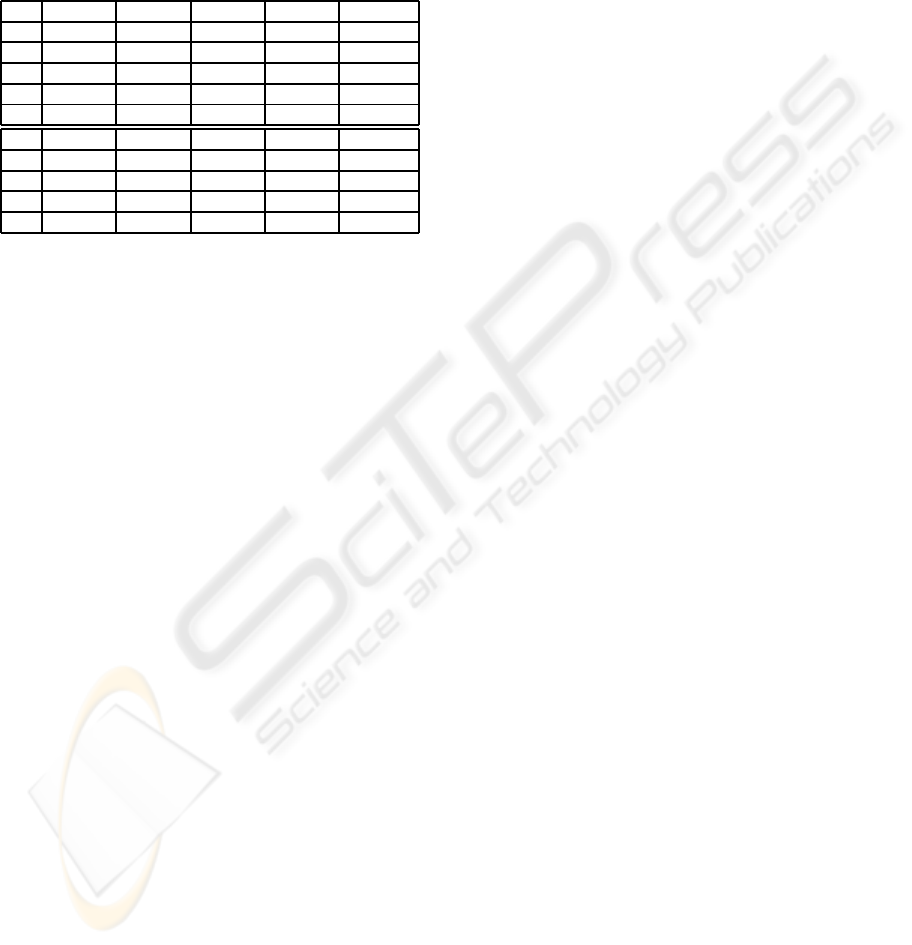
Table 1: Summary statistics of IPOP-CMA-ES (first block,
taken from (Auger and Hansen, 2005)) and PS-CMA-ES
(second block) on the multi-funnel functions f11 to f13,
f15, and f16 of the CEC 2005 test suite in n = 10 dimen-
sions. The minimum, median, maximum, and mean num-
ber of function evaluations needed to solve the problems
are reported over 25 repetitions of each test. The last col-
umn reports the success rates. See main text for strategy
parameters used.
min med max mean Suc. Rate
f11 3.05e+04 - - 6.31e+4 2.40e-01
f12 2.37e+03 3.10e+04 - 2.88e+04 8.80e-01
f13 - - - - 0
f15 - - - - 0
f16 - - - - 0
f11 3.56e+04 - - 5.02e+04 4.00e-01
f12 1.66e+04 1.89e+04 2.13e+04 1.92e+04 1.00e+00
f13 8.34e+04 - - 8.34e+04 4.00e-02
f15 3.30e+04 - - 3.65e+04 1.20e-01
f16 5.09e+04 - - 5.09e+04 4.00e-02
5 CONCLUSIONS
In summary, we have proposed: (a) a tunable real-
world multi-funnel test case for gradient-free evolu-
tionary optimizers and (b) that parallel island models
can relax the performance decrease of CMA-ES on
multi-funnel functions where the global optimum is
not located in the broadest funnel. The latter can be
explained by the irresolvable trade-off for the optimal
population size of CMA-ES on multi-funnelfunctions
(Lunacek et al., 2008). The larger the population size,
the more likely CMA-ES converges in the broadest,
sub-optimal funnel. This is supported by the fact that
IPOP-CMA-ES performs remarkably well when the
PES of LJ
38
is compressed to a single-funnel structure
and that the parallel island PS-CMA-ES outperforms
IPOP-CMA-ES on the considered sub-set of multi-
funnel functions from the CEC 2005 test suite. To
date, no population-based, gradient-free evolutionary
algorithm can solve the presented LJ
38
test case with-
out compression. Since many real-world applications
entail multi-funnel landscapes, we argue that the EC
community should focus on algorithms that can solve
such problems. As a benchmark, we suggest the LJ
38
problem, which should be considered in all future EC
studies that introduce a novel general-purpose opti-
mizer.
ACKNOWLEDGEMENTS
We thank Georg Ofenbeck for setting up the LJ cluster
simulations.
REFERENCES
Alba, E. (2005). Parallel Metaheuristics: A New Class of
Algorithms. Wiley-Interscience.
Auger, A. and Hansen, N. (2005). A restart CMA evolu-
tion strategy with increasing population size. In Proc.
IEEE Congress on Evolutionary Computation (CEC
2005), volume 2, pages 1769–1776.
Clark, P. L. (2004). Protein folding in the cell: reshaping
the folding funnel. Trends Biochem. Sci., 29(10):527–
534.
Doye, J. (2000). Effect of compression on the global opti-
mization of atomic clusters. Phys. Rev. E, 62(6, Part
B):8753–8761.
Doye, J. P. K., Miller, M. A., and Wales, D. J. (1999).
The double-funnel energy landscape of the 38-atom
Lennard-Jones cluster. J. Chem. Phys., 110(14):6896–
6906.
Hansen, N. (2007). The CMA Evolution Strategy: A Tuto-
rial. http://www.lri.fr/ hansen/cmatutorial.pdf.
Hansen, N. and Kern, S. (2004). Evaluating the CMA Evo-
lution Strategy on Multimodal Test Functions. In Lect.
Notes Comput. Sc., volume 3242 of Parallel Problem
Solving from Nature - PPSN VIII, pages 282–291.
Hansen, N. and Ostermeier, A. (2001). Completely Deran-
domized Self-Adaption in Evolution Strategies. Evo-
lutionary Computation, 9(2):159–195.
Lunacek, M., Whitley, D., and Sutton, A. (2008). The Im-
pact of Global Structure on Search. In Lect. Notes
Comput. Sc., volume 5199 of Parallel Problem Solv-
ing from Nature - PPSN X, pages 498–507.
M¨uller, C. L., Baumgartner, B., and Sbalzarini, I. F. (2009).
Particle swarm CMA evolution strategy for the opti-
mization of multi-funnel landscapes. In Proc. IEEE
Congress on Evolutionary Computation (CEC 2009),
pages 2685–2692.
Steinhardt, P. J., Nelson, D. R., and Ronchetti, M. (1983).
Bond-orientational order in liquids and glasses. Phys.
Rev. B, 28(2):784–805.
Suganthan, P. N., Hansen, N., Liang, J. J., Deb, K., Chen,
Y.-P., Auger, A., and Tiwari, S. (2005). Problem Defi-
nitions and Evaluation Criteria for the CEC 2005 Spe-
cial Session on Real-Parameter Optimization. Techni-
cal report, Nanyang Technological University, Singa-
pore.
Sutton, A. M., Whitley, D., Lunacek, M., and Howe, A.
(2006). PSO and multi-funnel landscapes: how co-
operation might limit exploration. In Proc. ACM
Genetic and Evolutionary Computation Conference
(GECCO’06), pages 75–82. ACM.
Wales, D. (2004). Energy Landscapes : Applications
to Clusters, Biomolecules and Glasses (Cambridge
Molecular Science). Cambridge University Press.
Wales, D. J. and Doye, J. P. K. (1997). Global Optimiza-
tion by Basin-Hopping and the Lowest Energy Struc-
tures of Lennard-Jones Clusters Containing up to 110
Atoms. J. Phys. Chem. A, 101(28):5111–5116.
A TUNABLE REAL-WORLD MULTI-FUNNEL BENCHMARK PROBLEM FOR EVOLUTIONARY OPTIMIZATION
- And Why Parallel Island Models Might Remedy the Failure of CMA-ES on It
253