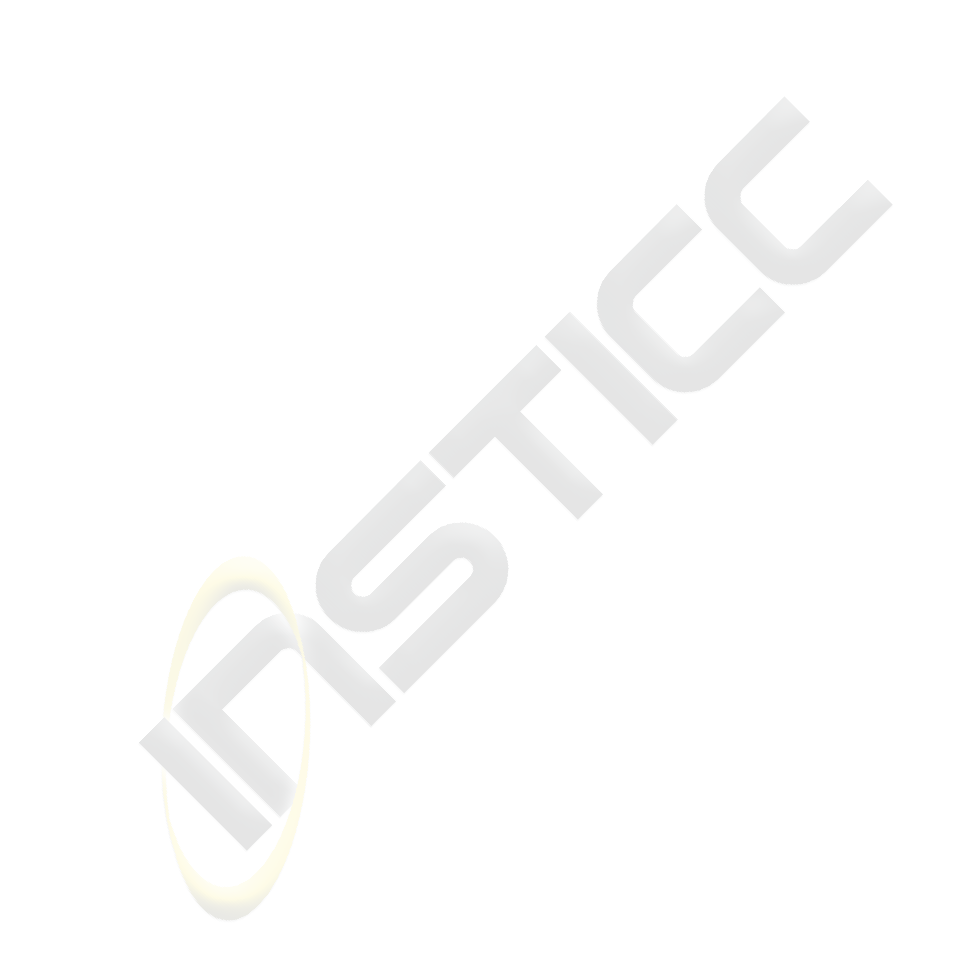
A Hybrid Multi-Experts Methodology for Mechanical
Defects’ Detection and Diagnosis
Kurosh Madani
1
, Moustapha Sene
2
and Véronique Amarger
1
1
Images, Signals, and Intelligent System Laboratory (LISSI/ EA 3956)
PARIS-EST/ PARIS 12 University, Senart Institute of Technology
Avenue Pierre Point, Lieusaint, 77127, France
2
Gaston Berger University, UFR SAT, Route de Ngallèle, BP 234 Saint-Louis, Sénégal
Abstract. Compared with parametric classifiers, several advantages set Neural
Networks as privileged approaches to be used as discriminating classifiers in
performing diagnosis tasks. In this paper, we present a hybrid Multi-Experts
neural based architecture for mechanical defects’ detection and diagnosis. This
solution is evaluated within vibratory analysis frame using a wavelet transform
faults’ detection scheme.
1 Introduction
Monitoring of mechanical systems requires development of adapted procedures com-
patible with the operation ranges (shapes) of the monitored devices. Generally, be-
haviours analysis is associated to a set of signals (called also signatures of the moni-
tored effects”). An example of such signatures could be obtained from chemical or
physical characteristics of materials composing the monitored mechanical devices or
involved in their operational phases, as: current, lubricant viscosity, acoustical signa-
tures, etc. For bearing defects, these signatures are characterized by transitory phe-
nomena (repetitive or random) due to the shocks’ effect on the structures. Such signa-
tures compile the frame of the vibratory analysis. A number of previous works show
that vibratory analysis issued signatures include pertinent information about mechani-
cal devices’ worsening [1], [2]. Note that conventional approaches of signal process-
ing don’t permit to exploit this information in its totality especially if the related sig-
natures are not periodical signals [3].
The general frame of the present work deals with early faults’ detection in indus-
trial plants, especially with mechanical faults’ detection in turning machines. For the
turning machines, the main faults which could be diagnosed through vibration analy-
sis are: imbalance, misalignment, looseness, shaft, bearing and gear damages, cavita-
tions in pumps, turbulent flows in ducts, foundation problems and electrical faults [4].
An additional difficulty related to the above-mentioned defects is due to the fact a
large part of mechanical devices in a turning machine are inaccessible, because they
are generally located inside the machine. Concerning inaccessible mechanical de-
vices, the vibratory analysis issued techniques show attractive features because they
may detect vibratory effects of internal devices from a global vibratory signature.
Proceedings of ICINCO 2009
6th International Conference on Informatics in Control, Automation and Robotics
Copyright © INSTICC