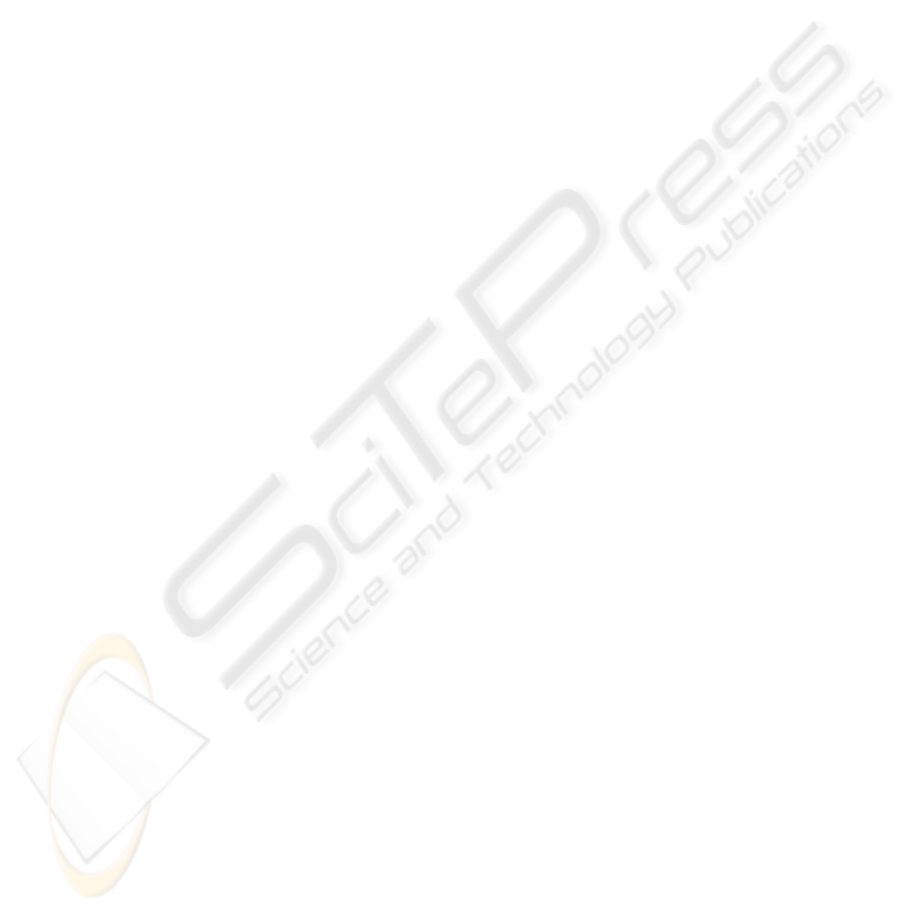
THE COLLABORATIVE LEARNING AGENT (CLA)
IN TRIDENT WARRIOR 08 EXERCISE
Charles Zhou, Ying Zhao and Chetan Kotak
Quantum Intelligence, Inc., 3375 Scott Blvd. Suite 100, Santa Clara, CA 95054, U.S.A.
Keywords: Agent learning, Collaboration, Anomaly search, Maritime domain awareness, Intelligence analysis,
Unstructured data, Text mining.
Abstract: The Collaborative Learning Agent (CLA) technology is designed to learn patterns from historical Maritime
Domain Awareness (MDA) data then use the patterns for identification and validation of anomalies and to
determine the reasons behind the anomalies. For example, when a ship is found to be speeding up or
slowing down using a traditional sensor-based movement information system such as Automatic
Information System (AIS) data, by adding the CLA, one might be able to link the ship or its current position
to the contextual patterns in the news, such as an unusual amount of commercial activities; typical weather,
terrain and environmental conditions in the region; or areas of interest associated with maritime incidents,
casualties, or military exercises. These patterns can help cross-validate warnings and reduce false alarms
that come from other sensor-based detections.
1 INTRODUCTION
Port security is important. The Navy needs to
enhance its awareness of potential threats in the
dynamic environment of Maritime Domain
Awareness (MDA) —and plan for potential high-
risk events such as use of maritime shipping for
malicious activities.
With ever-increasing operations with joint,
coalition, non-government, and volunteer
organizations require analysis of open-source
(uncertain, conflicting, partial, non-official) data.
Teams of analysts in MDA may consist of culturally
diverse partners, each with transient team members
using various organizational structures. These
characteristics place increasingly difficult demands
on short turn-around, high stakes, crisis driven,
intelligence analysis. To respond to these challenges,
more powerful information analysis tools can be of
great assistance to reduce their workload.
Structured data are typically stored in databases
such as Excel or XML files with well-defined labels
(meta-data). The unstructured data include free text,
word, .pdf, Powerpoint documents, and emails. A
large percentage of data remains unstructured
despite rapid development of database and data
management technologies. Organizations have an
opportunity to use unstructured data, if analysis tools
can be developed. In the MDA domain, both
structured data, e.g. Automatic Information System
(AIS) data of monitoring the tracks of vessels, and
unstructured data, e.g. intelligence reports from
various sources, are important. Anomalies in the
structured data such as vessels that are off tracks can
be detected using traditional anomaly detection
methods. However, it is challenging to analyze the
large amount unstructured data that are available.
There are a number of extant tools for text mining
including advanced search engine (Foltz, 2002;
Gerber, 2005), key word analysis and tagging
technology (Gerber, 2005), intelligence analysis
ontology for cognitive assistants (Tecuci et al., 2007,
2008); however, better tools are needed to achieve
advanced information discovery. Furthermore, it is
also challenging is to tie the anomalies detected
from structured data to the context of unstructured
data, which might shed light on social, economic
and political reasons for why anomalies occur.
Trident Warrior is an annual Navy FORCEnet Sea
Trial exercise to evaluate new technologies that
would benefit warfighers. The CLA technology was
selected for Trident Warrior 08 (TW08). This paper
reports the results from this exercise. In this paper,
we report how the CLA technology was applied and
evaluated in TW08.
323
Zhou C., Zhao Y. and Kotak C. (2009).
THE COLLABORATIVE LEARNING AGENT (CLA) IN TRIDENT WARRIOR 08 EXERCISE.
In Proceedings of the International Conference on Knowledge Discovery and Information Retrieval, pages 323-328
DOI: 10.5220/0002332903230328
Copyright
c
SciTePress