviour.
ACKNOWLEDGEMENTS
The partial support of “Fundação para a Ciência e
Tecnologia - Portugal” through project POCI/
EME/55497/2004 and scholarships SFRH/
BD/28166/2006 and SFRH/BD/23302/2005 is
gratefully acknowledged.
REFERENCES
Baschuk, J..J. and Li, X., 2000, Modeling of polymer
electrolyte membrane fuel cells with variable degrees
of water flooding, J. of Power Sources, Vol. 86, pp.
181-195.
Biyikoglu, A., 2005, Review of proton exchange fuel cell
models, International Journal of Hydrogen Energy,
Vol. 30, pp. 1185-1212.
Chiang, Y.M., Chang, L.C., Chang, F.J.,2004.
Comparison of static-feedforward and dynamic-
feedback neural networks for rainfall–runoff
modelling. Journal of Hydrology 290 (3-4), 297-311.
Gupta, R.R., Achenie, L.E.K., 2007. A network model for
gene regulation. Computers & Chemical Engineering
31 (8), 950-961.
Nagy, Z.K., 2007. Model based control of a yeast
fermentation bioreactor using optimally designed
artificial neural networks. Chemical Engineering
Journal 127 (1-3), 95-109.
Nguyen, M.H., Abbass, H.A., McKay, R.I., 2005.
Stopping criteria for ensemble of evolutionary
artificial neural networks. Applied Soft Computing 6
(1), 100-107.
Ogaji, S.O.T., Singh, R., Pilidis, P., Diacakis, M., 2006.
Modelling fuel cell performance using artificial
intelligence. Journal of Power Sources 154 (1), 192-
197.
Ou, S., Achenie, L.E.K., 2005. A hybrid neural network
model for PEM fuel cells. Journal of Power Sources
140 (2), 319-330.
Özesmi, S.L., Tan, C.O., Özesmi, U., 2006.
Methodological issues in building, training, and
testing artificial neural networks in ecological
applications. Ecological Modelling 195 (1-2), 83-93.
Saengrung, A., Abtahi, A., Zilouchian, A., 2007. Neural
network model for a commercial PEM fuel cell system.
Journal of Power Sources 172(2), 749-759.
Sousa, S.I.V., Martins, F.G., Alvim-Ferraz, M.C.M.,
Pereira, M.C., 2007. Multiple linear regression and
artificial neural networks based on principal
components to predict ozone concentrations.
Environmental Modelling & Software 22 (1), 97-103.
Uncini, A., 2003. Audio signal processing by neural
networks. Neurocomputing 55 (3-4), 593-625.
0.0
0.2
0.4
0.6
0.8
1.0
1.2
0.00 0.05 0.10 0.15 0.20 0.25 0.30 0.35 0.40 0.45 0.50
Vo lt age [V ]
Current Density [A/cm
2
]
Tgases=298 K Exp Tgases=313 K Exp Tgases=333 K Exp
Tgases=298 K Model Tgases=313 K Model Tgases=333 K Model
0.0
0.2
0.4
0.6
0.8
1.0
1.2
0.00 0.05 0.10 0.15 0.20 0.25 0.30 0.35 0.40 0.45 0.50
Vo lt age [V ]
Current Density [A/cm
2
]
Tgases=298 K Exp Tgases=313 K Exp Tgases=333 K Exp
Tgases=298 K Model Tgases=313 K Model Tgases=333 K Model
ARTIFICIAL NEURAL NETWORK MODEL APPLIED TO A PEM FUEL CELL
439