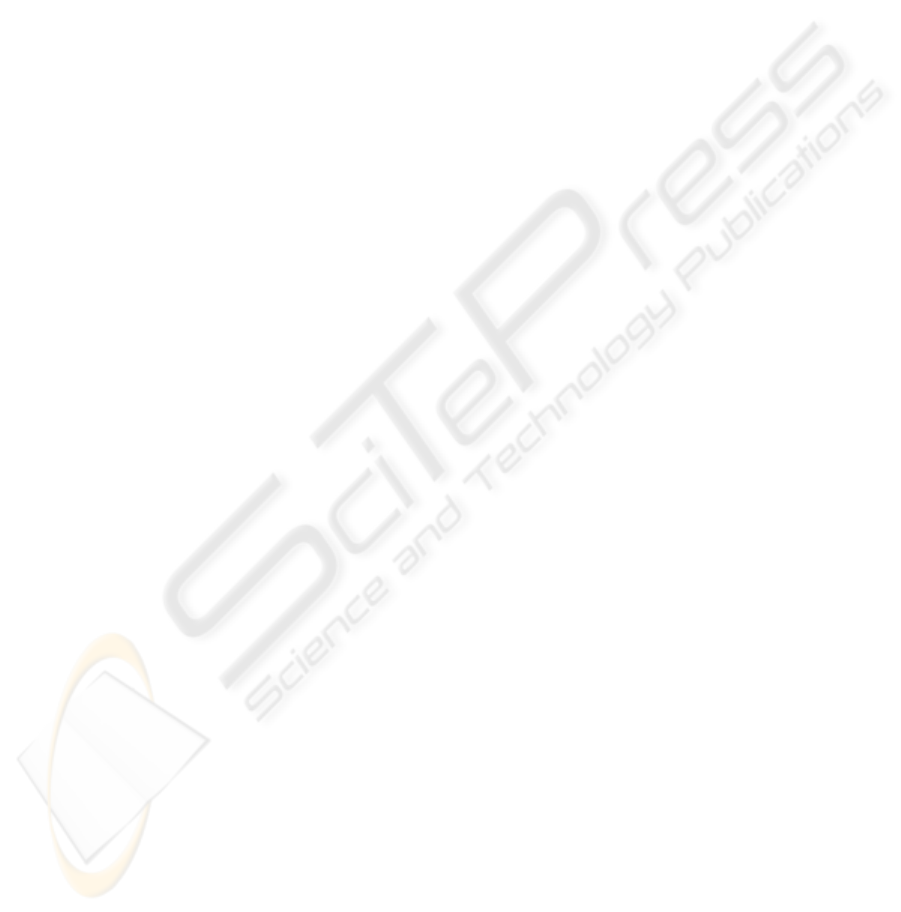
memory, and in the LTP process. It is capable to
produce hybrid neuromodulation, diffusive hybrid
neuromodulation (DHN) (Suárez Araujo, 2000). NO
has also opened a new dimension in our concept of
neural communication, overlaying the classical
synaptic neurotransmission, where information is
passed between neuronal elements at discrete loci
(synapses).
An intrinsic feature of the NO diffusion is the
formation of not-wired neighbourhoods, diffuse
neighbourhoods (DNB), which supports the
emerging of complex structures. The formation of
these structures has been a subject studied by other
authors (Krekelberg, 1996, 1997) and (Krekelberg
and Taylor, 1998). These studies have been specific
studies concerning with the cortical map formation
and their relationship with neighbourhoods in the
Kohonen SOM. They have not considered the DNB
as a possible underlying communication schema in
BNN and ANN. Our studies consider this capacity.
Our work introduces and studies fundamental
concepts in the formation of DNB, product of the
dynamics of NO diffusion. We understand DNB as
an auto-contained element in the behavior of NO
and the study of its dynamics as essential,
independent from the learning model or the neural
architecture where it is embodied in. This will
provide us a better understanding of the
computational skills that NO has; computational
skills based on the neural recruitment mechanisms,
emergence of complex structures and increase of
information capacity and processing. This justifies
the need of a generalist and in-depth development
like the one our work presents. We provide concepts
located in the Theoretical Framework of the study of
NO (Suárez Araujo, 2000). It will be able to manage
studies of causal aspects of the NO dynamics, and
comparative studies with concepts and classical
architectures.
In this paper, we focus our effort on the analysis
of DNB dynamics and its possible influence in
mechanisms and processes at the neural circuit
and/or higher level. An important aim is to infer
from the analysis a possible implication of VT in the
increase of the information representation capacity
in both BNN and ANN, in their architectures and in
the functional complexity of its main computation
element, the neuron.
We present a computational analysis of DNB,
based on our compartmental model of diffusion of
NO (Suárez Araujo, et al., 2006). This work requires
of a set of own concepts of the diffusive phenomena,
which we have defined and that are: Directionality
of NO dynamics (DNO), Average Influence (AI),
Diffusion Centre of the DNB (CDN) and DNB Limit
(DNBL).
The importance of this study is providing the
needed formalism to quantify the information
representation capacity that a type of NO diffusion-
based signalling can present.
2 METHOD
Diffusion is the main axis in the study of the NO
dynamics (Wood and Garthwaite, 1994), as well as
the main responsible of the NO influence to different
brain zones from a functional and structural point of
view. This influence is materialized, essentially, by
means of the Diffuse Neighbourhoods (DNB). This
concept allows to analyze how NO influences move
by means of diffusion as well as what is their
dependence with non-isotropy and non-
homogeneity. The establishment and analysis of
DNB, which we will perform using the
compartmental model of NO diffusion, precise to
formalize intrinsic aspects to the diffusion
phenomena and to the NO dynamics. On one hand,
we have the directionality measure of the NO
dynamics, which provokes different spatial-temporal
influences in the diffusion environment. This takes
us towards the concept of Average NO Influence,
key variable in the DNB definition. On the other
hand, its dynamic, adaptive and no local character
justifies the need of variables which formalize that
dynamism and its effect as diffusion centre of the
DNB and DNB limit.
2.1 Compartmental Model of NO
Diffusion and Concepts of Diffusion
The compartmental model of NO diffusion (Suárez
Araujo, et al., 2006) is a discrete computational
model that allows us to study the dynamic of NO,
generation, diffusion, self-regulation and
recombination, in biological and artificial
environments. Its main feature is its simplicity, it
can be considered as a general formal tool with
biological plausibility. It gathers real features of the
diffusion environment such as the no homogeneity
and the no-isotropy and possible morphology of the
NO synthesis.
It represents an important tool for designing and
interpreting biological experiments on NO behaviour
and its effect on brain structure and function.
The model is based in compartmental systems
(Anderson, 1983) and it is defined by a system of
A COMPUTATIONAL STUDY OF THE DIFFUSE NEIGHBOURHOODS IN BIOLOGICAL AND ARTIFICIAL
NEURAL NETWORKS
491