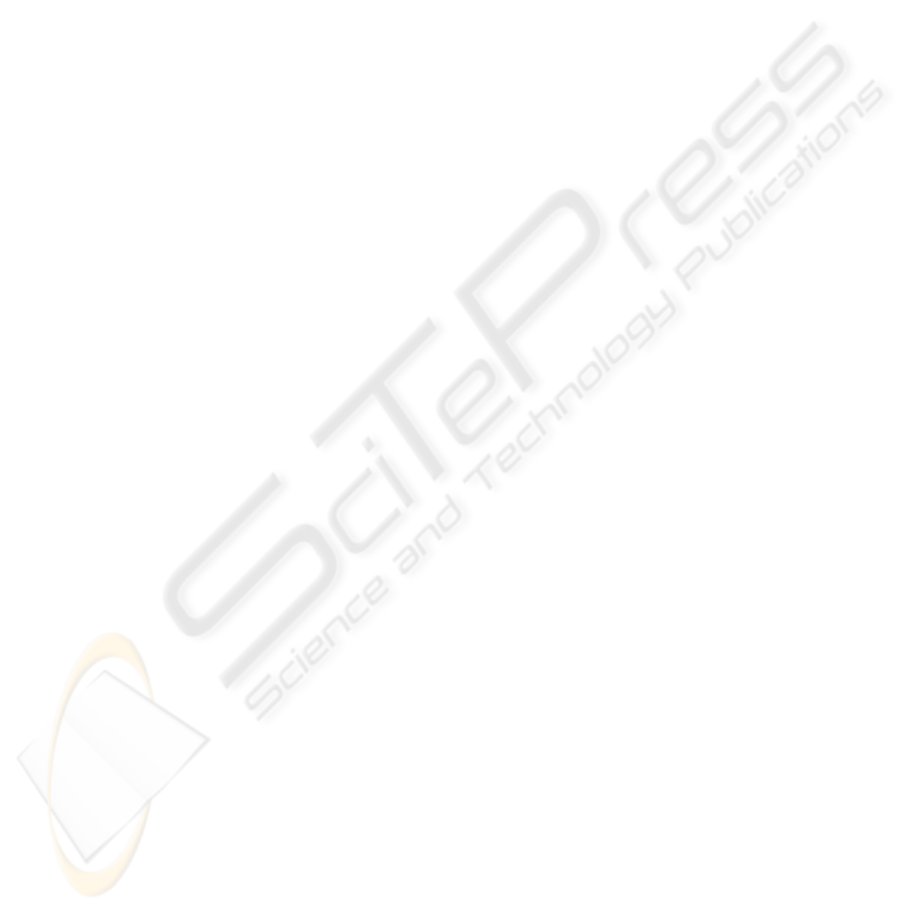
also added to the steering mechanism (Section 4.2).
To allow adjusting for incomplete turns, we also refine
the terminal set with 15 degree turns instead of 90
degree turns. Eventually in Section 4.3 the evolved
controller is transfered to the real Eyebot.
4.1 Evolution in Simulation
Table 1 lists the results of the experiments in simula-
tion. The best controller of every experiment is able
to navigate through all corridor systems, except in ex-
periments 4 and 6, where the controller solves 14 out
of 15.
Next to the more classic approach of changing the
number of generations and population size, we will
focus mainly on combining different fitness cases.
More precisely, we investigate if and how we can im-
prove the resulting controllers and reduce the com-
putation time by constructing well-considered cate-
gories of training environments.
Columns 7 and 8 display the results from the evo-
lutionary process. From all three runs, the best per-
forming controllers’ fitness on the last category is
listed. Remark that the differences in absolute num-
bers are small however significant, since a difference
of 0.001 can still be noticed by observation of the con-
trollers behaviour. Furthermore, the best controllers’
fitness of each run is averaged in the column mean.
Columns 9–12 contains results of verification tests.
Conclusions concerning the robustness and general-
ity of the best controller require verification in new
environments which were not used during evolution.
We considered five environments with the same diffi-
culty level of the environments in the last category of
fitness cases. The number of collisions (too close to
the wall) and the number of moves aggregated over all
five environments are listed. The number of environ-
ments (out of five) in which the robot reaches the end
in less than 500 moves is indicated as well. Finally
the fitness averaged over all 5 verification environ-
ments is found in the last column. Note that this was
calculated in order to make them comparable over all
penalty values.
For the noiseless simulations, experiment 1 us-
ing the mixed approach clearly yields the best results.
Even with significant time reduction compared to ex-
periments 2 and 3, this setup results in a more robust
(all verification environments are successfully com-
pleted) and efficient (small number of movements)
controller. Therefore it is very beneficial using mixed
categories containing enough diversity and increas-
ingly difficult fitness cases. This diversity enables the
evolutionary process to build further on more general
controllers.
4.2 Preparing for Reality
As stated in literature, noise can improve simulation
results and lead to more robust controllers (Jakobi
et al., 1995; Br
¨
aunl, 2006; Reynolds, 1994). Main
argument is that with noise, evolution is no longer
able to exploit coincidences in fitness cases only valid
in simulation and therefore leads to more robust con-
trollers. Indeed, in the second series of experiments,
with noise added to the sensor values, results im-
proved significantly.
Experiment 5 in Table 1 leads to a reasonable con-
troller without collisions, though two verification en-
vironments were not solved successfully. However,
since entropy is still fairly high, further improvements
can be expected. To verify this, in experiment 6 the
best run from experiment 5 was allowed to evolve for
some more generations resulting in a successful con-
troller which navigates very robustly and efficiently
in all verification environments. Important remark is
that the mean fitness value from experiment 5 is ex-
tremely high, even better than the best results in all
other experiments. Therefore the gradual approach is
very interesting for this problem domain since most
runs will lead to excellent controllers.
This intermediary setup thus provides sufficient
knowledge to move on for a more realistic simula-
tion. Next to the noisy sensor values, noise is added
to the steering mechanism as well. Furthermore, turns
of 15 degrees are used instead of 90. This way, the
controller will be able to navigate smoother and ad-
just for incomplete turns, which are very common in
reality. This clearly is a scaled up version of the pre-
vious problem. Though, by using exactly the same
setup of experiment 5 and augmenting the population
size and the number of generations, a very efficient
and robust solution was evolved. This solution is able
to fulfil, with 4 collisions in total, all tested environ-
ments, whether they were used during evolution or
not. An example trail of this controller is depicted in
Figure 2(a).
An interesting remark is that the underlying strat-
egy of nearly all successful controllers of the experi-
ments is wall following. Mostly, the left wall is fol-
lowed, navigating to the end of the corridor system
without turning and proceeding in the wrong direc-
tion. This general strategy is a nice example of evolu-
tionary processes to come up with simple yet general
and efficient solutions.
4.3 Transfer to Real World
When transferring the best controller thus far to real-
ity, performance slightly decreased. This was mainly
EVOLVING ROBUST ROBOT CONTROLLERS FOR CORRIDOR FOLLOWING USING GENETIC
PROGRAMMING
445