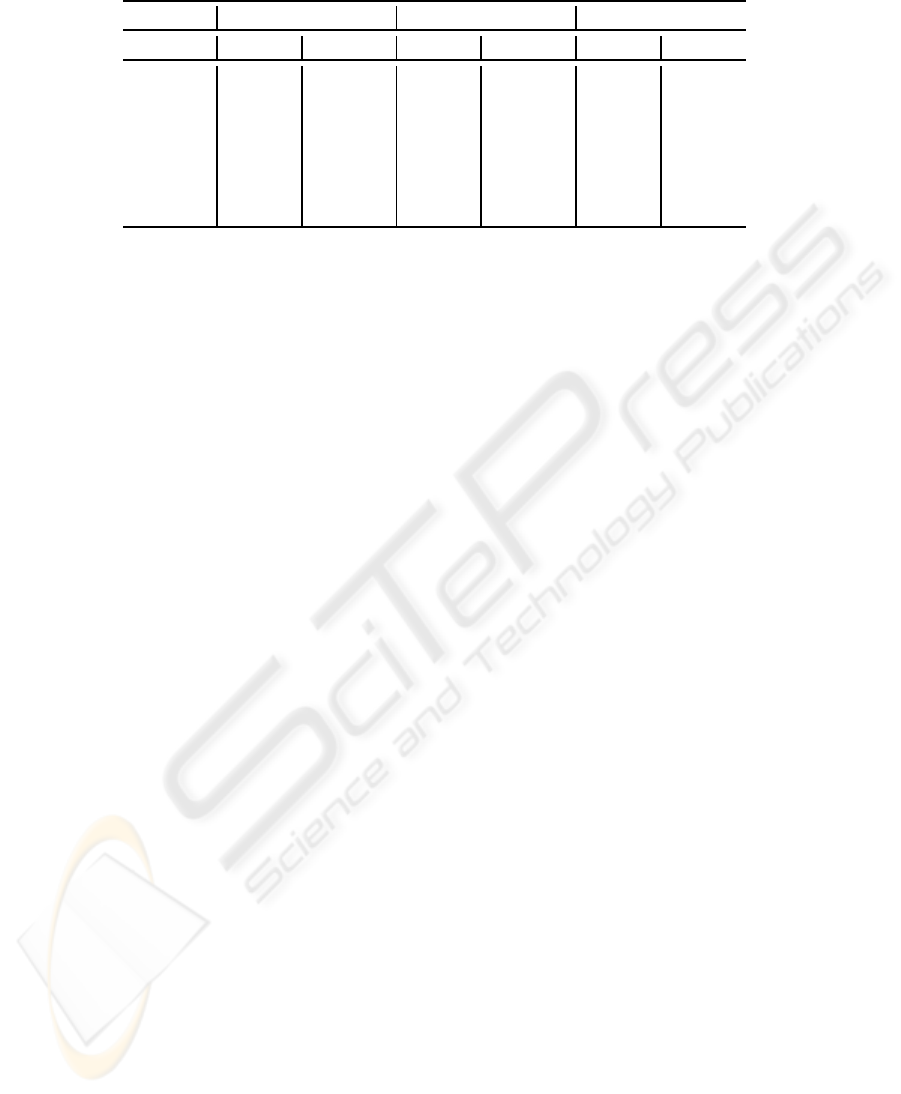
Table 4: Mean and standard deviation of sensitivity (in %) and specificity (in %) metrics on UCI data sets.
StdMLP SVM BalMLP
Data Set sens spec sens spec sens spec
Diabetes 61± 07 81± 06 70± 10 77± 04 73± 09 73 ± 04
Breast 42± 15 86± 08 60± 26 77± 08 66± 23 74 ± 11
Heart 47± 19 83± 05 61± 10 96± 09 73± 15 75± 09
Glass 84± 16 98± 02 87± 26 100± 00 90± 13 98± 03
Car 00± 00 100± 00 44 ± 21 98± 03 80± 15 77 ± 17
Yeast 06± 16 100± 00 29± 27 99± 04 82± 14 85± 03
Abalone 00 ± 00 100± 00 00± 00 100± 00 73± 12 79± 03
served that it depends on the number of class exam-
ples. We have also realized that this dependency can
be influenced by the asymptotic boundaries imposed
by the reduced size of the training and test data sets
and also by the difference in noise level between the
classes.
REFERENCES
Asuncion, A. and Newman, D. (2007). UCI machine learn-
ing repository.
Cortes, C. and Mohri, M. (2004). Auc optimization vs. error
rate minimization. In Advances in Neural Information
Processing Systems 16. MIT Press, Cambridge, MA.
Cortes, C. and Vapnik, V. (1995). Support-vector networks.
Mach. Learn., 20(3):273–297.
Egan, J. P. (1975). Signal Detection Theory and ROC Anal-
ysis. Academic Press.
Elkan, C. (2001). The foundations of cost-sensitive learn-
ing. In Proceedings of the Seventeenth International
Joint Conference on Artificial Intelligence, IJCAI,
pages 973–978.
Everson, R. M. and Fieldsend, J. E. (2006). Multi-class roc
analysis from a multi-objective optimisation perspec-
tive. Pattern Recogn. Lett., 27(8):918–927.
Fawcett, T. (2004). Roc graphs: Notes and practical consid-
erations for researchers. Technical report.
Graening, L., Jin, Y., and Sendhoff, B. (2006). General-
ization improvement in multi-objective learning. In
International Joint Conference on Neural Networks,
pages 9893–9900. IEEE Press.
Haykin, S. (1994). Neural Networks: A Comprehensive
Foundation. Macmillan, New York.
Japkowicz, N. (2000). The class imbalance problem: Sig-
nificance and strategies. In Proceedings of the 2000
International Conference on Artificial Intelligence,
pages 111–117.
Kupinski, M. A. and Anastasio, M. A. (1999). Multiobjec-
tive genetic optimization of diagnostic classifiers with
implications for generating receiver operating charac-
terisitic curves. IEEE Trans. Med. Imag., 18:675–685.
Provost, F. and Fawcett, T. (2001). Robust classification for
imprecise environments. Mach. Learn., 42(3):203–
231.
Provost, F. J., Fawcett, T., and Kohavi, R. (1998). The case
against accuracy estimation for comparing induction
algorithms. In ICML ’98: Proceedings of the Fif-
teenth International Conference on Machine Learn-
ing, pages 445–453, San Francisco, CA, USA. Mor-
gan Kaufmann Publishers Inc.
Rumelhart, D. E. and McClelland, J. L. (1986). Parallel dis-
tributed processing: Explorations in the microstruc-
ture of cognition, volume 1: Foundations. MIT Press.
Sanchez, M. S., Ortiz, M. C., Sarabia, L. A., and Lleti, R.
(2005). On pareto-optimal fronts for deciding about
sensitivity and specificity in class-modelling prob-
lems. Analytica Chimica Acta, 544(1-2):236–245.
Sun, Y., Kamel, M. S., Wong, A. K. C., and Wang, Y.
(2007). Cost-sensitive boosting for classification of
imbalanced data. Pattern Recognition, 40(12):3358–
3378.
Van Gestel, T., Suykens, J. A. K., Baesens, B., Viaene, S.,
Vanthienen, J., Dedene, G., De Moor, B., and Vande-
walle, J. (2004). Benchmarking least squares support
vector machine classifiers. Mach. Learn., 54(1):5–32.
Weiss, G. M. (2004). Mining with rarity: a unifying frame-
work. SIGKDD Explor. Newsl., 6(1):7–19.
Wu, G. and Chang, E. Y. (2005). Kba: Kernel boundary
alignment considering imbalanced data distribution.
IEEE Trans. Knowl. Data Eng., 17(6):786–795.
Zhou, Z.-H. and Liu, X.-Y. (2006). Training cost-sensitive
neural networks with methods addressing the class im-
balance problem. IEEE Trans. Knowl. Data Eng.,
18(1):63–77.
ARTIFICIAL NEURAL NETWORKS LEARNING IN ROC SPACE
489