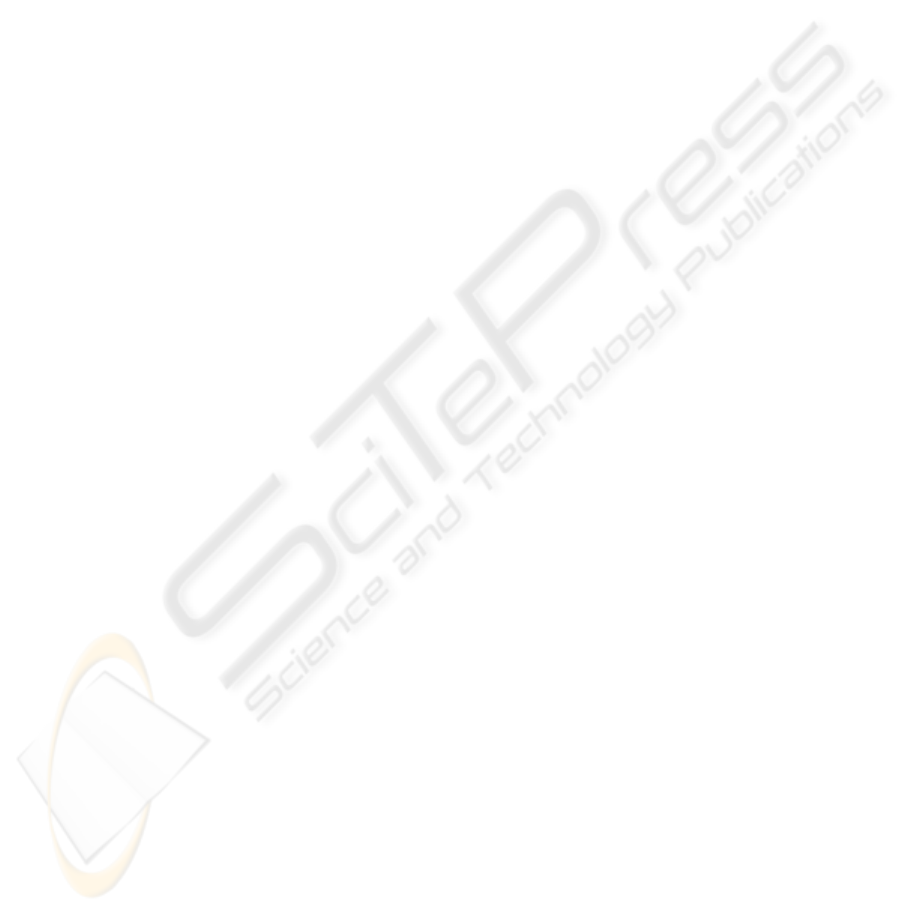
5 DISCUSSION
We have presented a neural network model, trained
on a diverse sample of images of both males and
females (with their respective human ratings), to
predict facial attractiveness means with a high
degree of correlation and agreement with human
raters. This study helps to reinforce the claim that
attractiveness assessments are data-driven, and
further expands on existing research using
computational modeling to make facial
attractiveness judgments. Given a larger dataset, it
may be possible to create a neural network that is
capable of producing human-like evaluations with
stronger correlations and agreement with human
raters.
In addition to learning facial attractiveness, we
have trained an ANN to distinguish between both
males and females, and found some evidence that
would suggest confidence plays a role in sex
classification. If it turns out that these confidence
ratings correspond to prototypicality
or averageness
– the more confident the network is that an image is
male (or female) the more prototypically male (or
female) it is – then we would have an especially
interesting result. This speaks to a larger and more
difficult question we have insufficient room to
explore at this point: why is prototypicality a
contributing factor to facial attractiveness? If an
ANN, in solving for sex classification, yields
prototypical male and female outputs in a way that at
least roughly corresponds to attractiveness ratings,
then one starts to wonder about the following
hypothesis: the contribution of prototypicality to
facial attractiveness could be a neurocomputational
consequence of mastering the task of male-female
facial classification. In other words, the
contribution of prototypicality to attractiveness may
“fall out of” the solution to male-female
classification (of course, as literature surveyed in the
introduction suggests, prototypicality is only one of
several contributors to attractiveness). That said,
any link between confidence ratings and
prototypicality needs to be independently
motivated. Moreover, much more work is required
in neuropsychology and computational modeling to
examine the preceding hypothesis, but it is at least
worth mentioning at this point.
All training of the sex classification network
made use of the sigmoid activation function. We
have not yet trained sex classification networks
using the radial basis function. This is an important
consideration for future work since the radial basis
function may make it easier than the sigmoid
function to motivate a link between confidence
ratings and prototypicality.
In conclusion, this work has produced useful
results. There were significant correlations with
human ratings of attractiveness despite the
ostensible difficulty of this computational task.
Corroborating other research, it would seem that
there are grounds to believe that human assessments
of facial attractiveness can be learned by a machine.
ACKNOWLEDGEMENTS
The authors would like to thank Kaitlin Tremblay
and Emrah Eren for helping to compile and compute
some of the data. An additional thanks is extended to
the participant in the above picture for allowing the
use of his image as well as to Kate Hargreaves for
editing.
REFERENCES
Bronstad, P. M., Langlois, J. H., & Russell, R. (2008).
Computational models of facial attractiveness
judgments. Perception, 37(1), 126.
Cheng, Y. D., O'Toole, A. J., & Abdi, H. (2001).
Classifying adults' and children's faces by sex:
Computational investigations of subcategorical feature
encoding. Cognitive Science: A Multidisciplinary
Journal, 25(5), 819-838.
Cunningham, M. R., Roberts, A. R., Barbee, A. P., Druen,
P. B., & Wu, C. H. (1995). Their ideas of beauty are,
on the whole, the same as ours: Consistency and
variability in the cross-cultural perception of female
physical attractiveness. Journal of Personality and
Social Psychology, 68(2), 261-279.
Dailey, M. N., Cottrell, G. W., Padgett, C., & Adolphs, R.
(2002). EMPATH: A neural network that categorizes
facial expressions. Journal of Cognitive Neuroscience,
14(8), 1158-1173.
DeSantis, A., & Kayson, W. A. (1997). Defendants'
characteristics of attractiveness, race, and sex and
sentencing decisions. Psychological Reports, 81(2),
679-683.
Eisenthal, Y., Dror, G., & Ruppin, E. (2006). Facial
attractiveness: Beauty and the machine. Neural
Computation, 18(1), 119-142.
Feingold, A. (1992). Gender differences in mate selection
preferences: A test of the parental investment model.
Psychological Bulletin, 112(1), 125-139.
Fiske, S. T. (2001). Effects of power on bias: Power
explains and maintains individual, group, and societal
disparities. The use and abuse of power: Multiple
perspectives on the causes of corruption, 181-193.
Furl, N., Phillips, P. J., & O'Toole, A. J. (2002). Face
recognition algorithms and the other-race effect:
IJCCI 2009 - International Joint Conference on Computational Intelligence
478