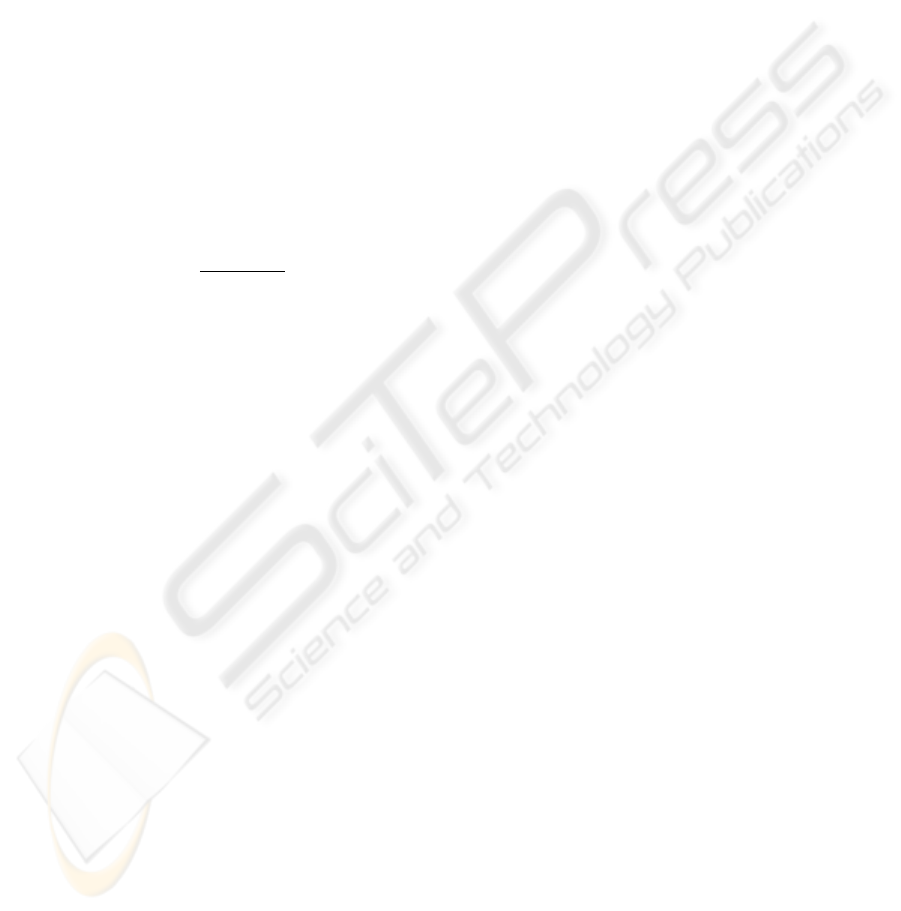
in a non-stream environment as well.
4 CONCLUSIONS
This position paper contains in extracts a bio-inspired
model, which follows the natural example of a molec-
ular world and which understands graph-related struc-
tures as molecular entities. The main objective is to
define a model that autonomously and adaptively be-
haves while performing internal tasks like the com-
munication with its environment, for example inside
communities. In this respect, fundamental compo-
nents like single/double bonds have been presented as
well as simple molecular shapes.
Currently, we are working on the stability of
atomic node, molecules, and proteins: a first approach
towards the stability of proteins is surely to count the
number of actor nodes at time points t and t − 1, re-
spectively, where we then get
∆(Π
i
,t) =
α
t
A
i
− α
t−1
A
i
α
t−1
A
i
(11)
The stability of a protein decreases, if ∆(Π
i
,t) ≤
0; it increases, if ∆(Π
i
,t) > 0. Even better, the corre-
sponding activity weights ω
A
i
→A
j
of the bonds and the
activation state of the atomic actor node σ
A
i
shall be
taken into account. However, the question concerning
the stability of molecular bonds and atomic actors is
herewith not answered and we for example check up
if a “valency” can be simulated as well and if other
criteria may be taken to fulfill a merge between ac-
tor nodes: when a catalyst starts its activity, does it
make a difference to start with some actor node or is
it of interesting to distinguish between “begin” and
“end” actor nodes? Furthermore, the semantic roles
inside a protein surely plays a promising aspect to-
wards the stability, since if all actor nodes are satisfied
and “convivial” in some way then the stability surely
is stronger than in another situation. Another inter-
esting point is the communication of a protein with
its environment, respective with other proteins: how
can information smoothly addressed to all proteins?
Here, we are currently thinking on taking into account
achievements from other bio-inspired systems like ar-
tificial immune systems.
ACKNOWLEDGEMENTS
The work is currently been done at the MINE re-
search group of the ILIAS Laboratory, Department of
Computer Science and Communication, University of
Luxembourg.
REFERENCES
Cai, D., Shao, Z., Hen, X., Yan, X., and Han, J.
(2005). Community mining from multi-relational net-
works. In Lecture Notes in Computer Science Vol.
3721/2005. Springer.
Girvan, M. and Newman, M. E. J. (2002). Community
structure in social and biological networks. In Na-
tional Academy of Science USA, 99:8271-8276.
Inokuchi, A., Washio, T., Nishimura, K., and Motoda, H.
(2002). A fast algorithm for mining frequent con-
nected subgraphs. In IBM Research, Tokyo Research
Laboratory. IBM Press.
Leach, A. (2001). Molecular Modelling - Principles and
Applications. Prentice Hall, 2nd edition. Mika, P.
(2007). Social Networks and the Semantic Web.
Springer, 1st edition.
Schommer, C. (2008). Sieving publishing communities in
dblp. In ICDIM Third International Conference on
Digital Information Management. IEEE Computer
Society.
Yan, X. and Han, J. (2002). gspan: Graph-based substruc-
ture pattern mining. In IEEE International Conference
on Data Mining (ICDM 2002). I EEE Computer So-
ciety.
KDIR 2009 - International Conference on Knowledge Discovery and Information Retrieval
222